Table Of Contents
What Is Tobit Regression?
A tobit regression or censored regression model refers to a category of regression models where the dependent variable’s observed range is censored. It helps in estimating linear relations between variables in case left or right censoring of the dependent variable takes place.
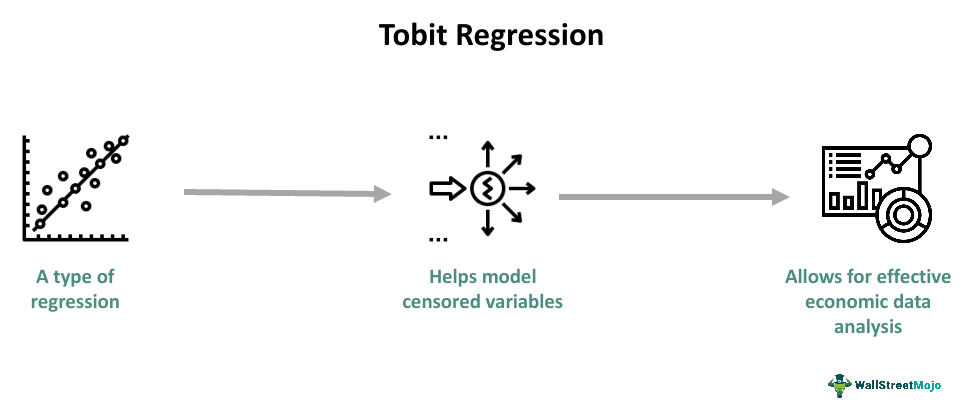
This biometric and econometric technique originally aimed to reduce the zero-inflated data issue for the observations concerning household expenses resulting from durable goods. James Tobin, an American economist, proposed this model in 1958. Censored dependent variables often arise in the field of econometrics. A common example is the supply of labor. This model is suitable if dependent variables are within a specific limit.
Key Takeaways
- The tobit regression model can be used to explain the relationship between a minimum of one independent variable and a dependent variable that is nonnegative.
- Tobit regression coefficient interpretation is identical to OLS regression coefficients’ interpretation.
- There are various benefits offered by this model. For example, one can apply it to different types of analyses, such as analysis of time series and heavy panel data. Additionally, such a model provides stability and extremely reliable estimations.
- A key tobit regression assumption is the variables’ normal distribution.
Tobit Regression Model Explained
Tobit regression models are statistical models that describe the relationship between at least one independent variable, xi and a censored continuous dependent variable. Econometrics researchers frequently utilize this tool to model censored variables. One must note that the tobit regression coefficient interpretation is the same as the interpretation of ordinary least squares (OLS) regression coefficients. That said, the linear impact is on uncensored latent variables rather than observed outcomes.
In the case of a tobit model, the variable linearly relies on xi through a vector or parameter β. It determines the relationship between the latent and the independent variables. Additionally, ui, an error term that is normally distributed, exists for the purpose of capturing random influences on the relationship. Individuals must remember that yi, the observable variable, is identical to the latent variable if the latter is 0 or more than 0.
Let us look at a few more things about this model that will help one understand the concept better.
- Individuals utilize tobit regression with censored data. This form of data is different from truncated data.
- Such a model can spot the right relationship under a maximum cluster.
- One requires a censored least absolute deviator (CLAD) indicator.
Assumptions
Let us look at the tobit regression assumptions.
- This model assumes that a latent variable exists.
- It assumes that the latent variable is unobserved over the entire range. This may happen because of censoring or truncation. If truncation takes place, persons on a particular range of that variable are not a part of the data set.
- The regression model assumes a normal distribution of variables.
Examples
Let us look at a few tobit regression examples to understand the concept better.
Example #1
A study conducted in 2008 involved using the tobit regression to analyze the data of six West African countries in the period ranging from 1995-2002. As per the empirical findings, information and communication technology (ICT) did not contribute to economic freedom within the nations selected for that study.
Moreover, in 2010, a study investigated the relationship between bank efficiency and nonperforming loans in Singapore and Malaysia using this model on data from the period between 1995 and 2000 for those nations’ banks. The results of the study indicated that the higher nonperforming loans minimize the cost efficiency, which increased nonperforming loans.
Example #2
A research paper issued in 2020 evaluated the factors significantly associated with the use of financial services among the individuals of the states in North-East India. This part of the country had a low penetration concerning financial services and banking, as reported by the different regulators. The research paper aimed to determine the factors that were acting as impediments toward using financial services and impacted the choice of not utilizing or utilizing such financial products or services.
Note that it involved using tobit regression to get a clear idea regarding the linear relation existing between the variables affecting the utilization of financial services. The model helped identify the following factors that played a crucial role in the use of financial services:
- Members’ economic status
- Financial awareness
- Financial products’ suitability
- Users’ age
- Banking correspondents’ presence
- Education of the user
Applications
Individuals and organizations can apply this regression model in an extensive range of areas. Let us look at some of them.
- People can use this model to specify and estimate models concerning dividend behavior.
- One can utilize it to evaluate innovation processes in the agriculture sector.
- Additionally, organizations can use this regression model to analyze labor dynamics.
- In business, this model helps in measuring the relationship between a firm’s efficiency and working capital management.
Advantages And Disadvantages
Let us look at the benefits and limitations of this kind of regression model.
Advantages
- Such a model helps in effectively analyzing economic data even when the sample data do not fully represent the whole population because of different reasons, such as the analysis’s nature and data availability.
- This type of statistical model pioneered areas that involve semicontinuous data within the analysis.
- The model is very stable. Moreover, it provides highly dependable estimations.
- It applies to an extensive range of analyses that include straightforward time series analysis as well as analysis of heavy panel data.
- This model offers results similar to OLS results in a certain factor, helping in establishing consistency and comparability of results.
Disadvantages
- This model is complex. Hence, individuals and organizations may find it challenging to use.
- The application of such a model is highly subjective.
- There are loopholes associated with the interpretation and application of this model.
- Questions arise regarding the model’s exactness because of the associated inconsistencies.
Tobit Regression vs Linear Regression vs OLS Regression
The concepts of tobit, linear, and OLS regressions can be confusing for one new to the world of finance. To clearly understand their purpose and meaning, one must know their key differences, highlighted in the table below.
Tobit Regression | Linear Regression | OLS Regression |
---|---|---|
This model helps estimate the linear relation between multiple variables in the case of right or left censoring of the dependent variable. | It helps predict the value of a variable on the basis of another variable’s value. | This type of regression helps estimate the relation between a couple of ratio or interval-level variables. |
It is able to handle censored data. | Such a model cannot handle censored data. | Results obtained using this kind of model are inconsistent if the data are censored. |