Spatial Autoregressive Model
Published on :
21 Aug, 2024
Blog Author :
N/A
Edited by :
Shreeya Jain
Reviewed by :
Dheeraj Vaidya
What Is A Spatial Autoregressive Model(SAR)?
The spatial autoregressive model (SAR) is a statistical and econometric model used to analyze and understand spatial data by accounting for spatial dependencies or spatial autocorrelation. The primary purpose of this model is to quantify how the spatial arrangement and proximity of observations affect the variable being studied.
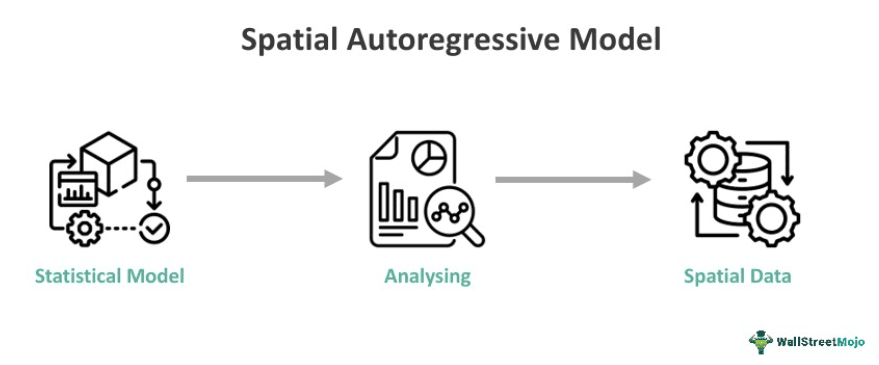
This model can provide a rigorous framework by analyzing spatial data and account for spatial dependencies, providing more accurate, unbiased, and insightful results in a wide range of applications. Researchers commonly use these models in fields like geography, economics, and environmental science to address research questions involving spatial data and dependencies.
Table of contents
- Spatial autoregressive models are used to account for spatial dependencies in data. These are often seen in geography, economics, and environmental science.
- They help in analyzing how the proximity of observations affects the variable being studied, making predictions and understanding spatial relationships more accurate.
- Common types of spatial autoregressive models are Conditional Autoregressive, Simultaneous Autoregressive, and Moving Average Process Models. Each addresses spatial dependencies in different ways and finds applications across various fields.
Spatial Autoregressive Model Explained
Spatial Autoregressive Models (SARs) provide an efficient means to address the common challenge of spatial dependence within geographic research. It introduces a mathematical structure that characterizes the interplay between values in different locations.
One primary rationale for the adoption of this model is to account for spillover effects that transcend location boundaries. In the housing market, neighboring homes' prices influence a home's price, not solely determined by its attributes. At the same time, studying phenomena where the values of the same variable in nearby locations influence the values of a variable in one location.
Therefore, by incorporating spatially lagged variables as predictors, researchers can effectively make use of these effects. These models also help alleviate the risk of omitted variable bias, a scenario where the model omits crucial factors. When dealing with spatial data, disregarding spatial dependence can lead to biased and less efficient estimates.
The use of SARs becomes indispensable in these situations, as they constitute an established statistical framework for modeling relationships between variables when spatial dependencies are evident. By doing so, SARs ensure greater accuracy and reduce bias in the results of geographic and spatial data analyses.
Moreover, when applying a spatial autoregressive structure to a variable, it simplifies the representation of spatial dependencies by introducing an n x n spatial weights matrix denoted as W. This matrix, which can take various forms, constrains how observations relate to each other in the model and aids in estimating covariance among locations.
Thus, a spatial autoregressive model is a statistical tool that helps researchers account for and analyze spatial dependencies in data. Therefore, by providing a more accurate and insightful understanding of how phenomena vary across geographic space.
Types
There are three common spatial autoregressive model types - the Conditional Autoregressive Model (CAR), the Simultaneous Autoregressive Model (SAR), and the Moving Average (MA) Process Model.
- Conditional Autoregressive (CAR) models are constructed based on Markovian principles. Here, the conditional distribution of a component of the random vector is influenced solely by a defined set of neighboring components. In essence, this model is specific instances of Markov random fields. These models have found applications in various scientific disciplines, with examples including image analysis, epidemiology, and agriculture.
- Simultaneous Autoregressive (SAR) models are valuable when dealing with data that have discrete locations or areas of interest. Researchers often set up these models hierarchically, resembling mixed-effects regression models. In this setup, a traditional linear regression controls the first-moment structure (the average behavior), while correlated random effects influence the second-moment structure (variance and correlation).
- Moving-average model (MA model) is a commonly used method in time series analysis to model a single time series variable. It suggests that the current value of the variable depends on a linear combination of its recent past values, along with a random (unpredictable) term.
