Table Of Contents
What Is Volatility Clustering?
Volatility clustering is a financial phenomenon characterized by significant fluctuations or changes in asset prices that form clusters or groups. This clustering occurs primarily due to the extreme volatility experienced by the market in response to global events.
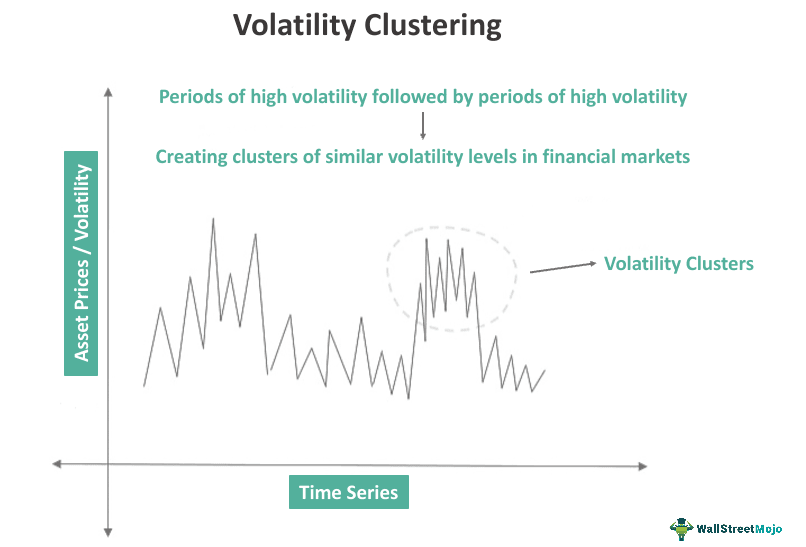
Detecting volatility clustering in financial markets involves considering various factors. These clusters persist over specific periods and are often followed by periods of low volatility. To identify it, several tests have been developed. However, it's important to note that the mixed reactions from investors can significantly influence these market swings and contribute to the observed clustering patterns.
Table of contents
- Volatility Clustering refers to the phenomenon of extreme fluctuations in asset prices leading to the formation of clusters or groups within the price chart.
- This concept was first observed by the American mathematician Benoit Mandelbrot in 1963, specifically in commodity prices within the exchange market.
- In addition to clustering, econometricians use models like ARCH (Autoregressive Conditional Heteroskedasticity) and GARCH (Generalized Autoregressive Conditional Heteroskedasticity) to analyze and predict volatility patterns.
- Among the different causes of it, behavioral switching and market regimes play significant roles.
Volatility Clustering Explained
Volatility Clustering in finance is crucial to understanding the trends and patterns from the clusters formed due to market fluctuations. According to econometricians, it is also known as autoregressive conditional heteroskedasticity (ARCH). Another extension is volatility clustering and GARCH (Generalized Autoregressive Conditional Heteroskedasticity). Here, volatility plays a crucial role in their formation. It causes huge changes in asset prices. With high volatility, there are clusters developed at certain points. They are later followed by low volatility, where there is minimal change. However, this clustering can bring overreaction and amplified swings.
American Mathematician Benoit Mandelbrot first proposed volatility clustering detection in 1963. The first observation occurred in the commodity prices. In the later stages, the influence was visible in the stock and other asset prices. Besides, a similar effect existed in the exchange rates and market indices. Other economists like Tim Bollerslev and Robert F. Engle also contributed. They prepared supporting volatility clustering and GARCH, and ARCH models. This model can also be used in developing portfolio models and risk estimation.
With investors' frequent and strong trading activities, prices are attracted to a stable circle. In such a case, the prices fluctuate with higher or lower volatility. This change usually depends on the current ongoing market conditions. Traders skeptical of the market may rush with their decision. As a result, they might sell or pick up certain trades.
In this process, the market flow gets disrupted, causing high volatility. And during that time, the noise traders get triggered to switch positions constantly. Thus, these market shocks lead to the formation of volatility clustering. In short, a cluster or group of inconsistent candles in a certain trend is visible. However, capturing volatility clustering requires different tests.
