Below is a comparison of survival analysis and logistic regression, focusing on their application:
Table Of Contents
What Is Survival Analysis?
Survival analysis, also known as time-to-event analysis or event history analysis, is a statistical and analytical method used to study the time it takes for an event of interest to occur. This event can be any event with a temporal dimension, such as the failure of a machine, the onset of a disease, or various financial events like default on a loan, bankruptcy, or a stock price hitting a certain level.
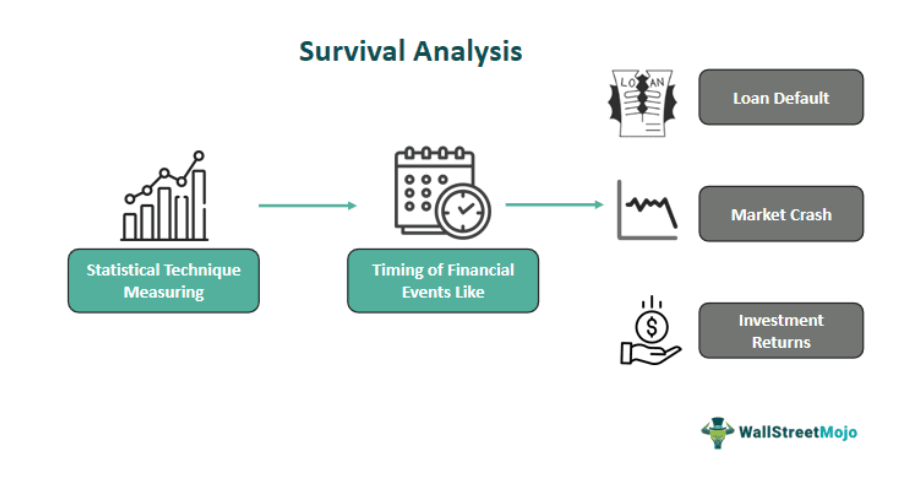
It predicts the likelihood and timing of default events for loans, bonds, or other financial instruments. Financial institutions can develop models to estimate the probability of default for individual borrowers or issuers. Financial institutions and credit rating agencies use survival analysis to assess credit risk.
Key Takeaways
- Survival analysis is a statistical method tailored to model the timing of financial events. It is especially valuable where timing is often as crucial as the event itself.
- It accommodates censored data, where events have not occurred for all observations. It allows for a more realistic analysis of financial events.
- It is essential for credit risk assessment, helping financial institutions predict and manage the timing of loan defaults. It also guides lending decisions and risk pricing.
- It accounts for time-varying covariates, making it suitable for assessing changing risk factors over time, an essential consideration.
Survival Analysis Explained
Survival analysis is a specialized statistical technique that explores and predicts the timing of crucial financial events and outcomes. Outcomes involve loan defaults, bond defaults, market crashes, or investment returns.
The origins of survival analysis can be attributed to actuarial science and the insurance industry. Historically, it was initially developed to calculate the longevity of insurance policyholders and estimate payouts. Over time, financial experts recognized its broader applicability and adapted it to assess financial risks.
From this perspective, it seeks to achieve several goals:
- Risk Quantification: It enables financial institutions and investors to quantify and manage risks. It models the probability of financial events occurring within specific timeframes.
- Default Prediction: For lenders, it is a powerful tool for predicting loan defaults and credit risk. By analyzing historical data, they can estimate when borrowers are likely to default, helping make sound lending decisions.
- Portfolio Optimization: It assists financial institutions in optimizing portfolios by evaluating the expected duration of assets and investments.
- Investment Insights: Investors use it to assess the timing of market events and make informed decisions regarding investments, factoring in the probability of reaching specific financial goals.
Assumptions
Survival analysis, like in any other application, is based on certain assumptions to make meaningful predictions and inferences about the timing of financial events. Here are the key assumptions when applying it in a financial context:
- Non-Informative Censoring: It assumes that censoring (i.e., the event of interest not occurring during the study period) is non-informative. This means that the reasons for censoring (e.g., data on a loan default being unavailable because the loan is still active) are not related to the likelihood of the event occurring.
