Statistical Process Control
Last Updated :
-
Blog Author :
Edited by :
Reviewed by :
Table Of Contents
Statistical Process Control (SPC) Meaning
Statistical process control (SPC) is a method used in quality management to monitor and control industrial processes through statistical techniques. The purpose of SPC is twofold: first, it enables the detection of process variations in real-time, allowing for immediate corrective actions to prevent defects. Second, it provides valuable insights into the overall process performance.
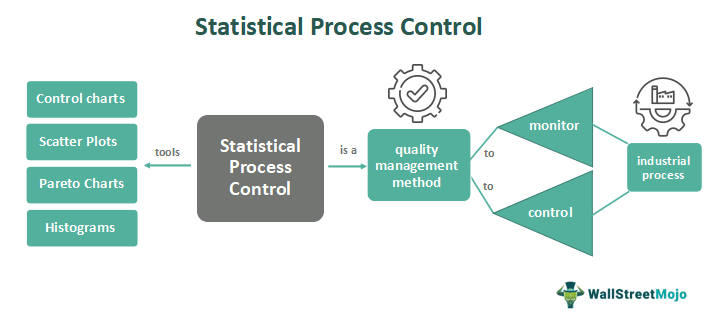
SPC ensures product quality, enhances customer satisfaction, and increases operational efficiency. By monitoring process parameters, identifying trends, and detecting anomalies, SPC helps organizations maintain process control, minimize defects, and achieve consistent output. It provides a scientific and data-driven approach to quality management, supporting informed decision-making and fostering a culture of continuous improvement.
Table of contents
- Statistical Process Control (SPC) is a methodology used to monitor and control industrial processes by applying statistical techniques to analyze process data.
- SPC helps detect real-time process variations, allowing immediate corrective actions to prevent defects and maintain process stability.
- It provides insights into process performance, enabling continuous improvement efforts and waste reduction.
- SPC ensures consistent product quality, enhances customer satisfaction, and drives operational efficiency through data-driven decision-making.
Statistical Process Control ExplainedÂ
Statistical Process Control (SPC) is a methodology to monitor and control industrial processes. It involves applying statistical techniques to analyze data collected during production to understand and improve process performance.
Statistical techniques are used to identify and eliminate variations that could lead to defects or non-conformities. SPC is widely employed across various industries to ensure consistent product quality and process stability.
The core principle of SPC is that most process variations can be categorized as either common cause variations or special cause variations. Common cause variations are inherent to the process and expected to occur within certain limits. In contrast, special cause variations are caused by identifiable factors not part of the normal process.
Steps
Let us look at the steps involved in implementing SPC:
- Define the Process: Clearly define the process or the specific aspect that will be monitored and controlled using SPC. Then, identify the critical process parameters and quality characteristics of the product or service.
- Collect Data: Establish a data collection plan to gather relevant data about the process. Then after, determine what data needs to be collected, how frequently, and at what point. Ensure that the data collected is representative of the process and its variation.
- Construct Control Charts: Select appropriate control charts based on the data collection type (e.g., variable data or attribute data). Plot the collected data on the control chart, setting appropriate control limits based on statistical analysis.
- Monitor the Process: Continuously collect data and update the control chart. Monitor the process performance by observing the data points plotted on the control chart. Monitor for out-of-control signals, including data points outside the control limits or non-random patterns.
- Analyze Data: Conduct statistical data analysis to distinguish between common cause and special cause variations. Identify any trends, patterns, or abnormal signals in the data. Investigate special cause variations to understand their root causes.
- Take Corrective Actions: When special cause variations are detected, take appropriate corrective actions to address the identified root causes. This may involve process adjustments, equipment maintenance, operator training, or other necessary actions to bring the process back under control.
- Sustain and Improve: Continuously monitor the process and use the feedback from SPC to drive improvements. Regularly review and update control charts as needed. Implement preventive measures to avoid future special cause variations and optimize the process for improved performance and quality.
Tools
Let us look at important tools utilized in SPC:
- Control Charts: Control charts are graphical representations that plot process data over time, allowing for the detection of variations. They help identify common causes and special cause variations, making it easier to determine if a process is stable or out of control.
- Histograms: Histograms display the data distribution by grouping it into intervals or bins and showing the frequency of data points within each bin. They provide a visual representation of data distribution, allowing for the identification of patterns and potential issues.
- Scatter Plots: Scatter plots examine the relationship between two variables. They plot paired data points on a graph, enabling the analysis of correlations, trends, or patterns between variables, which can provide insights into process behavior.
- Pareto Charts: Pareto charts help prioritize and focus improvement efforts by identifying and ranking the most significant factors contributing to defects, errors, or issues. Thus they display the frequency or impact of each factor in descending order, allowing teams to tackle the most critical problems first.
- Cause-and-Effect (Fishbone) Diagrams: Fishbone diagrams visually illustrate the potential causes of a problem or variation. They identify different categories of potential causes (such as people, processes, equipment, materials, etc.) and visually represent the relationships between these causes and the problem under investigation.
