Sequential Sampling
Table Of Contents
What Is Sequential Sampling?
Sequential sampling refers to a non-probabilistic statistical method that facilitates the testing of subsequent groups of observations until the researcher is sure about the accuracy of the outcome. However, the sample size n and the timeframe for the collection of sample data are not predetermined in such cases.
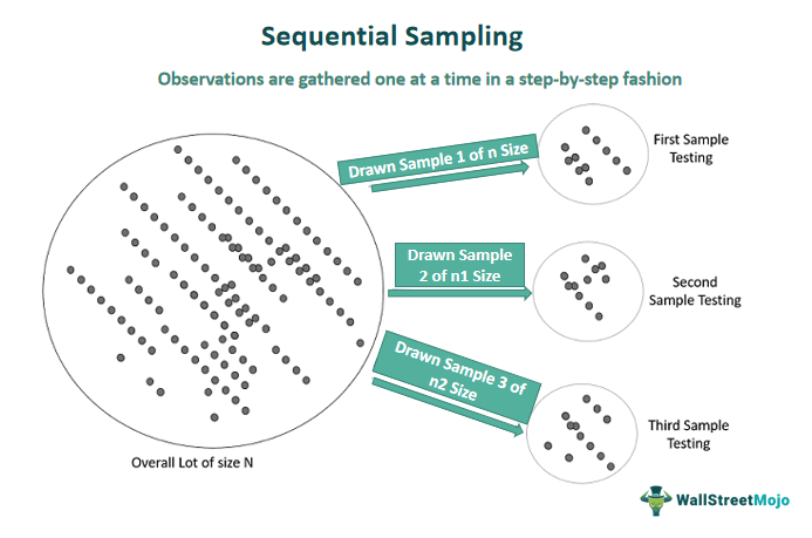
It requires incremental collection of data in a step-by-step manner. Also, the researcher can verify the outcome of the foremost sample testing with the other sets of observations to verify its precision. This approach is commonly employed when acquiring all data simultaneously is impractical or time-consuming, thus enabling continuous analysis and decision-making as new data emerges.
Table of contents
- Sequential sampling is a statistical process of performing simultaneous sample testing one at a time while drawing different sets of observations from a given lot. Notably, the sample size and the timeframe for data gathering need to be the same.
- It is a widely applicable technique in businesses for quality control, environmental monitoring, medical trials, marketing, finance, and simulation or machine learning.
- The hypothesis testing can have three outcomes: the null hypothesis is accepted, the null hypothesis is rejected, or an uncertain result. When the null hypothesis is accepted, the researcher can verify the outcome with multiple other sample sets from the same population.
Sequential Sampling Explained
Sequential sampling is a method that tests sample data progressively for different observation sets in a sequential order without knowing a fixed sample size or period. It, thus, helps in updating decisions or conclusions as additional data becomes available. This non-probabilistic method is widely utilized in decision-making, experimentation, and statistical inference, where the introduction of each new data point contributes to a better understanding or sensible decision-making based on the evolving information.
In this process, the researcher primarily tests a sample with the first group of observations. Then, the outcome of this test can be either to accept the null hypothesis, reject the null hypothesis, or have an uncertain outcome. If the researcher accepts the null hypothesis, then they can continue to ascertain the accuracy of the statistical inference by sample testing the other sets from the same lot. Also, they may take another set of observations if the previous sampling provides an uncertain outcome. Moreover, technology and computers have introduced sequential sampling plans like negative binomial and Poisson distribution to streamline the process.
The sequential sampling plans can be categorized as follows:
- Item-by-item: In this form of sampling, the researcher takes one group of observations at a time from the lot for testing; also, the sample sizes of various sets can vary. Also, it is the most preferred method while employing a sequential process.
- Group: In a group sequential sampling, the researcher can simultaneously perform two or more sample tests using the samples drawn out of the same lot.
Applications
The versatility of sequential sampling makes it a workable method across diverse fields, contributing to the decision-making processes that involve the progressive collection of data. Some of these fields include:
- Quality Control: It is useful in manufacturing to assess product quality by inspecting a product sample progressively until a decision happens about the entire batch.