When we discuss time series modeling, the MA model is one of two fundamental models, the other being the autoregressive (AR) model. Together, they form critical components of more complex models like ARMA (Autoregressive moving-average model) and ARIMA (Autoregressive integrated moving average). These more advanced models account for both autoregressive and moving-average components. However, it provides a more detailed representation of time series data with complex stochastic patterns.
Examples
Let us look at the spatial autoregressive spatial models examples to understand the concept better.
Example #1
Let’s say in a statistical research project, Jane is investigating crime rates across multiple districts within a city. She is interested in comprehending how the crime rate in a specific district is influenced not solely by local factors but also by the crime rates in nearby districts. To address this spatial dependency, Jane employs a spatial autoregressive model.
With this model, Jane delves into the data and estimates the degree to which crime rates in one district are impacted by the crime rates in adjacent districts. Therefore, this enables her to make more precise predictions about future crime rates, taking into account the spatial interactions and relationships between the various districts. Essentially, this model helps her uncover and quantify the spatial patterns and influences in crime rates, providing valuable insights for her statistical analysis.
Example #2
Imagine a study examining the volatility of stock prices in a network of financial markets, such as stock exchanges in different countries.
In this context, this model could help uncover whether fluctuations in stock prices for a particular market are influenced not only by its internal factors but also by the volatility in neighboring markets.
For instance, suppose there is a sudden surge in volatility in one stock exchange. Hence, this model may reveal whether this heightened volatility propagates to nearby exchanges due to spatial dependencies.
Understanding these spatial relationships could be crucial for risk management and investment strategies. Investors and financial analysts could benefit from insights, as in how shocks or fluctuations in one market may have a ripple effect on neighboring markets. Moreover, by considering the spatial interdependencies, the model provides a lens through which to analyze and navigate the complex dynamics of financial markets in an interconnected global economy.
Applications
Some of the common and impactful applications of Spatial Autoregressive (SAR) models:
#1 - Economic Analysis
In economic research, researchers use these models to analyze regional economic disparities. They help economists understand how economic conditions in one geographic area affect those in neighboring areas. This is crucial for regional economic planning, resource allocation, and addressing economic inequalities.
#2 - Real Estate And Housing Markets
These models are applied to the real estate industry to study housing markets. They help analysts assess how property prices influence the prices of properties in one location in nearby areas. Hence, this information is invaluable for real estate investors, developers, and policymakers.
#3 - Criminology
Criminologists use these models to investigate crime patterns and understand the spatial distribution of crime by examining how crime rates in one area are linked to those in neighboring areas. Thus, law enforcement and policymakers can make more informed decisions about crime prevention and resource allocation.
#4 - Healthcare And Epidemiology
In epidemiology, this model is employed to model the spread of diseases. They help researchers understand how diseases move across geographic regions and identify the factors contributing to disease transmission. This is crucial for designing effective disease control and prevention strategies.
#5 - Environmental Science
Environmental scientists use this model to analyze environmental data, such as air or water quality measurements. These models help researchers understand how pollution or contamination spreads across geographic regions. This information is vital for environmental monitoring, risk assessment, and remediation efforts.
Limitations
- These models require the specification of a spatial weights matrix that defines the spatial relationships between observations. Selecting an appropriate weights matrix can be challenging, and the choice of weights can significantly impact model results.
- Spatial autocorrelation in this model can lead to multicollinearity, where predictor variables are highly correlated. Thus, this can make it challenging to identify the individual effects of predictors on the response variable.
- Moreover, this model can become complex when modeling multiple spatial relationships, such as different lag structures or varying weights. Thus, this complexity can make model selection and interpretation more difficult.
- Here, this model can be sensitive to the choice of spatial weights, lag structures, and other modeling decisions. Small changes in these choices can lead to different results.
Frequently Asked Questions (FAQs)
Estimating parameters in this model involves specialized techniques such as maximum likelihood estimation (MLE) or generalized method of moments (GMM). Software packages like GeoDa, R, and Python provide tools for implementing and estimating SAR models.
Yes, it can be extended to higher-order autoregressive models to capture more complex spatial dependencies. Higher-order models consider the influence of locations beyond immediate neighbors.
This model can be implemented using software such as GeoDa, R (using packages like spider), and Python (using libraries like PySAL or sci-kit-spatial). These tools provide functions for model specification, estimation, and interpretation.
These models may be sensitive to changes in spatial scale, and researchers need to consider the appropriate scale for their analysis. Aggregation or disaggregation of spatial units could impact the relationships captured by the model.
Recommended Articles
This article has been a guide to what is Spatial Autoregressive Model. Here, we explain its types, examples, applications, and limitations. You may also find some useful articles here -