- Constant Hazard: It typically assumes that the hazard rate, which represents the instantaneous risk of the event occurring, given that it has not occurred yet, remains relatively constant over time.
- Independence: It is assumed that the survival times (times to financial events) of different individuals or financial instruments are independent of each other. In reality, correlations and dependencies might exist in financial markets or portfolios, but for simplicity, survival analysis often assumes independence.
- Proportional Hazards (Cox Model): When using the Cox proportional hazards model, an essential assumption is that the hazard ratio between two groups remains constant over time.
- No Competing Risks: It assumes that there is only one type of event of interest. This might only sometimes hold. For example, a financial instrument might be subject to both default and prepayment. In such cases, the assumption of no competing risks may not be satisfactory, and more complex models are required.
- Exogeneity: Financial events should not affect the covariates or predictors used in the survival analysis. If an event itself impacts the variables used for modeling, it can violate this assumption.
- Homogeneous Populations: It often assumes that the population under study is homogeneous, meaning that all individuals or financial instruments share the same characteristics or risk factors.
Types
Survival analysis encompasses several types or variations, each tailored to address specific financial applications and challenges. Here are some common types of it from a financial perspective:
- Kaplan-Meier Survival Analysis: The Kaplan-Meier estimator is a fundamental tool for estimating the survival function, which represents the probability of a financial event not occurring up to a specific time. This analyzes the survival or default rates of financial instruments, such as bonds or loans, and compares different groups of financial assets.
- Cox Proportional Hazards Model: The Cox model analyzes the impact of various factors (covariates) on the timing of financial events, like loan defaults or bankruptcy. It assumes that the hazard ratio between different groups remains constant over time, making it suitable for modeling risk factors and predicting default probabilities.
- Parametric Survival Models: Parametric models, like the Weibull or exponential distributions, make explicit assumptions about the functional form of the hazard rate. They estimate the time of financial events, incorporating specific assumptions about the event's distribution.
- Censored Data Handling: It often deals with censored data, where events haven't occurred for all observations in the dataset. Specialized techniques like the Turnbull and Nelson-Aalen estimators handle censored data and provide more accurate survival estimates.
- Accelerated Failure Time Models: These models assume that the logarithm of the survival time is linearly related to covariates. They quantify how financial events affect other factors, helping assess the impact of different variables on the timing of the event.
- Frailty Models: These models account for unobserved heterogeneity among financial instruments or individuals. Frailty models help in understanding how unobserved factors may influence the timing of events like loan defaults.
Examples
Let us understand it better via the following examples.
Example #1
Suppose a fictional bank, "FuturaBank," wants to predict loan defaults more accurately using survival analysis. They collect data on various loans, including loan amounts, interest rates, credit scores, and employment histories. The event of interest is the default on a loan.
FuturaBank employs the Cox Proportional Hazards model to analyze the data. After setting up the model, they found that higher interest rates and lower credit scores significantly increase the risk of loan default. The model also reveals that the risk of default is relatively stable over time.
By applying survival analysis, FuturaBank can estimate the probability of loan default for individual borrowers throughout the life of their loans. This helps the bank make better-informed lending decisions, set interest rates more accurately, and manage credit risk more effectively.
Example #2
In a recent development of 2023, Tune Insight, an AI-driven financial technology company, has successfully secured $3.4 million in funding. The company specializes in credit risk analysis and offers a unique approach to the world of finance. Using advanced algorithms, including survival analysis, Tune Insight provides an innovative solution for predicting credit risk and analyzing loan defaults. This new funding round, led by prominent investors, will further strengthen the company's mission to revolutionize credit risk assessment.
Survival analysis, a statistical method crucial in financial risk assessment, is instrumental in Tune Insight's predictive capabilities. By modeling the timing of financial events, such as loan defaults, Tune Insight aims to empower financial institutions with more accurate tools for credit scoring and portfolio management. This recent funding injection bolsters their innovative approach, helping to refine the art of credit risk assessment in the financial industry.
