Table Of Contents
What Are Unit Root Tests?
A Unit Root Test is a statistical method employed in econometrics to determine whether a time series dataset is non-stationary and contains a unit root. A unit root indicates that a variable is affected by random shocks and tends to return to its mean over time, suggesting a lack of long-term trend or stability. These tests are used in econometrics to analyze economic data.
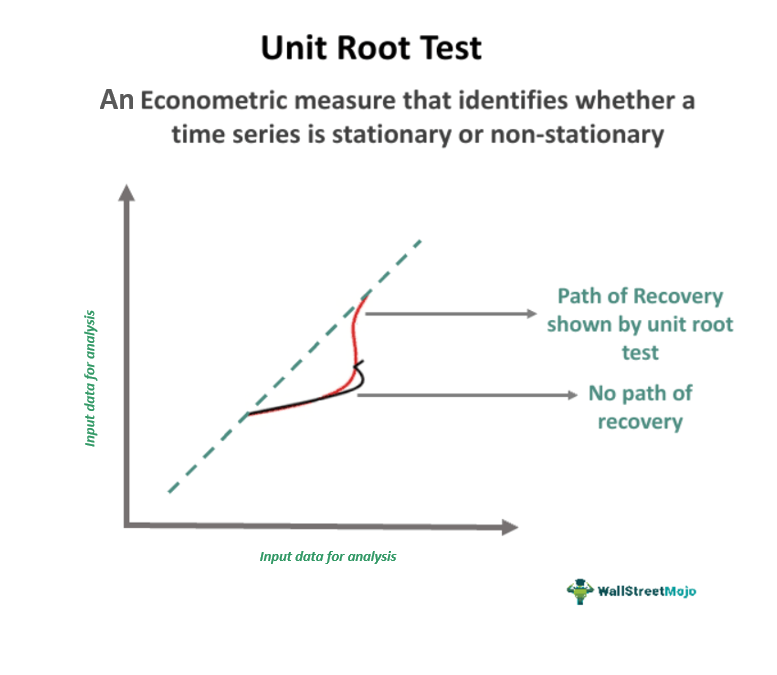
Stationary time series data is crucial for accurate modeling and forecasting in statistical analysis. Hence, researchers, economists, and analysts employ the unit root test to confirm if statistical properties like mean and variance have remained constant over time. If a series is non-stationary (contains a unit root), it can lead to misleading regression results and unreliable forecasts. It happens because the statistical properties of non-stationary data change over time.
Key Takeaways
- A unit root test refers to an econometric measure that aids researchers in identifying whether a time series is stationary or non-stationary.
- Some of the standard methods are the Augmented Dickey-Fuller (ADF), Dickey-Fuller (DF), and Phillips-Perron (PP) tests.
- Rejecting the null hypothesis of a unit root test indicates stationarity, making the data suitable for various time series models and analyses.
- Such tests are critical as they ensure the validity of statistical analysis, improve forecasting accuracy, and provide a solid foundation for economic research and policymaking.
Unit Root Tests Explained
Unit root tests are essential tools in time series analysis. They are used to determine whether a variable is non-stationary (has a unit root) or stationary. A non-stationary variable (as the name suggests) is one where the mean, variance, or autocorrelation changes over time. A stationary variable is one where statistical property remains unchanged over time.
There are many unit root tests in econometrics and time series analysis, as discussed below:
- Augmented Dickey-Fuller (ADF) Test: In the context of the ADF test, a unit root suggests that the series follows a random walk pattern, where changes from one period to the next are unorganized and unpredictable.
- Dickey-Fuller Test (DF) Test: This is the simpler version of the ADF test, which does not include additional lagged differences in the regression equation.
- Phillips-Perron (PP) Test: Similar to ADF, the PP test examines a unit root and rectifies errors like autocorrelation and heteroskedasticity in the given series. It is especially useful for analyzing non-normal data or data that contains outliers since it is based on nonparametric regression. Nonparametric regression does not make any assumptions about the underlying distribution of the data in question.
- Elliott-Rothenberg-Stock (ERS) Test: This test is specifically designed to handle structural breaks in time series data, allowing for a more robust analysis in the presence of such noise. Structural breaks refer to changes in underlying trends or patterns of a time series.
- Zivot-Andrews Test: It is used when there might be a single structural break in the data. It is an extension of the ADF test that allows for a structural break at an unknown point in the series. A structural break is a sudden and permanent change in the level or trend of a time series.
