Table Of Contents
What Is Sampling Theory?
Sampling theory refers to the statistical branch dealing with the selection, gathering, and evaluation of data obtained from a sample population. It provides efficient and trusted methods to estimate and draw inferences, plus estimate characteristics of the whole population along with testing hypotheses.
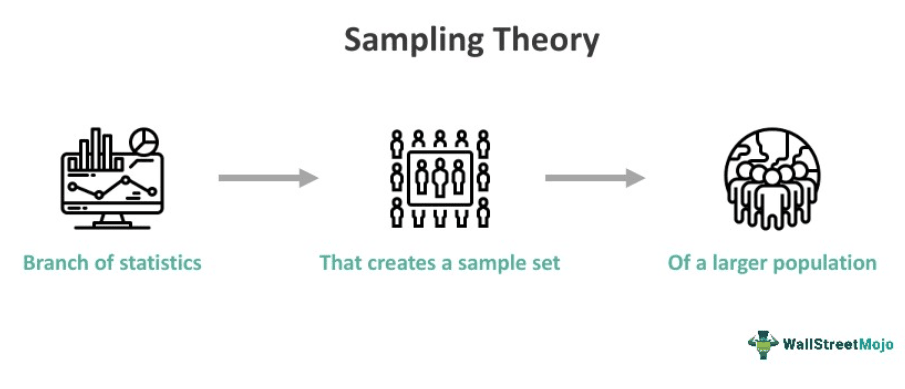
Sampling theory is fundamental in various fields, including market research, political polling, quality control in manufacturing, and scientific research. Moreover, it aids surveys, observational studies, and experiments. Researchers use it for data collection of animals, plants, and other organisms' populations. It finds usage in testing and inspecting processes and products.
Table of contents
- Sampling theory is a branch of statistics that provides a framework for making inferences about a population based on a subset of that population, called a sample.
- Its types include simple random, systematic, stratified, cluster, non-probability, convenience, judgmental, snowball, and quota sampling.
- Moreover, businesses, marketers, financiers, educators, economists, quality control specialists, agriculturists, forest managers, healthcare professionals, environmental researchers, and social scientists all apply it.
- Hence, it is essential for efficient data collection, cost-effectiveness, informed decision-making, population representation, practicality in complex situations, and statistical analysis support.
Sampling Theory in Statistics Explained
Sampling theory in statistics means the practice of choosing a subset entity or individual out of a larger population to collect data to make inferences about the whole population. Moreover, it works by selecting a representative sample of market participants or financial data. The samples could contain investor behavior, stock prices, or bond yields.
Furthermore, researchers employ a random or systematic sampling method to ensure unbiased pictures. They then analyze these samples and draw appropriate conclusions concerning the larger financial landscape. Additionally, sampling theory bias arises when the sample selection is more or less likely to include specific individuals, resulting in inaccurate or skewed results.
Therefore, financial analysts and researchers use it to draw inferences about entire market participants' stock prices or bond yields in a short time and affordable cost. Hence, using sampling, they are able to estimate vital financial elements like market trends, average returns, and volatility with confidence. In the financial world, it has led to the development of different models like the Black-Scholes-Merton model and the Capital Asset Pricing Model (CAPM). These models forecast the return and risk of markets by utilizing statistical sampling techniques.
In finance, professionals utilize it for risk assessment, market research, and portfolio management. It encompasses the sampling theory definition and its practical implementation, ensuring that researchers select representative samples for accurate data collection. Besides, attribute sampling theory is particularly relevant in auditing, quality control, and compliance testing.
In summary, it has advanced financial research, modeling, and decision-making processes by enabling effective data collecting and estimating. Hence, the critical goal of sampling theory in research is to ensure that the sample is representative of the population, allowing researchers to draw accurate and meaningful conclusions.
Types
Sampling theory encompasses various types of sampling methods, each with its own set of principles and procedures. Here are some common types of sampling methods:
- Probability sampling: Every member of the dataset has an equal chance of getting selected for the sample, making it the most reliable sampling type. It can be further subdivided into.
- Simple random sampling: A random number generator conducts the sampling, providing every member with an equal opportunity for selection.
- Systematic sampling: It is applicable to a population organized in any way, like a customer list. Here, every kth member of the list is selected, originating from a random starting point.
- Stratified sampling: It is applicable in a heterogeneous population by dividing it into different groups and choosing a random sample from every group.
- Cluster sampling: It is done for widely scattered populations, and it isn't easy to recognize every member. It divides the population into clusters from which any single cluster is selected randomly.