Applications
Survival analysis has various applications, providing valuable insights and predictive capabilities for understanding and managing financial risks. Some of its critical applications include:
- Credit Risk Assessment: Financial institutions use it to assess and predict the risk of loan defaults and credit events. By modeling the time to default for borrowers, they can make more informed lending decisions and set appropriate interest rates based on individual creditworthiness.
- Default Prediction: It predicts the likelihood and timing of bond and loan defaults. This is essential for both creditors and investors in assessing the creditworthiness of issuers and making investment decisions.
- Loan Portfolio Management: Banks and other lending institutions apply such analysis to optimize their loan portfolios. They can analyze the expected time to default for different types of loans, helping them diversify their portfolios and allocate capital efficiently to manage risk.
- Risk Assessment for Investments: Investors use this analysis to assess the risks associated with various financial investments, such as stocks and bonds. It allows them to model the time-to-market events, such as reaching a specific price level, and make more informed investment choices.
- Market Event Analysis: It analyzes the timing of significant market events like stock market crashes or changes in asset prices. Understanding the timing of such events is essential for traders, investors, and risk managers to respond effectively.
- Customer Lifetime Value: Financial institutions use this analysis to estimate the lifetime value of a customer. By modeling the duration of customer relationships and predicting churn, they can develop strategies to retain profitable customers and acquire new ones.
- Insurance Risk Assessment: Insurance companies utilize this analysis to assess the timing of insurance claims, payouts, and policy lapses. This is critical for pricing insurance products and managing their financial liabilities.
Advantages And Disadvantages
Advantages
- Time-Dependent Analysis: It allows for the modeling of events that occur over time, which is crucial, where timing is often as important as the event itself.
- Censors Data: It can handle data where events have not occurred for all observations, making it suitable for scenarios where default or other financial events haven't happened to all subjects.
- Dynamic Risk Assessment: It accounts for time-varying covariates, allowing for the assessment of changing risk factors over time, which is essential in the financial sector where risk factors can evolve.
- Flexible Model Selection: A variety of models and techniques are available, enabling flexibility in selecting the most suitable model for a specific financial application.
- Quantifying Time-to-Event Risk: It provides a way to quantify and express the timing of financial events, enabling risk quantification, pricing, and portfolio management.
Disadvantages
- Censoring and Missing Data: Handling censored data can be complex, and the analysis may be sensitive to how censored observations are treated, potentially introducing bias.
- Assumption Sensitivity: The results can be sensitive to model assumptions, such as the proportional hazards assumption in the Cox model. Violation of these assumptions can lead to inaccurate predictions.
- Complexity: Survival analysis models can be more complex and may require advanced statistical knowledge, which could be a disadvantage for those without the necessary expertise.
- Data Quality: The quality of data is crucial. Incomplete or inaccurate data can lead to unreliable results, and data preparation can be time-consuming.
- Limited Interpretability: Survival analysis models might be less intuitive for non-statistical audiences, making communication of results challenging.
Survival Analysis vs Logistic Regression
Aspect | Survival Analysis | Logistic Regression |
---|---|---|
1. Focus | Time to event (e.g., loan default) Time-to-event data, modeling event timing | Binary outcome (e.g., default: yes/no) Quantifies and manages risks associated with the timing of events, helping with risk pricing and management |
2. Censoring | Handles censored data (events that have not occurred for all subjects) | Inefficient for censored data, as it doesn't account for time-to-event information |
3. Time Dependency | Models the timing of events, essential for finance where timing matters (e.g., predicting default at a specific time) | Doesn't inherently capture the temporal aspect, as it provides probabilities but not timing information |
4. Assumptions | Assumes proportional hazards or other distribution assumptions | Assumes linearity and independence among predictor variables |
5. Interpretability | Hazard ratios or survival curves may require specialized interpretation
| Odds ratios are more interpretable and straightforward |
6. Applications | Assess default risk, bond default prediction, credit scoring, loan portfolio management | Binary classification tasks such as credit approval, fraud detection, customer churn prediction |
7. Risk Assessment | Quantifies and manages risks associated with the timing of events, helping with risk pricing and management. | Focuses on the likelihood of an event without explicitly considering the timing aspect |