The Shapiro-Wilk and Kolmogorov-Smirnov tests are two different metrics used to test whether a sample is normally distributed or not. While both are suitable for small sample sizes, they differentiate from one another in the following ways:
Table Of Contents
What Is The Shapiro-Wilk Test?
The Shapiro-Wilk test is a statistical method used to assess whether a given dataset is normally distributed. It calculates a test statistic that measures how well the ordered sample quantiles align with the quantiles of a standard normal distribution.
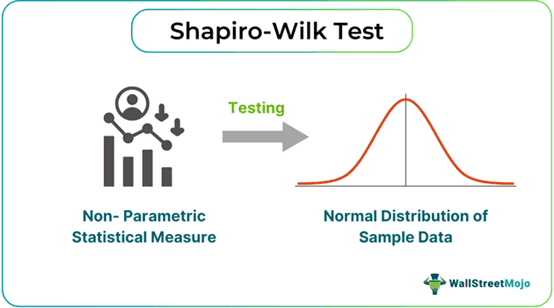
You are free to use this image on your website, templates, etc.. Please provide us with an attribution link.
The method is developed by statisticians Samuel Sanford Shapiro and Martin Bradbury Wilk in 1965, the Shapiro-Wilk test assesses whether a sample, often small, follows a normal distribution. It is especially useful for small sample sizes and is considered one of the most powerful methods for testing normality due to its strong theoretical and foundational support. It is considered one of the most powerful statistical methods for testing normality, with strong theoretical and foundational support.
Key Takeaways
- The Shapiro-Wilk test is a parametric statistical technique used to determine whether sample data is normally distributed.
- Analysts consider a null hypothesis of normal distribution for the sample data. If the p-value is less than or equal to 0.05, the null hypothesis is rejected.
- The method has the highest power for analyzing the normality of sample data, particularly when the sample size is small (i.e., below 50).
- The test statistic is denoted by W and can be mathematically expressed as W = (b / s√n-1)^2.
Shapiro-Wilk Test for Normality Explained
The Shapiro-Wilk test is a statistical tool used to test the hypothesis of normality in sample data; the null hypothesis assumes that the sample comes from a normal distribution. A small W or p-value leads to the rejection of the null hypothesis. The Shapiro–Wilk (S–W) test is a widely recommended formal test for assessing normality. It has demonstrated strong statistical power against various non-normal distributions and complements graphical methods for evaluating normality. The test has been applied in multiple studies, including those involving biomarker data, though some misuse, such as treating it as upper-tailed, has occurred.
The test works by ranking the sample data, computing a weighted sum of these ordered values, and then comparing this sum to the variance of the sample. The resulting statistic, denoted as W, ranges between 0 and 1, with values close to 1 suggesting a good fit to the normal distribution. For practical implementation, the test is often performed using statistical software, as it involves complex calculations and using coefficients specific to the sample size.
To perform the S–W test, assume the sample consists of n independent observations from a normal distribution. The test statistic W is calculated based on ordered sample values and specific constants related to sample size. While W is not normally distributed under the null hypothesis, it can be approximated as normal for sample sizes between 7 and 2,000. Special methods are required for smaller sample sizes, and considerations for ties in data are crucial. The test can be executed using statistical software like StatXact or SAS.
Examples
The Shapiro-Wilk test for normality is widely used in statistical and financial modeling to ensure that the data is normally distributed before proceeding with sample data. Some of its implications can be seen through the following examples:
Example #1
Imagine an economist who wants to explore the relationship between education and poverty in society. The economist collects sample data from the population and uses the Shapiro-Wilk test to check for normality. The test results indicate that the data is normally distributed, i.e., the null hypothesis is not rejected. Therefore, she can proceed with parametric statistical tests that assume normality, such as linear regression, to analyze the relationship further.
Example #2
Suppose a health department wants to analyze the distribution of a health metric, such as blood pressure, across a large population. They collect data from 10,000 individuals and use the Shapiro-Wilk test to assess normality. Suppose the test indicates that the data is not normally distributed. In that case, i.e., the null hypothesis is rejected, the department might opt for non-parametric methods, such as the Kruskal-Wallis H test, to analyze the data, as these methods do not assume normality.
Advantages And Disadvantages
The Shapiro-Wilk test is a critical measure for testing the sample normality since, for performing any statistical test, normal distribution of data is a crucial requirement. However, it has certain limitations. These pros and cons are as follows:
Advantages
- It is highly effective for detecting deviations from normality, especially in small sample sizes.
- Provides reliable results for normality testing in smaller samples.
- Useful as a prerequisite for other parametric statistical tests that assume normality.
- Based on solid statistical theory and concepts.
- Easily implemented through statistical software.
Disadvantages
- The test is complex to compute manually and generally requires statistical software.
- May detect minor deviations from normality in large samples, leading to potential over-rejection of the null hypothesis.
- Does not quantify the degree of deviation from normality.
- While effective for small samples, its performance with very large samples might be less intuitive and can result in over-sensitivity
Shapiro-Wilk Test vs Kolmogorov Smirnov
Basis | Shapiro-Wilk Test | Kolmogorov Smirnov |
---|---|---|
1. Definition | Hypothesis test for normality, assuming normality as the null hypothesis. | Hypothesis test for normality, assuming normality as the null hypothesis. |
2. Power | More powerful for small sample sizes and detecting deviations from normality. | More powerful for small sample sizes and detecting deviations from normality. |
3. Tests | Evaluates the goodness-of-fit of the sample to a normal distribution by comparing observed and expected order statistics. | Evaluates the goodness-of-fit of the sample to a normal distribution by comparing observed and expected order statistics. |
4. Considers Covariances | Considers covariances of order statistics. | Considers covariances of order statistics. |
5. Sample size | Effective for small sample sizes (n < 50). | Effective for small sample sizes (n < 50). |