Table Of Contents
Sampling Error Definition
Sampling error is the deviation between a sample (the mean or proportion) and the corresponding population parameter. Reducing it aims to improve statistical estimates' accuracy and reliability and minimize the risk of making incorrect inferences about the population.
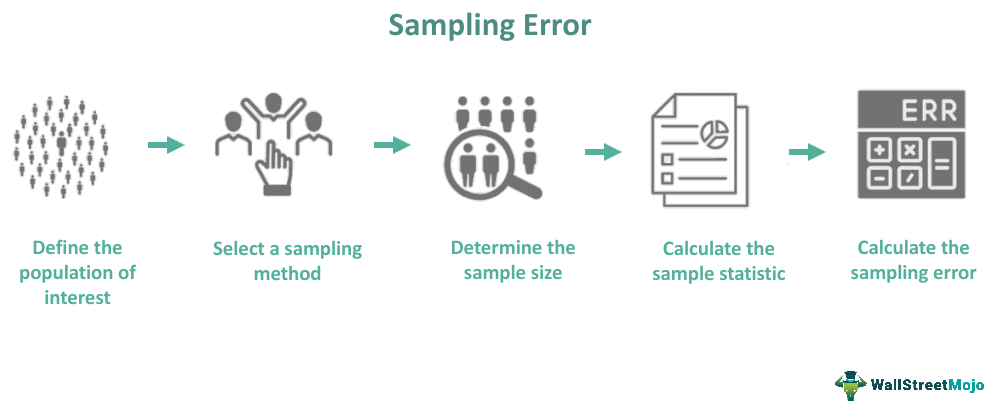
To achieve these aims, researchers typically use random sampling techniques to ensure that their sample is representative of the population. They may also use larger sample sizes to reduce the variability and increase the precision of their estimates. In addition, reducing such errors can improve the validity and generalizability of research findings.
Key Takeaways
- Sampling error is the variation between a sample and the corresponding population parameter. This is due to the natural variation that arises when a random sample is selected from a population.
- It uses mathematical formulas and depends on factors like the sample size, population standard deviation, and confidence level.
- It can lead to imprecise or biased estimates of population parameters. This can have significant implications for research findings, policy decisions, and business decisions.
Sampling Error Explained
Sampling error is a critical concept in statistics that has important implications for the validity and reliability of research findings. The idea arises because it is often impractical or impossible to directly study an entire population of interest. Instead, researchers use samples to conclude the population.
Its origin roots in probability theory. This tells us that the results of a sample are subject to random variation due to chance. This variation can be quantified and characterized mathematically, forming the basis for statistical inference. However, errors can also arise from non-random sources like selection bias, measurement error, or non-response bias. This leads to systematic differences between the sample and the population.
The significance of such error lies in its impact on the accuracy and reliability of statistical estimates. Significant errors can result in biased or imprecise estimates not representative of the population of interest. As a result, errors can lead to incorrect inferences about the people and undermine the validity of research findings. Moreover, suppose the sample does not represent the people. In that case, this can lead to biased estimates that over- or under-estimate critical parameters of interest, which can have significant practical and policy implications.
Reducing error is a critical objective in many research studies. Researchers aim to use random sampling techniques to ensure that their sample is representative of the population. In addition, they may use larger sample sizes to reduce the variability and increase the precision of their estimates. By reducing errors, researchers can improve their calculations' accuracy and reliability and increase their findings' validity and generalizability.
Causes
Some of the most common causes are:
- Random Variation: It can occur due to chance. Because a sample is only a subset of the population, it will naturally have some degree of variation due to the randomness of the selection process. This random variation can cause the sample statistics to differ from the population parameters.
- Sampling Bias: Bias can occur if the sample does not represent the population. This bias can occur due to non-response, self-selection, or convenience sampling.
- Measurement Error: It can also arise from measurement error, which occurs when the measurements or observations are not accurate or precise. Measurement error can be due to the instrument that measures a variable, data collection methods, or the observer making the measurements.
- Non-response Bias: Non-response bias can occur when individuals selected for the sample choose not to participate in the study. If the non-response is related to the variables of interest, this can lead to biased estimates of population parameters.
- Sampling Frame Errors: Sampling frame errors can occur when the list or frame used to identify the population is incomplete or inaccurate.
Types
There are two main types:
- Random Error: It is due to the natural variation that occurs when a random sample is selected from a population. It results from chance factors and is an inherent part of the sampling process. The magnitude of unexpected errors can be reduced by increasing the sample size. As the sample size increases, the variability in the sample means decreases, and the sample mean becomes a better estimate of the population tell.