Causes
Every concept has certain factors to influence, and so does volatility clustering. Here are certain reasons that lead to the formation of clusters in the market. Let us look at them:
- Behavioral Switching: Among the major causes, the investor's behavior plays a vital role in the clustering. In this type, the market players switch from fundamental to chartist behavior. It means that they tend not to follow their analysis but instead follow the charts. As a result, this behavioral switching leads to larger fluctuations. Also, the model presented by Thomas Lux and Michele Marchesi in 2000 defines the same.
- Historical Volatility: The degree of price fluctuations in the past significantly influences today's volatility. By measuring the yield rate of security, it is possible to test for volatility clustering. Likewise, it is also viable for capturing clustering and trend patterns.
- Arrival Rates of Information: This heterogeneity in the news arrival can also lead to the origin of volatility clustering. A certain piece of news can deliver varied results for investors in different time zones. If it occurs, it can have different market reactions. As a result, time horizons can cause an impact on price dynamics, causing clusters.
- Evolutionary Models: The competition between various strategies and models tends to create clusters. The evolution in the trader's strategy and trading method is also connected to the clusters. Thus, its identification and detection are crucial at certain points.
- Time Spent in the Market Regime: Behavioral switching and the time spent in the market also influence the volatility. The traders with heavy, long-tailed durations are indirectly involved in forming clusters. This system is also known as "renewal switching."
Examples
Let us look at the different examples of volatility clustering to comprehend the concept in a better and easier way:
Example #1
Suppose Glossy and Stefin are traders operating in their respective stock exchanges. For the past month, the market has been operating steadily. There were minimum fluctuations with a consolidation phase in action. But now, the market receives negative sentiment about the nation's safety. In short, the country declared war against its rivals. As a result, out of panic, Glossy, Stefin, and other investors switched positions. It resulted in extreme volatility and price fluctuations. A week later, the market indices showed a cluster formation that day. Low-volatility candles later followed it.
Example #2
The volatility clustering of ESG (Environmental, social, and governance) indices is lower in the advanced markets. However, the same volatility in emerging markets is relatively higher. Of all, Brazil has the highest volatility of 95%. Whereas the United States noted 91%.
Importance
Volatility clustering plays a significant role in determining future market effects. Let us look at the importance of volatility clustering in various segments of the market:
- Volatility clustering can help in identifying future risks. Investors can estimate the risk level and update their portfolios.
- Users can produce a forecast of the market consequences. With the help of analytical tools, further data on market regimes and shift in sentiments can be extracted.
- It helps traders to improve their option pricing strategies. As this clustering affects future volatility, pre-deciding the prices of options becomes easy.
- Volatility can highly influence the portfolios of clients and users. As a result, this clustering can help to prevent market shocks.
Frequently Asked Questions (FAQs)
It has a significant impact on asset management. Asset managers need to understand and account for volatility clustering when making investment decisions. Groups of high volatility can indicate periods of increased market risk and uncertainty, influencing asset allocation, risk management strategies, and the selection of investment instruments. Properly considering clustering helps asset managers navigate changing market conditions and optimize portfolio performance.
Volatility clustering has several applications in finance and risk management. It is used in options pricing models to estimate future volatility and determine appropriate option premiums. Cluster analysis is also valuable in risk management, as it helps quantify the potential downside risk and develop risk mitigation strategies. Additionally, volatility clustering models can be utilized in developing trading strategies and asset allocation models and assessing the performance of investment portfolios.
Different tests determine volatility clustering by examining the statistical properties of asset price movements. Furthermore, different tests assess the persistence of volatility. High-frequency data analysis provides insights into short-term clustering patterns.
Recommended Articles
This article has been a guide to what is Volatility Clustering. Here, we explain it in detail, including its examples, importance, and causes. You may also find some useful articles here -