Table Of Contents
What Is Stationary In Time Series?
A stationary in time series is the element of a time series that remains constant in its properties regardless of the observation time. Long-term, predictable patterns are generally absent from this time series. Time plots demonstrate the series with horizontal behavior (with constant variance, but some cyclic behavior may be conceivable).
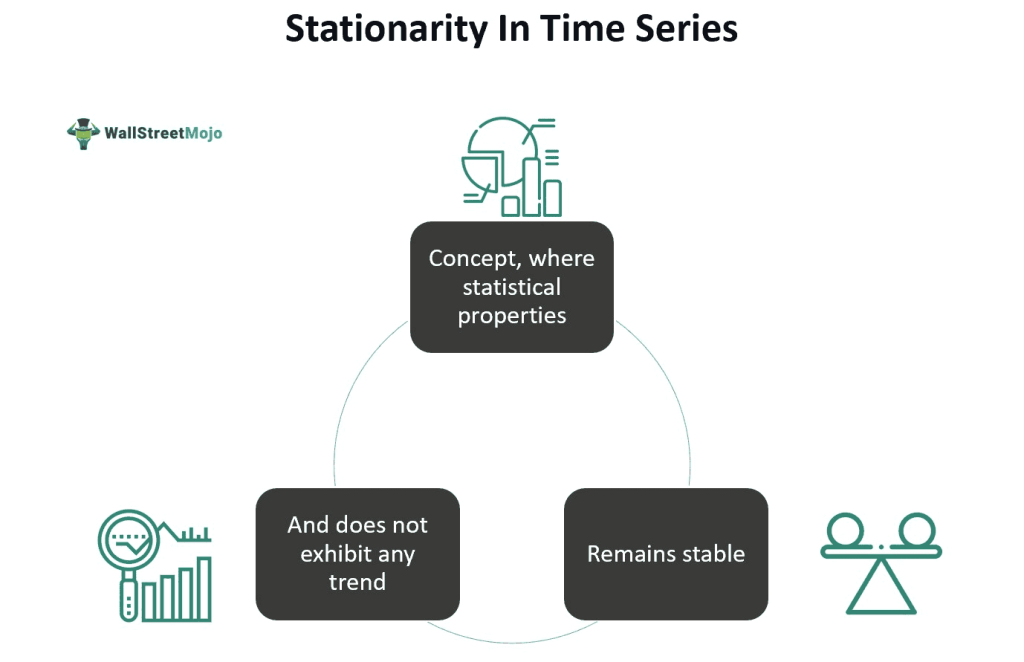
Stationary factor refers to the statistical properties of a process that does not change its way of changing over time, generating a time series. This is important because stationary processes are more accessible to analyze and investigate. Hence, this makes it easier to model and investigate these processes, making them a more comprehensive understanding of reality.
Key Takeaways
- A stationary time series is the element of a time series that remains constant in its properties regardless of the observation time.
- It is considered beneficial due to its simplicity to examine. Stationary processes are a subset of a more prominent family of potential reality models. It is significantly simpler to model and research this subclass.
- Three requirements must be met for a time series to be deemed stationary consistent mean with time, consistent variance with time, and consistent autocorrelation with time.
- Thus, it is a crucial concept in time series analysis, influencing how data is perceived and predicted.
Stationarity In Time Series Explained
Stationarity in time series data is the element of a series that remains constant throughout time in terms of its statistical attributes, such as mean, autocorrelation, variance, etc. In its most basic form, stationarity refers to the consistency of a process's statistical characteristics across time in the generation of a time series. The series may change over time. But, the manner in which the changes are unveiled does not change over time. It is considered beneficial due to its simplicity to examine. Stationary processes are a subset of a more prominent family of potential reality models. Therefore, it is significantly simpler to model and research this subclass.
A stationary factor in a time series has constant statistical properties over time, making it easy to predict through statistical forecasting methods. These properties include factors of mean, autocorrelation, and variance. A time series can be rendered stationary (approximately) through mathematical transformations. Such stationarized series are relatively easier to predict. The predictions for the stationarized series can later be "untransformed" by undoing or reversing the previous mathematical transformations used to obtain predictions for the original series.
Stationarising a time series allows for the acquisition of meaningful sample statistics like variances, means, and correlations with other variables. These statistics are helpful in describing future behavior only if the series is stationary. For instance, if the mean and variance will increase with the sample size if the series is continuously rising, this will lead to an underestimation in subsequent periods. If the mean and variance are well-defined, then its relationships with other variables are also better defined. It's advisable to be cautious when extrapolating regression models used in non-stationary data.
Conditions
Three requirements must be met for a time series to be deemed stationary:
- Consistent Mean with Time: We can estimate the mean using all of the data if we assume a constant mean.
- Consistent Variance with Time: Time does not affect variation. Variance is finite and constant.
- Consistent Autocorrelation with Time: The significance of this is that the correlation between two points is solely dependent on their separation in time, not on their actual location.
Examples
Let us look at a few examples to understand the concept better.
Example #1
Let’s say Dan, an investor, is analyzing a company's stock prices over time to determine if they exhibit stationarity, which refers to the constant statistical properties of the stock prices, such as mean and variance. He does this because stationarity is crucial for investors as it allows them to make reliable predictions and decisions based on historical data. If stock prices are stationary, it implies that past patterns are likely to continue in the future, allowing him to use time series analysis techniques like forecasting models to make informed investment choices.
Dan chooses to examine stationarity to understand stock price behavior and identify underlying trends. He does this by assessing the reliability of historical data for investment decisions and developing strategies based on observed patterns. Therefore, this example of stationarity in time series forecasting benefits Dan in making informed decisions.
Example #2
Let's say Finalis Ltd, an investment banking company, analyzes interest rates of investments over time to determine if they exhibit stationarity. Stationarity is crucial for the pricing and valuation of financial instruments, as it ensures that the market conditions remain stable. This allows for accurate pricing, as well as risk assessment and investment strategy formulation. The company chooses to study stationarity in interest rates to assess market stability, make reliable predictions, evaluate pricing models, manage risk effectively, and make informed decisions regarding portfolio management, asset allocation, and hedging strategies.
Importance
Stationarity in time series data is a crucial concept in time series analysis, influencing how data is perceived and predicted. Most time series models work with an assumption that each point is independent of the other points, and this is evident with the past stationary data sets. This stability is essential for many time series analysis techniques, such as the Auto-Regressive Integrated Moving Average (ARIMA) model for forecasting. Weak stationarity in time series can show inaccuracies. This is because it cannot faithfully reflect underlying patterns and may result in incorrect or misleading results.
Non-stationarity data may give the impression of a greater connection between variables due to seasonal or trend factors in the data. They help to facilitate meaningful comparisons between different periods. Therefore, achieving stationarity improves the forecasting accuracy of models, especially in capturing the underlying dynamics of the time series.
Stationarity vs Non-Stationarity In Time Series
The following are the differences between both concepts:
- A stationary time series' statistical characteristics are unaffected by the observational point in time. At the same time, a non-stationary time series' statistical characteristics depend on the point in time at which it is recorded.
- The stationary series's mean, variance, and other elements don't change. But, in a non-stationary time series, mean, variance and other characteristics vary over time.
- The conclusions drawn from the stationary series analysis are reliable, and weak stationarity in time series can be inaccurate. Inferences drawn from the study of a non-stationary series may need to be more accurate.
- Stationary time series do not exhibit seasonality, trends, and other features.
In contrast, if a series shows trends or seasonality, it is considered non-stationary.