- Statistical Analysis Software: Various statistical analysis software, such as Minitab, JMP, or Excel with built-in statistical functions, are commonly used to perform calculations, generate control charts, conduct data analysis, and obtain statistical insights.
Examples
Let us look at examples to understand the concept better.
Example #1
As per an article by QualityMag, implementing Statistical Process Control (SPC) can be advantageous for manufacturers facing high process variation and overfill. Since, SPC allows manufacturers to gain insights into the true sources of variation, leading to permanent corrective actions and cost savings in their operations. By utilizing Xbar/R Control Charts, a specific SPC method, manufacturers can effectively reduce fill weight variation, maintain centerlines, and minimize the need for rework.
While capability analysis and SPC methods significantly reduce overfill, it is crucial to supplement these approaches with lean tools, techniques, and methods. Incorporating these charts with lean principles helps foster a continuous flow of materials, increases equipment uptime, and decreases changeover time. Through this combined effort, manufacturers can achieve savings and improve overall throughput.
Example #2
Let us consider a beverage manufacturing company that produces carbonated soft drinks and wants to ensure consistent carbonation levels in its products. Thus, to implement SPC, the company sets up a monitoring system that regularly collects data on the carbonation level of randomly selected bottles from the production line. The collected data is then analyzed using statistical techniques.
Over time, the SPC analysis reveals occasional variations in the carbonation level, indicating a potential issue in the production process. The company identifies a specific machine responsible for injecting carbon dioxide into the beverages and notices that it occasionally malfunctions, resulting in higher or lower carbonation levels.
With this information, the company takes immediate corrective action, such as repairing or calibrating the malfunctioning machine whenever a deviation is detected. The company can promptly address these variations and maintain the desired carbonation level, ensuring consistent product quality. Additionally, the SPC data allows the company to track the performance of the carbonation process over time, identify trends, and make process improvements.
Advantages And Disadvantages
Let us look at the advantages of Statistical Process Control (SPC):
- Early Detection of Issues: SPC allows for the early detection of process deviations and non-conformities, enabling prompt corrective actions to prevent the production of defective products or the delivery of poor-quality services.
- Data-Driven Decision Making: SPC provides objective and data-driven insights into process performance, allowing organizations to make informed decisions based on statistical analysis rather than relying solely on intuition or subjective judgments.
- Continuous Improvement: SPC facilitates a culture of continuous improvement by providing valuable information about process capability, trends, and opportunities for optimization. Thus, it supports evidence-based decision-making for process enhancements.
Let us look at the disadvantages of Statistical Process Control (SPC):
- Statistical Expertise: SPC relies on statistical analysis techniques, which may require specialized knowledge and expertise. Hence, organizations may need to invest in training or employ statistical experts to effectively implement and interpret SPC results.
- Limitations in Complex Processes: SPC may be less effective in highly complex processes or those with multiple interacting variables. Thus, it may not capture all nuances and interactions, potentially leading to incomplete insights or overlooking critical factors.
- Overreliance on Historical Data: SPC primarily relies on historical data for analysis and decision-making. While it provides valuable insights based on past performance, it may not account for unforeseen changes or future variations.
Statistical Process Control vs Statistical Quality Control
Let us look at the differences between Statistical Process Control (SPC) and Statistical Quality Control (SQC):
Parameters | Statistical Process Control (SPC) | Statistical Quality Control (SQC) |
---|---|---|
Focus | Control and monitoring of individual processes | Overall quality management and control of multiple processes |
Scope | A narrow focus on a specific process or operation | Broader focus on the entire quality management system |
Purpose | Monitor process variations and ensure process control | Monitor and improve overall product/service quality |
Data Collection | Collects and analyzes data from a single process | Collects and analyzes data from multiple processes |
Statistical Tools | Control charts, histograms, scatter plots, etc. | Pareto charts, cause-and-effect diagrams, etc. |
Control Limits | Set based on process-specific variability | Set based on quality standards and customer requirements |
Key Application | Monitoring and controlling individual process variables such as temperature, pressure, etc. | Ensuring overall product quality, customer satisfaction, and adherence to quality standards |
Frequently Asked Questions (FAQs)
Yes, SPC can be applied to both manufacturing and service industries. It is commonly associated with manufacturing processes. However, the principles, and tools of SPC can also be adapted to service-oriented processes.
Yes, Statistical Process Control can be beneficial for small businesses. It may require some initial training and data collection investment. SPC can help small businesses improve their processes, reduce variations, and enhance product quality. It enables them to detect and address issues early on, leading to cost savings and increased customer satisfaction.
Unlike traditional quality control methods that inspect finished products, SPC focuses on monitoring and controlling the underlying process variables in real time. By analyzing process data using statistical techniques and control charts, SPC helps identify variations and trends that can lead to defects.
Recommended Articles
This article has been a guide to Statistical Process Control (SPC) and its meaning. Here, we explain its tools, examples, steps, advantages and disadvantages. You may also find some useful articles here -