- Systematic Error: It is due to factors that systematically bias the sample in a particular direction. It is not due to chance factors; it can occur when the sampling method or sampling frame is flawed. For example, if a researcher only samples from one geographic region, this can lead to a biased sample if the population of interest is spread across multiple areas. Systematic error can also occur due to measurement error or non-response bias.
Other types of such errors that can occur include:
- Coverage Error: Coverage error occurs when the sampling frame used to define the population needs to be completed or accurate, resulting in certain groups being underrepresented or excluded from the sample.
- Non-response Error: Non-response error occurs when individuals selected for the sample choose not to participate in the study, leading to a biased sample. This can occur due to factors such as unwillingness to participate.
- Measurement Error: Measurement error occurs when the instrument used to measure a variable is not accurate or precise, leading to biased estimates of population parameters. Measurement error can occur due to poorly calibrated instruments, observer bias, or sampling method bias.
Formula
It can be estimated using the following formula:
Sampling error = (Z-score) x (standard deviation of the population / square root of the sample size)
Where:
The Z-score is the number of standard deviations from a normal distribution's mean corresponding to the desired confidence level (1.96 for a 95% confidence level).
The standard deviation of the population is the standard deviation of the variable of interest in the population.
The sample size's square root equals the sample's total number of observations.
This formula calculates the maximum expected difference between the sample statistic and the proper population parameter due to chance variation. The size of the error depends on the confidence level chosen (i.e., the probability of being correct), the standard deviation of the population, and the sample size. The error decreases as the sample size increases, while the Z-score and standard deviation's effect remains constant.
Examples
Let us understand it better with the help of examples:
Example #1
Suppose a researcher wants to estimate the average height of adult males in a city. They randomly sample 100 males from a population of 100,000 and calculate the mean sample height as 5 feet 10 inches. The researcher then uses this sample mean to estimate the population means size, assuming that the model represents the people.
However, the error associated with this estimate is likely to be large, given the small sample size relative to the population size. Furthermore, if the standard deviation of heights in the population is high, the error could be quite large and lead to incorrect inferences about the population's mean size.
Example #2
One example of a recent sampling error in the news is the COVID-19 vaccine efficacy estimates. Vaccine efficacy estimates are calculated by comparing the number of COVID-19 cases in the vaccinated group to those in the placebo group. However, these estimates are subject to such error due to the small sample sizes and the random variation in the number of issues between the two groups.
For example, the efficacy estimate for the Pfizer vaccine was initially reported to be 95%, but this estimate had a confidence interval that ranged from 90% to 98%. This means that the true vaccine efficacy may be lower or higher than the reported estimate due to chance variation in the sample.
Sampling Error vs Non-Sampling Error
The main differences between them are:
- Definition: Sampling error is the error that occurs due to the natural variation that arises between a random sample and a population. Non-sampling error, on the other hand, is an error that occurs due to factors other than the sampling process, such as errors in data collection, processing, or analysis.
- Origin: Sampling error arises from the sampling process, whereas non-sampling error can arise from any stage of the research process, from study design to data analysis.
- Type of Error: Sampling error is a random error due to chance factors and can be quantified mathematically. Non-sampling error, on the other hand, can be accidental or systematic and is often more challenging to quantify and address.
- Magnitude: Sampling error tends to decrease as the sample size increases, while non-sampling error can be reduced through careful study design, data cleaning, and statistical analysis.
- Impact on Results: Sampling error can lead to biased or imprecise estimates of population parameters, while non-sampling error can lead to biased estimates, incorrect conclusions, or invalid generalizations.
Sampling error vs Sampling Bias
Sampling error and bias are two familiar sources affecting statistical samples' accuracy and representativeness. Here are the main differences between them:
- Definition: Sampling error is the difference between a sample statistic and the corresponding population parameter. On the other hand, sampling bias occurs when the sample is not representative of the population due to systematic factors such as non-random sampling or non-response.
- Origin: The former arises from the inherent randomness of the sampling process while sampling bias arises from systematic factors that distort the sample selection process.
- Type of Error: The former error is random due to chance factors and can be quantified mathematically. Sampling bias, on the other hand, is a type of systematic error, which means that it is due to frequent factors and can lead to biased estimates that are not representative of the population.
- Magnitude: While the former tends to decrease as the sample size increases, sampling bias may be a free sample size and may persist even with extensive samples.
- Impact on Results: The former can lead to imprecise estimates of population parameters. In contrast, sampling bias can lead to estimates systematically different from the population parameters, resulting in incorrect conclusions and invalid generalizations.