Spearman Rank Correlation Coefficient
Published on :
21 Aug, 2024
Blog Author :
N/A
Edited by :
Ashish Kumar Srivastav
Reviewed by :
Dheeraj Vaidya
What Is The Spearman Rank Correlation Coefficient?
The Spearman rank correlation coefficient, often denoted as ρ (rho), is a statistical measure that assesses the strength and direction of the relationship between two variables. This method is beneficial when the data is in the form of ranks or ordinal scales rather than continuous variables. Instead of considering the actual values, Spearman's correlation focuses on the order or ranking of the data points.
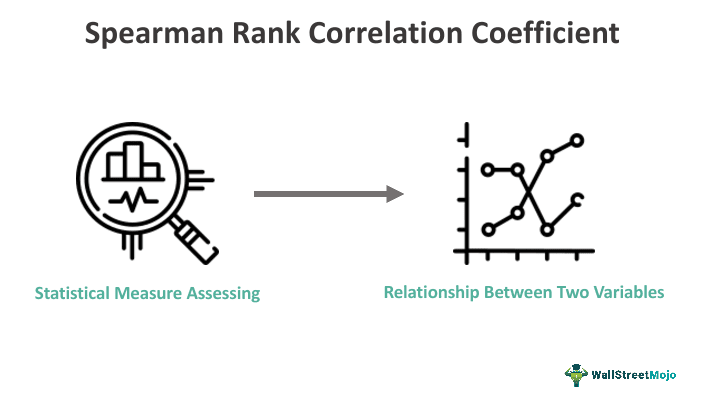
It aims to quantify the strength and direction of the monotonic association between two variables. This provides insight into whether the variables move together in terms of their ranks. Spearman's correlation is less sensitive to outliers compared to Pearson's correlation. It makes it a valuable tool for analyzing non-linear relationships and data sets with outliers or skewed distributions.
Table of contents
- Spearman's rank correlation coefficient measures the strength and direction of the monotonic association between two variables based on their ranks.
- It is ideal for non-parametric data or ordinal variables. It is especially true when data doesn't meet the assumptions of parametric tests like Pearson's correlation.
- Less sensitive to outliers and extreme values compared to Pearson's correlation, providing a more stable measure of association.
- It involves ranking data, computing differences between ranks, and applying a specific formula to determine the correlation coefficient.
Spearman Rank Correlation Coefficient Explained
Spearman's rank correlation coefficient is a statistical measure evaluating the relationship between two variables based on the ranks or orders of their values. Developed by Charles Spearman, a British psychologist, in 1904, this method addresses scenarios where the actual numerical values may not be as crucial as the relative order or ranking of those values.
Spearman's approach originates from Spearman's "law of comparative judgment," a psychological theory. It suggests that individuals can rank a set of items based on their perceived differences. Spearman introduced the concept of a correlation coefficient. It measures the extent to which two ranked sets of data correspond to each other.
This method involves converting the original data into ranks. It assigns a rank to each value based on its position relative to others. The correlation coefficient is then calculated by comparing the differences between these ranks for the paired data points. This coefficient ranges from -1 to 1, where values closer to 1 indicate a strong positive monotonic relationship, while values closer to -1 represent a strong negative monotonic relationship. A value of 0 suggests no monotonic association between the variables.
Assumptions
Spearman's rank correlation coefficient, like most statistical methods, relies on certain assumptions for its accurate interpretation and application:
- Monotonic Relationship: The primary assumption is that there exists a monotonic relationship between the variables being analyzed. This means that as one variable's values increase, the other variable's values consistently increase or decrease, although not necessarily at a constant rate. The association might be in the form of a straight line, but it doesn't have to be linear – it might follow a curve, but the key is that the relationship does not reverse direction.
- Ranking Consistency: The data should be transformed into ranks effectively, and the ranks should be assigned without bias or error. Each value should receive a unique rank, and tied values should have their ranks averaged based on their position.
- Independence: Spearman's correlation assumes that the observations are independent of each other. This means that the ranking or order of one observation should not be influenced by or dependent on the ranking of another observation.
- Homogeneity of Variance: While Spearman's correlation is robust to outliers, the variability in the differences between ranks should remain relatively consistent across the range of values for accurate interpretation.
Formula
The formula for Spearman's rank correlation coefficient quantifies the strength and direction of the relationship between two variables based on their ranks. The calculation involves several steps:
- Ranking the Data: For each variable, convert the actual values into ranks. Ranks are assigned to each value based on its position when the data is sorted in ascending order. Tied values are given the average of their ranks.
- Calculating Differences: Determine the differences between the ranks of corresponding pairs of data points. Subtract one rank from the other for each paired observation.
- Square the Differences: Square each of these differences. Squaring eliminates the impact of the sign (positive or negative) and emphasizes the relative magnitude of the differences.
- Summation and Application of the Formula: The Spearman correlation formula is ρ=1−6∑d2/n(n2−1) where ρ is the Spearman correlation coefficient, d represents the differences between the ranks of paired observations, and n is the number of observations. The term 6/n(n2−1) normalizes the sum of squared differences.
