Let us look at the key differences between the Weibull distribution and the lognormal distribution:
Table Of Contents
Weibull Distribution vs Lognormal Distribution
Parameters | Weibull Distribution | Lognormal Distribution |
---|---|---|
1. Distribution shape | Can have various shapes: increasing, decreasing, or constant hazard rate
| Positively skewed, right-skewed distribution |
2. Transformation | It can be transformed into a straight line in a Weibull probability paper | It cannot be transformed into a straight line in a probability paper |
3. Symmetry | Asymmetrical | Positively skewed, right-skewed |
4. Applications | Reliability analysis, failure modeling, lifetime analysis | Financial modeling, stock prices, income distribution |
5. Tail Behavior | Can have different tail behaviors, such as heavy or light tails | Can have long right tails |
6. Data Modeling | Suitable for modeling data with different failure rates over time | Suitable for modeling data with multiplicative growth processes |
What Is Weibull Distribution?
The Weibull distribution is a continuous probability distribution commonly used to model the lifetime or reliability of a product. It is widely applied in various fields such as engineering, reliability analysis, finance, and healthcare. The purpose of the Weibull distribution is to describe the distribution of failure or survival times of a given population.
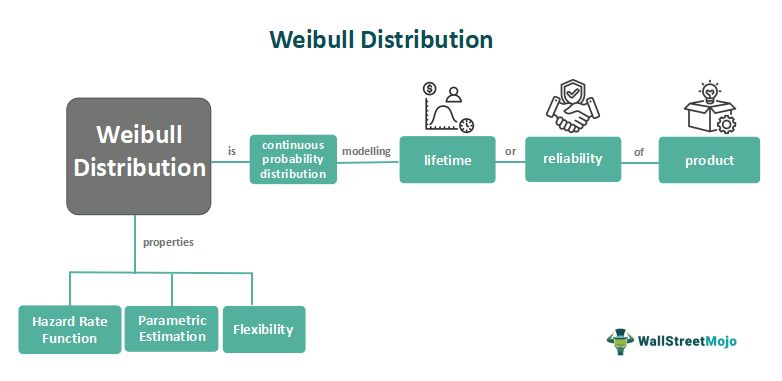
The importance of the Weibull distribution lies in its flexibility and ability to capture a wide range of failure patterns. Adjusting the shape and scale parameters can model various failure behaviors, such as early failures, constant failure rates, or wear-out failures. This makes it a valuable tool for reliability engineers and analysts to assess and predict the reliability and lifetime of products and systems.
Key Takeaways
- The Weibull distribution is a flexible probability distribution commonly useful in reliability analysis to model product, system, or material lifetime or failure times.
- It can capture various failure behaviors, including increasing, decreasing, or constant hazard rates, making it suitable for analyzing diverse reliability patterns.
- The distribution's shape and scale parameters allow customization to fit observed data and predict future reliability.
- Its applications extend to fields such as engineering, finance, healthcare, and risk assessment, where it aids in assessing reliability, planning maintenance, and making informed decisions.
Weibull Distribution Explained
The Weibull distribution is a probability distribution used to model the lifetime or reliability of products, systems, or materials. It was introduced by Wallodi Weibull in 1951. It is characterized by two parameters: the shape parameter, which determines the hazard rate's shape (increasing, decreasing, or constant), and the scale parameter, which determines the location on the time axis. The flexible distribution can capture various failure patterns, making it valuable for reliability analysis. It helps assess and predict the reliability and lifetime of various objects, making it widely used in engineering, finance, healthcare, and other fields.
It is particularly useful for analyzing data where the hazard rate (the probability of failure per unit time) changes over time. The distribution is characterized by the shape parameter (k) and the scale parameter (λ). The shape parameter determines the slope of the hazard rate function, which can be increasing, decreasing, or constant. The scale parameter determines the location of the distribution on the time axis.
Properties
Let us have a look at its key properties:
- Flexibility: The Weibull distribution is highly flexible and can model various failure behaviors. Adjusting the shape and scale parameters can capture various hazard rate shapes, including increasing, decreasing, and constant failure rates.
- Reliability Analysis: The distribution is widely useful in reliability analysis to assess the reliability and lifetime of products, systems, or materials. It provides insights into the failure behavior and helps estimate the probability of survival or failure at different time points.
- Survival and Failure Functions: The Weibull distribution provides convenient survival and failure functions. The survival function gives the probability of an object surviving beyond a specific time, while the failure function gives the probability of failure within a given time frame.
- Hazard Rate Function: The hazard rate function of the Weibull distribution describes the probability of failure per unit time. It can exhibit various shapes, such as increasing, decreasing, or constant, allowing for accurate modeling of different failure patterns.
- Parametric Estimation: The Weibull distribution allows parameter estimation based on observed data. This enables analysts to fit the distribution to empirical data and predict future reliability.
Formula
The formula gives the probability density function (PDF) of the Weibull distribution:
f(x; k, λ) = (k/λ) * (x/λ)^(k-1) * exp(- (x/λ)^k)
Where:
- x is the random variable (typically representing time or a measure of reliability)
- k is the shape parameter, which determines the shape of the hazard rate curve
- λ is the scale parameter, which determines the location of the distribution on the time axis
The formula gives the cumulative distribution function (CDF) of the Weibull distribution:
F(x; k, λ) = 1 - exp(-(x/λ)^k)
This formula gives the probability that the random variable x is less than or equal to a given value.
The hazard rate function (h(x)) of the Weibull distribution is given by:
h(x; k, λ) = (k/λ) * (x/λ)^(k-1)
The hazard rate represents the instantaneous failure rate at time x.
These formulas allow for calculating probabilities, quantiles, and other statistical measures related to the Weibull distribution, facilitating its application in reliability analysis and lifetime modeling.
Applications
Let us have a look at some notable applications:
- Reliability Engineering: The Weibull distribution is extensively useful to model the lifetimes of products, systems, or components. It helps analyze failure patterns, estimate failure rates, and predict the reliability of equipment over time.
- Failure Analysis: By fitting the Weibull distribution to failure data, analysts can identify failure modes, determine the causes of failures, and assess the reliability of different components or materials.
- Quality Control: The Weibull distribution assists in quality control processes by characterizing the failure behavior of manufactured products. It enables manufacturers to set appropriate warranty periods, identify potential issues, and improve product design and manufacturing processes.
- Reliability Testing: The Weibull distribution is useful in accelerated life testing. Products are subjected to accelerated stress conditions to predict their lifetime under normal operating conditions. It aids in determining product durability and reliability under different environments and usage scenarios.
Weibull Distribution vs Normal Distribution
Let us look at the key differences between the Weibull distribution and the normal distribution:
Parameters | Weibull Distribution | Normal Distribution |
---|---|---|
1. Shape | Can have various shapes: increasing, decreasing, or constant hazard rate | The symmetrical, bell-shaped curve |
2. Symmetry | Asymmetrical | Symmetrical |
3. Applications | Reliability analysis, failure modeling, risk assessment | Statistical analysis, process control, finance |
4. Tail Behavior | Can have different tail behaviors, such as heavy or light tails
| Tails decrease rapidly (bell-shaped) |
5. Data Modeling | Suitable for modeling data with different failure rates over time | Suitable for modeling symmetric and unimodal data |