Table Of Contents
What Is A Unit Root?
A unit root is a property of a stochastic process, making the statistical analysis of time series models complex. In other words, the presence of a unit root indicates a stochastic trend.
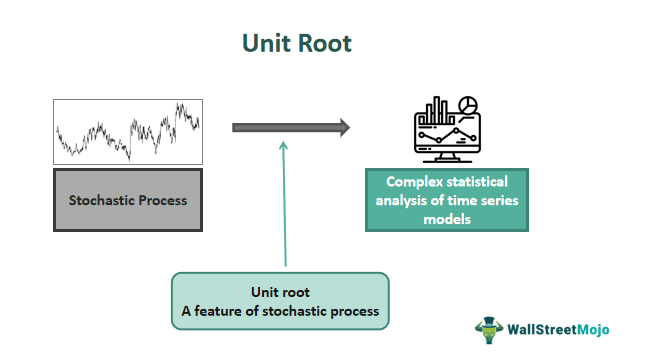
An econometric analysis is conducted to determine whether a time series data set is stationary. This depends on the presence of the unit root. In case a unit root appears in the time series model, it may appear prominent at first but is mostly meaningless and leads to spurious regressions. Therefore, testing for unit roots is important in time series analysis.
Key Takeaways
- The unit root is the presence of uncalled variations and fluctuations in a time series model, mostly in stochastic processes. Its presence explains whether the time series dataset is stationary or non-stationary.
- If a non-stationary element or process is present, the time series is non-stationary and hence is differentiated to achieve stationarity before picking up for any further analysis.
- Some major non-stationary element detection tests are Phillips-Perron, Augmented Dickey-Fuller, Zivot Andrews, and Elliott Rothenberg Stock tests.
- Not checking the time series for non-stationarity can bring negative outcomes, inaccuracy, errors, and impact decision-making.
Unit Root In Time Series Explained
Unit root refers to the appearance of fluctuations and variations in the time series dataset, defining it to be non-stationary. It is a critical aspect of time series analysis which eventually leads to deriving meaningful data insights. When a unit root is present in a time series, it indicates a lack of stationarity and can lead to fake regression. Before any time series is employed for forecasting and modeling, it is necessary to be verified by conducting a unit root testing because if a unit root is present, the data is not differentiated or cleared; it would simply bring errors, negative implications, and outcomes in the forecasting, which means the analyst will have wrong insights and, hence, will make incorrect decisions.
The unit root test finds the unit root and is also performed as part of the econometric analysis to derive a time series's structural break and integration order. This integration order defines the number of differences to make the series stationary. As its application in finance is concerned, unit root plays a key role in asset pricing models.
The unit root process, in a nutshell, states that its presence refers to the unit root in a time series model and where a single shock has a persistent effect, which means any random event can influence the process indefinitely. It is imperative to achieve stationarity in the time series before jumping on to any further analysis.
How To Detect?
There are different methods and tests to detect unit roots in time series; some of the main tests widely used by analysts are -
#1 - Augmented Dickey-Fuller Test (ADF)
It is the most common test. It is known to handle complex models but must be used cautiously. The test has a null hypothesis, which suggests the presence of a non-stationary element, and an alternative hypothesis, referring to a stationary trend.
#2 - Zivot-Andrews Test
This test detects a single structural data break. It is employed as an extension of the ADF test to have a structural break at any unknown point.
#3 - Kwiatkowski Phillips Schmidt Shin (KPSS) Test
This test is again important to detect non-stationary elements with a mean or linear trend. The test uses mean and variance across time. Again, this test follows hypothesis testing. The null hypothesis suggests the data is stationary, and the alternative hypothesis states that the data is not stationary. This test divides the series into parts, like a deterministic trend, a stationary error, and a random walk.
#4 - Elliott-Rothenberg-Stock (ERS) Test
This test is particularly useful in handling structural breaks in time series. Structural breaks refer to changes that occur in time series patterns and trends.
#5 - Phillips-Perron Test
Again, this test is quite similar to ADF; this test evaluates time series for non-stationary elements and sometimes corrects autocorrelation, heteroskedasticity, and other data errors. Every test has its specialty; the Phillips-Perron test is known for testing abnormal data.
Examples
Let us look into a few examples:
Example #1
Suppose Julia is an ace investor and is familiar with stock price analysis and market research. She is currently interested in investing in a steel industry stock. She is focusing on studying its historical stock prices to determine any pattern or price trend, including growth rate, daily return, and comparative analysis with other stocks.
Julia collects the stock price data from various sources and fits it into a time series model for analysis to predict the future stock price. However, at first, the unit root has a strong presence because the time series is trending up and down with varying fluctuations over time. It simply means that the stock price has a problem, and the model is non-stationary.
Julia cannot analyze the stock if there is a problem with its data. Therefore, Julia first decides to achieve stationarity and employs various methods to do so. One common technique is to differentiate the series, which means subtracting the current value from its previous value.
Once Julia achieves stationarity in its time series dataset, she can analyze the stock prices more accurately and has no concerns regarding the outcome, relevance, and efficiency. This is a simple example; in reality, many other factors are considered.
Example #2
Suppose a multinational company that manufactures multiple products across different global locations wants to forecast the annual sales of the next year. For this, the company collects data from all locations and gathers a huge amount of data. Since the data is accumulated from different locations, it has huge errors and fluctuations. At the same time, the company analyst did not consider testing the dataset for unit roots to spot whether the time series data is stationary.
It led to serious implications of irrelevant outcomes from the analysis. Still, the management ignored the unit's root presence and went on to make manufacturing and sales decisions, which eventually brought a reasonable loss to the company in the following year. It all happened because the non-stationarity's presence was not tested and cleared in the time series data.
Difference Between Unit Root And Stationarity
The main differences between unit root and stationarity are -
- The unit root points to a non-stationary process, whereas stationarity itself depicts a scenario defining the consistent time series dataset with no variations and fluctuations.
- Analysts conduct tests to detect unit root presence in time series. In comparison, the stationarity condition depends on the appearance of the unit root.
- Data analysts seek and prefer to achieve stationarity with no presence of unit root in time series analysis. In case a unit root is detected, the analysts try differencing the series to achieve stationarity.