Table Of Contents
What Is The Statistical Test?
Statistical test refers to a systematic implementation of mathematical calculations and interpretation skills for determining whether the observed differences or relationships in the sample will likely reflect actual patterns in the entire population. These tests help researchers and analysts to make predictions and well-informed decisions.
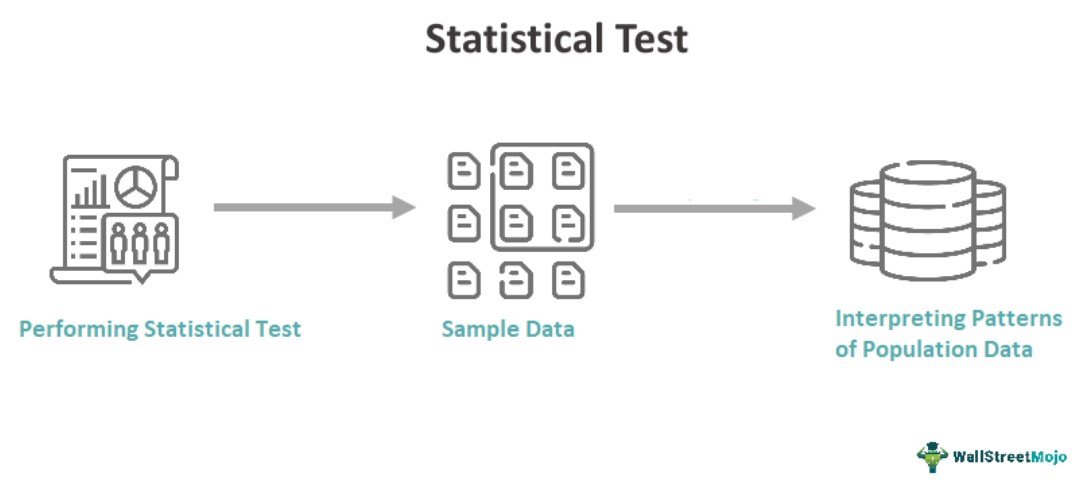
The selection of a particular statistical measure is majorly based on the type of data variables. There are many statistical tests, such as t-test, chi-square, ANOVA, Kruskal-Wallis test, and regression analysis, each serving different needs. It is a crucial parameter in economics, business, investment, finance, research, and medical science for gauging trends and possibilities.
Key Takeaways
- Statistical tests are methods used to analyze and draw inferences from the sample data, such as determining whether an assertion or hypothesis stands true or false for the population.
- It even aids in sensible decision-making and risk assessment in various fields like research, economics, finance, business, investment, medicine, and social science.
- Researchers and analysts conduct numerous statistical tests; however, some popular ones are the T-test, Z-test, ANOVA, Chi-square test, correlation analysis, and regression analysis.
Statistical Test ExplainedÂ
Statistical tests hold significance due to their ability to analyze data, draw meaningful conclusions, and make valid inferences about populations based on sample data. These tests facilitate comparisons between groups or variables, helping identify differences, similarities, or relationships. Moreover, these measures play a crucial role in decision-making processes, such as clinical trials and quality control, ensuring that conclusions are reliable and significant.
These measures are indispensable tools in scientific research, business, and various other fields, guiding researchers and professionals in making informed and evidence-based decisions. The process begins with the collection of relevant data for analysis. When the researcher has sufficient data, they can proceed with the hypothesis testing by selecting a suitable statistical method. The analysts derive the outcome by either accepting or rejecting the null hypothesis.
However, statistical measures are prone to various limitations. These are sensitive to the sample size and rely on assumptions that may not be steadfast in real-world scenarios. Moreover, misinterpreting the results or assuming that correlation implies a specific cause can lead to incorrect conclusions. Personal biases and errors often influence the statistical results and interpretation. Also, sometimes, experiments that would provide strong evidence are unethical or impractical to conduct, limiting the ability to draw definitive conclusions.
FactorsÂ
Several aspects impact the outcomes and interpretation of a statistical test. Thus, it becomes critical to consider the following components for conducting robust statistical analysis and drawing valid conclusions from the data:
- Sample Size: Larger sample sizes generally provide more accurate and reliable results, increasing the power of statistical testing.
- Significance Level: This represents the probability of rejecting a true null hypothesis. Commonly used values are 0.05 and 0.01, indicating a 5% or 1% possibility of a Type I error.
- Effect Size: It represents the magnitude of the difference or relationship being studied. A larger effect size increases the likelihood of detecting a significant result.
- Variability: It denotes the extent to which the data points in a data set differ from the mean. Higher variability can make it harder to detect significant differences.
- Data Type: Different tests are suited for different types of data and research questions—for instance, t-tests for comparing means, chi-square tests for categorical data, and correlation tests for relationships.
- Assumptions: Most tests rely on particular assumptions such as normal distribution or homogeneity of variance. Violations of these assumptions can affect the results.
TypesÂ
Many specialized statistical tests are used in different research and analysis fields. The choice of a specific measure depends on the research question, the type of data, and the assumptions underlying the test. Let us discuss some common ones below:
- T-Test: A t-test is particularly useful when dealing with numerical data that follows an approximately normal distribution. The t-test calculates a t-value, which is then compared to a critical value to gauge the statistical significance of the difference between the means of the two groups.
- Chi-Square Test: The Chi-square test is employed to research the connection between two categorical variables in a given sample. Unlike parametric statistical tests, the Chi-square test does not rely on specific assumptions about the data distribution, making it a non-parametric test.
- Fisher's Exact Test: The Fisher's statistical test assesses nonrandom associations between two categorical variables in a 2x2 contingency table. It is valuable when dealing with small sample sizes where the assumptions of the chi-square test might not be valid.
- ANOVA (Analysis of Variance): The ANOVA statistical test compares means across three or more groups. It proves valuable when there's a need to simultaneously analyze differences among multiple independent groups.
- Regression Analysis: This is utilized to study the association between a dependent variable and the corresponding independent variables. It aids in foreseeing the dependent variable's value with the independent variables' help.
- Correlation Analysis: The correlation statistical test helps to reckon the robustness and direction of the connection between two or more continuous variables. It measures how changes in one variable are associated with changes in another variable.
- Mann-Whitney U Test: The Mann-Whitney U test is a non-parametric statistical test calculator used to assess whether there is a significant difference between the distributions of two independent groups. It is useful when the data doesn't meet the assumptions of normality or when dealing with ordinal, interval, or ratio data.
ExamplesÂ
Statistical tests have gained exposure in both scientific research and economic analysis. We can see its application in businesses and finance to improve operations and make decisions. Many statistical methods are available, each capable of answering specific types of research questions based on the nature of the data and the hypotheses under the test.
Here are some practical examples of these tests:
Example #1
If a teacher wants to compare the average test scores of two different classes to see if one class performed significantly better, he could use a t-test. The null hypothesis might be that there is no difference in the mean test scores between the two classes, and the alternative hypothesis would be that there is a significant difference.
Example #2
Suppose a person wants to determine if there is a significant relationship between gender (male or female) and the preference for a particular brand of soft drink among a group of people. They could use a chi-square test to analyze the data and determine if there is a statistically significant association between gender and soft drink preference. The null hypothesis might be that there is no association, while the alternative hypothesis would suggest an association between gender and soft drink preference.