Formula
Based on the assumptions, the formula for the unit root test varies as follows:
Case 1 - Without Constant and Trend:
δYt-1+ut
Case 2 - With Constant:
α+δYt-1+ut
Case 3 - With Constant and Trend:
α+βT+δYt-1+ut
Examples
A unit root test makes the analytical process seamless and efficient. Let us understand its application in certain scenarios.
Example #1
Suppose Laura, the sales head of ABC Co., uses the unit root test to analyze time series datasets for one of her retail stores. In this case, she wishes to analyze the monthly sales data. She applies the Augmented Dickey-Fuller (ADF) test to the sales data. The purpose of this test is to determine whether the data exhibits a unit root, indicating a random walk and non-stationary behavior, or if it is stationary over time.
The unit root test null hypothesis of the ADF test asserts that unit roots exist in the time series sample. If the calculated test statistic is significantly lower than the critical values, the null hypothesis can be rejected. This rejection implies that the data is stationary, without a unit root, making it valuable for accurate forecasting and understanding the underlying patterns in the sales data.
Now, let us see what Laura achieves by doing this. She ensures that the sales data is stationary before making important decisions. This is because stationary data will likely be more accurate, improving the quality and relevance of business decisions.
Example #2
Suppose the stock price movements of a specific stock in the last ten years without a constant trend are:
Time | Stock Price | ∆ Stock Price ($) |
---|---|---|
1 | $78 | |
2 | $77 | -1 |
3 | $81 | 4 |
4 | $87 | 6 |
5 | $85 | -2 |
6 | $89 | 4 |
7 | $92 | 3 |
8 | $95 | 3 |
9 | $92 | -3 |
10 | $94 | 2 |
Since the series has no constant trend, one can use the formula δYt-1+ut to find the time series. Using this test, analysts identify patterns in price movements, determining whether such data is stationary or non-stationary. It enables them to make decisions and market predictions.
Applications
Listed below are some ways in which the unit root tests can be used:
- It is used for forecasting economic variables by establishing the order of integration.
- It helps analyze the long-term behavior of economic variables.
- Unit root tests enable the examination of stock prices, exchange rates, and other financial variables to assess future trends in these areas.
- These tests are applied to macroeconomic variables such as GDP, inflation, and unemployment rates to understand their long-term patterns and relationships.
- They are used with asset pricing models to determine the stationarity of the variables used in these models.
- Environmental studies use these tests to analyze time series data related to pollution, climate, and other environmental factors.
- Monitoring, controlling, and improving the quality of products in manufacturing over time is possible by studying the results obtained from unit root tests.
- A major application that helps on a macroeconomic level is the analysis of patterns in disease prevalence, patient outcomes, or healthcare costs.
However, like other statistical techniques, this measure is also prone to certain limitations. Primarily, its outcomes can be sensitive to the sample size, leading to different results even with minor variations in input variables. Also, it assumes that the underlying process is linear, which may not always be the case. It even fails to provide information about the order of integration or the presence of structural breaks in the data.
Further, its interpretation is quite challenging and requires strong econometrics knowledge. Moreover, the outcomes may not always be definitive, requiring extensive analysis and testing with other methods.
Still, unit root tests are used extensively in situations where time series analysis is needed. Hence, using it in conjunction with other testing methodologies may prove wise.
Importance
Unit root tests are essential tools in time series analysis and econometrics. They play a crucial role in determining whether a given time series dataset is stationary or non-stationary. Its importance can be outlined as follows:
- Critical for Time Series Modeling: It facilitates employing time series models such as Vector Auto-Regression (VAR) and Auto-Regressive Integrated Moving Average (ARIMA), which requires the data to be stationary, showing constant mean and variance over time.
- Avoids Spurious Regression: Since non-stationary time series can lead to false regression outcomes, where unrelated series appear correlated simply because they both have trends, stationary testing helps identify and address this issue.
- Enhances Forecasting Accuracy: The stationary series tend to exhibit more predictable patterns, making them easier to forecast accurately. Hence, the unit root tests assist analysts in selecting appropriate models for such analysis.
- Facilitates Policy Formulation and Economic Research: In the field of economics, such tests are indispensable for making policy decisions and economic research that rely on interpreting the underlying patterns in economic variables identified through this test.
- Prevents Misinterpretation: These tests provide a systematic approach to assess the stationarity of a time series, saving analysts from misinterpreting results or drawing false conclusions about data patterns. Marketing analysts, financial analysts, and researchers are some examples of professionals who use this testing method to their advantage.
- Cointegration Analysis: Such tests are fundamental in cointegration analysis that helps understand the various equilibrium relationships among economic variables. Cointegration analysis is useful for identifying long-run relationships between the given time series, which helps reduce or eliminate spurious regression.