- Medical Trials: In clinical trials, sequential sampling is suitable for continuously monitoring new treatments' efficacy and safety based on interim analyses.
- Finance: It is applied in financial modeling for risk management and investment decisions, where data is collected over time to make informed choices about portfolio adjustments.
- Environmental Monitoring: This method is used to monitor environmental variables such as air or water quality, enabling the tracking of changes over time and timely decisions on interventions.
- Simulation: The four distinct sequential sampling procedures are employed to generate data from a computer simulation experiment featuring a multi-item inventory model for determining the computer time costs for achieving specific levels of statistical precision.
- Integrated Pest Management: These techniques help gauge the efficiency of pest control and its long-term prevention by testing different sample areas from the total area treated.
- Speech and Signal Processing: In real-time applications like speech recognition or signal processing, sequential sampling helps refine algorithms based on continuous input data.
Examples
Sequential sampling is a suitable method compared to random sampling when there is a limited lot size and decisions are to be made after thorough analysis. Some examples to illustrate the same are as follows:
Example #1
Consider a chocolate factory using sequential sampling for quality control. Rather than inspecting every chocolate bar in a batch simultaneously, the quality control team regularly tests small samples throughout production. Production continues smoothly if the initial samples meet the desired taste and texture. However, if an issue arises, adjustments can be made immediately, preventing the need for large-scale recalls.
Example #2
In a software development project, sequential sampling is appropriate to assess the effectiveness of different coding practices continuously. Instead of waiting until the end of the project to analyze all the code, the development team reviews subsets regularly. If a particular coding approach proves problematic, adjustments can occur promptly, ensuring a more efficient and error-resistant software development process.
Advantages And Disadvantages
Some of the advantages and disadvantages are the following:
Advantages
- Early Insights: The sequential sampling method provides a better identification of the problem than the random sampling method since it doesn’t require additional data for drawing statistical inferences.
- Time Efficiency: It facilitates ongoing data collection and analysis, thus saving time on the comprehensive data gathering before beginning the analysis.
- Cost-effectiveness: This approach proves more economical as resources or data are utilized thoroughly, allowing for decisions based on repetitive testing of the available data.
- Flexibility and Adaptability: It adapts to dynamic situations where conditions or parameters may change during the study. It even incorporates emerging data patterns, facilitating more versatile decision-making.
- Reduces Sample Size: Even when the lot contains a colossal database, this technique aids in testing small sample sets from the data pool.
Disadvantages
- High Risk of Biasness: There is a possibility of a biased outcome. Biased outcomes happen if the initial data is limited and considerably influences the decisions without proper control.
- Statistical Complexity: Sequential analysis methods are often prone to the complex nature of the statistical process and mathematical calculations. Also, excessive sampling may hinder the validity of the outcome.
- Resource Allocation Challenges: Continuous data collection may require constant changes in input variables. Mismanagement of such data can create inefficiencies.
- Limited Generalization: If the sample size is small, the generalization of the result to the broader population will not happen.
- Somewhere Time-consuming: The decisions regarding sample size selection can be extensively more time-consuming than the fixed size sampling method.
- Not Suitable for Large Lot Size: When the population size is massive, the sequential data may not serve the purpose. Since the research may draw different results each time multiple samples are under test from this lot.
Frequently Asked Questions (FAQs)
Sequential sampling is relevant across various fields for dynamic data collection and decision-making. It provides early insights, saves time, and proves cost-effective. Its adaptability to changing conditions makes it a valuable tool. Hence, its application is popular in manufacturing, clinical trials, finance, environmental monitoring, and speech and signal processing.
Sequential sampling of water involves collecting samples over time to monitor changes in water quality. It is crucial for environmental monitoring, allowing researchers to track variations in parameters like contaminants. This method helps in making timely decisions for interventions, ensuring the safety of water sources.
The key distinction lies in their approach to sample collection. Sequential sampling involves testing one sample at a time and continuously updating decisions based on emerging data. In contrast, multiple sampling entails collecting samples from different locations or groups simultaneously. While both aim to provide insights, their timing and data collection strategies differ.
Recommended Articles
This article has been a guide to what is Sequential Sampling. Here, we explain its examples, advantages, disadvantages, and applications. You may also find some useful articles here -