- Non-probability sampling: Here, every participant of a population does not have an equal chance to get selected in the sample.
- Convenience sampling: It selects easy-to-access members of a population when resources and time are limited.
- Judgmental sampling: Here, researchers select only those samples which they believe represent the entire population.
- Snowball sampling: Researchers begin the sampling from smaller samples and reach out to larger ones through them.
- Quota sampling: In it, first, small quotas are assigned, and then samples are selected till the quota of each group gets fulfilled.
Applications
Many fields widely use it to benefit from it. Hence, listed below are some of its applications:
- Business and marketing: Used in surveys of market research, evaluating customer satisfaction, and marketing campaigns.
- Finance: It gets applied in assessing transactions and financial audits.
- Education: It allows evaluation of student performance.
- Economics: It aids in data collection on economic indicators, consumer spending, and employment rates.
- Quality Control: In manufacturing and production, it helps in quality control.
- Forest Management: Habit data, species composition, and timber volume deploy it.
- Healthcare: It assists in monitoring disease outbreaks, conducting clinical trials, and assessing patient health.
- Environmental Studies: Environmental pollution levels, monitoring air and water quality, and biodiversity apply.
- Social Sciences: Demographic studies, surveys, and opinion polls use it.
- Epidemiology: Here, it helps in studying the prevalence and distribution of diseases in populations—random sampling of individuals to estimate disease rates and identify risk factors.
Examples
Let us look at a few examples to understand about the topic.
Example #1
Imagine, Market Vision Research, a prominent marketing research firm in the US, aims to understand consumer preferences for a new product. Instead of surveying every potential customer, which could be time-consuming and costly, the researchers decided to apply sampling theory. Hence, they start by defining the target population, which consists of all potential customers. When using random sampling, ensure each individual in the population has an equal chance of inclusion by selecting a subset of customers.
Therefore, this ensures that the sample is representative of the broader consumer base. The researchers may use various methods, such as phone interviews, online surveys, or in-person questionnaires, to collect data from the sampled individuals. Moreover, one can extrapolate the insights gained from this representative sample to make informed decisions about the entire population's preferences for the new product.
Thus, the application of this theory in this scenario enables the company to gather valuable information efficiently while minimizing costs and resources.
Example #2
In order to determine if data complies with these requirements, Langer Research Associates, the primary polling provider to ABC News, advises the news division on the standards for disclosure, validity, dependability, and unbiased content in survey research.
On disclosure, the company adds that they also want a clear methodology statement, the entire questionnaire, and all marginal data in their submissions, in addition to the names of the study sponsor and the fieldwork provider.
In terms of methodology, the firm requires a sampling theory, namely a probability-based sample with extensive coverage of a reliable sampling frame. Internet opt-in, e-mail, "blast fax," call-in, street intercept, and non-probability mail-in samples are only a few examples of non-probability, self-selected, or so-called "convenience."
Importance
Owing to its wide applications, as discussed in the previous section, it has many importance. Hence, let's list some of its major importance below:
- Efficient Data Collection: The use of smaller subgroups of populations simplifies data collecting and helps researchers save time and money.
- Cost-Effective Research: It is economical since examining a whole population may be quite expensive, particularly in fields like market research and healthcare.
- Informed Decision-Making: It offers trustworthy insights into industries like market research and healthcare, plus quality control, enabling well-informed decision-making.
- Population Representation: Ensuring that insights are typical of the total population is achieved by pulling data from samples, thereby increasing the validity of findings.
- Practicality in Complex Situations: It is crucial in situations when it is impracticable to examine the entire population, for example, when evaluating enormous forests or complex manufacturing processes.
- Statistical Analysis Support: It is an indispensable instrument for research, analysis, and effective resource management since it facilitates statistical analysis and helps with parameter estimates.
Frequently Asked Questions (FAQs)
According to sampling theory, researchers can generalize findings to what?
How is sampling theory linked to probability?
What is sampling theory in machine learning?
What is sampling theory in research methodology?
Key Takeaways
- Sampling theory is a branch of statistics that provides a framework for making inferences about a population based on a subset of that population, called a sample.
- Its types include simple random, systematic, stratified, cluster, non-probability, convenience, judgmental, snowball, and quota sampling.
- Moreover, businesses, marketers, financiers, educators, economists, quality control specialists, agriculturists, forest managers, healthcare professionals, environmental researchers, and social scientists all apply it.
- Hence, it is essential for efficient data collection, cost-effectiveness, informed decision-making, population representation, practicality in complex situations, and statistical analysis support.