Examples
Let us understand it better with the help of examples:
Example #1
Let's consider the exam scores of two students, Lily and Sam, in three subjects: Mathematics, Science, and English.
Lily's scores: Mathematics: 80 Science: 70 English: 90
Sam's scores: Mathematics: 75 Science: 85 English: 8
Step 1: Convert scores to ranks for each student in each subject, dealing with ties by averaging ranks.
Lily's ranks: Mathematics: 2 Science: 3 English: 1
Sam's ranks: Mathematics: 3 Science: 1 English: 2
Step 2: Calculate the differences between the ranks of corresponding pairs.
Differences:
Mathematics: ∣2−3∣=1∣2−3∣=1
Science: ∣3−1∣=2∣3−1∣=2
English: ∣1−2∣=1∣1−2∣=1
Step 3: Square these differences and sum them.
The sum of squared differences: 12+22+12=6
Step 4: Apply the Spearman's correlation formula.
Given n = 3 (number of subjects):
ρ = 1−6×6 / 3(32−1) = 1−36/24=1−1.5 = −0.5
Example #2
Suppose a small-town ice cream shop, "Scoops & Smiles," offers a variety of ice cream flavors. The owner, Sarah, wants to understand if there's a correlation between the popularity of flavors and their ranking on the menu. She ranks her top 5 ice cream flavors based on sales over a month, with one being the most popular and five the least popular:
- Chocolate (Rank 1)
- Vanilla (Rank 3)
- Strawberry (Rank 2)
- Mint Chip (Rank 4)
- Butter Pecan (Rank 5)
Now, Sarah wants to calculate Spearman's rank correlation coefficient to see if there's a significant relationship between flavor ranking and sales.
The calculation involves finding the differences between the ranks, squaring them, and then using the formula. In this case, the calculated Spearman's correlation coefficient might indicate a positive correlation, suggesting that as the ranking increases (from 1 to 5), the sales might also increase or decrease accordingly.
Applications
Spearman's rank correlation coefficient finds applications in various fields due to its ability to measure the strength and direction of monotonic relationships between variables. Some critical applications include:
- Psychology and Social Sciences: It helps analyze correlations between non-parametric data, such as assessing the relationship between test scores or rankings in surveys.
- Market Research: Useful in determining consumer preferences and rankings of products or services, aiding in market segmentation and understanding customer behaviors.
- Medicine and Health Sciences: It assists in examining associations between non-parametric medical data, such as ranking the effectiveness of treatments or understanding the relationship between risk factors and health outcomes.
- Finance and Economics: Used to analyze rankings or orders of financial data, like assessing the correlation between stock rankings or credit ratings.
- Biological Sciences: Employed in ecological studies to assess species diversity or to understand the relationship between the ranking of specific traits in organisms and their ecological success.
Advantages And Disadvantages
Following is a representation of the advantages and disadvantages of using Spearman's rank correlation coefficient:
Advantages | Disadvantages |
---|---|
Does not require data to be normally distributed | Sensitivity to outliers might affect results |
Applicable to ordinal and non-parametric data | Less precise for small sample sizes |
Robust to outliers and extremes | Loss of information due to ranking |
Provides a measure of monotonic relationship | Might not capture non-monotonic associations |
Not sensitive to the scale of measurement | Computationally more intensive than Pearson correlation |
Spearman Rank Correlation Coefficient vs Pearson Correlation Coefficient
Below is a comparison of Spearman's Rank Correlation Coefficient and Pearson Correlation Coefficient:
Criteria | Spearman's Rank Correlation Coefficient | Pearson Correlation Coefficient |
---|---|---|
Data Type | Ordinal, non-parametric data | Interval or ratio, parametric data |
Calculation Basis | Based on the ranks or orders of values | Based on actual values |
Sensitivity to Outliers | Less sensitive, robust to outliers | Sensitive, affected by outliers |
Non-linear Relationships | Measures monotonic relationships, not necessarily linear | Measures linear relationships |
Assumptions | Fewer assumptions – doesn't require data to be normally distributed | Assumes linearity and normality in data |
Practical Applicability | Useful for non-linear relationships and non-continuous data | Effective for linear relationships and continuous data |
Interpretation | Focuses on the direction and strength of the monotonic relationship | Emphasizes the direction and strength of linear relationship |
Frequently Asked Questions (FAQs)
It finds applications in psychology, social sciences, market research, medicine, finance, and biological sciences, especially when analyzing ordinal or non-parametric data to understand relationships between variables.
A negative Spearman correlation coefficient close to -1 suggests a solid negative monotonic relationship, indicating that higher ranks in one variable correspond to lower ranks in the other and vice versa.
Yes, Spearman's correlation is generally more robust to outliers due to its focus on the ranks of data rather than the actual values, making it less influenced by extreme values compared to Pearson's correlation.
Recommended Articles
This article has been a guide to what is Spearman Rank Correlation Coefficient. We explain its formula, examples, advantages, and comparison with Pearson Correlation. You may also find some useful articles here -