Table Of Contents
Robust Statistics Definition
Robust Statistics refers to the statistical metrics, methods, or tools that are not influenced by minor, negligible, or insignificant deviations while interpreting data for the whole population from idealized assumptions, providing efficient or strong performance. These deviations can include outliers or non-normality in the given data.
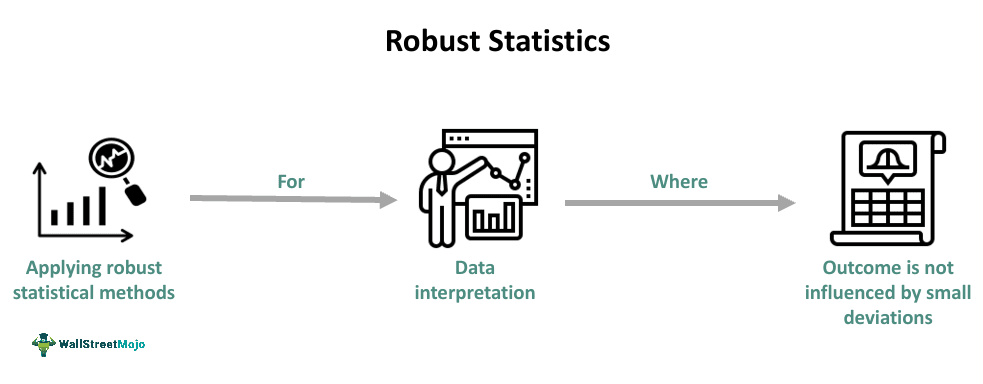
The most common methods or measures that ensure accurate outcomes are Winsorized Mean, Trimmed Mean, Median, Rank-based Tests, Interquartile Range (IQR), and Median Absolute Deviation (MAD). While dealing with real-world data analysis, statistical robustness is a crucial parameter in diverse fields, including finance, economics, bioscience, and environmental science.
Key Takeaways
- Robust statistics comprise statistical measures, which are known to provide reliable or accurate results for the whole population when the extracted data is skewed.
- Some prominent robust measures include median, Median Absolute Deviation (MAD), Interquartile Range (IQR), robust regression, and robust ANOVA.
- These methods are instrumental in drawing accurate statistical inferences, such as confidence interval estimation and hypothesis testing in real-world situations where data can be noisy or contain anomalies.
- In financial modeling, robust techniques can produce more reliable parameter estimates, ensuring that the model accurately represents the underlying patterns in the data.
Robust Statistics Explained
Robust statistics is a branch that focuses on methods insensitive to small outliers often seen in traditional statistical techniques. It seeks to derive accurate and reliable results even when the assumptions of classical statistics are not met. Most common robust statistics theories and methods include the median and trimmed means, which are less influenced by extreme values than the mean, and robust regression techniques like Huber regression and M-estimation.
The concept of robust statistics emerged in the mid-20th century. A prominent statistician, John Tukey, made significant contributions during the 1960s by introducing concepts like the Median Absolute Deviation (MAD) and advocating for the use of robust techniques across varied fields. In the subsequent decades, robust estimation methods, such as M-estimators, gained recognition.
In 1964, Peter Huber's work on breakdown points and robust regression techniques furthered the development of this field. Frank Hampel made an addition to the discipline in 1981. During the 1980s-1990s, robust methods found applications across various disciplines. It continues to evolve, leveraging modern computational tools to address complex data challenges.
Robust statistics Huber methods are widely used in finance, engineering, and environmental science, where data often deviates from idealized assumptions. Such metrics play a crucial role in data analysis, ensuring reliability even when the data used deviates from the ideal assumptions of traditional statistical methods.
Moreover, real-world data often contains outliers, errors, or non-normal distributions, making it essential to employ robust statistical techniques. Extreme values have less influence on these methods in the data, ensuring that these outliers do not disproportionately impact the results. It applies to a broader range of data types than traditional statistical methods.
Assumptions
Statistical tests are crucial in behavioral, social, and health science research to test hypotheses. They depend on assumptions like independence of the observations, equal variances, and normality.
- The independence of the observations means that observations should be independent of each other, i.e., every observation should be independent of each other.
- Equal variances mean that the variance of the population from which each observation is taken or drawn should be the same.
- Normality states that the distribution of the population from which each observation is drawn should be normally distributed.
The assumption of normal distribution is the most often used model formalization. It has been a part of statistics for two centuries and serves as the foundation for multivariate regression and analysis of variance. The central limit theorem is used to justify this assumption, assuming it holds exactly.
Examples
Robust statistics theory and methods are less popular than classical statistics. However, these methods provide valuable insight in some instances, such as in descriptive statistics. Some examples are discussed below.
Example #1
Let us take the example of the median. Take the following data set:
45, 47, 48, 54, 57, 59, 65, 66, 70
The numbers are arranged in an ascending order. The median is the 5th value in the data series, i.e., 57. Thus, using the robust method median, the central value acquired is 57. If we change the 9th value from 70 to 100, the median will remain the same. Hence, this anomaly will not affect the outcome much, unlike the mean.
Example #2
Assume a quality control manager is in charge of the production of batteries at 10,000 hours. The manufacturer claims these batteries have an average lifespan of 10,000 hours. To validate this assertion, the manager decides to conduct a hypothesis test using a sample of batteries from the production line.
For this, the manager will be required to formulate a hypothesis, choose a statistical test, collect the relevant data, and compute the figures based on the method chosen to arrive at a decision.
Solution:
Null Hypothesis (H0): The average lifespan of the batteries is 10,000 hours.
Alternative Hypothesis (H1): The average lifespan of the batteries is not 10000 hours.
A random sample of 100 batteries is selected, and their lifespans are recorded. For instance, the sample mean lifespan is 9900 hours, and the sample standard deviation is 60 hours.
Applying the t-statistic test to the problem:
t = ×√n
t = ×√100 = -16.67
Since the degree of freedom is 99, the p-value is 0, and it does not exceed 0.05, the manager can reject the null hypothesis. This implies there is sufficient evidence to conclude that the average lifespan of the batteries is different from the 10,000 hours claimed by the manufacturer.
Hence, this example demonstrates the application of t-procedures, specifically t-tests, in making statistically significant conclusions about population parameters based on sample data.
Applications
Robust statistics theory and methods are techniques used to make statistical inferences under conditions where the classical assumptions of many statistical methods may be violated. Here are some of its common uses in the real world:
- Outlier Detection: Robust statistics help identify outliers in data by providing resistant measures of central tendency and dispersion, such as the median and interquartile range, which are less influenced by extreme values.
- Hypothesis Testing: These statistical tests, such as the Mann-Whitney U test and Wilcoxon signed-rank test, are alternatives to classical parametric tests like the t-test and ANOVA when data does not meet the assumptions of normality.
- Time Series Analysis: Such methods are applied to time series data to account for outliers, trends, and seasonality, providing more reliable forecasts and analysis.
- Biosciences and Environmental Studies: These methods are crucial in ecology and genetics, where data can be influenced by non-normally distributed variables.
- Finance and Economics: Robust statistics are used in financial modeling and econometrics to handle extreme market events and deviations that could distort economic analysis and predictions.
Advantages And Disadvantages
The selection between robust and traditional statistics depends on specific data characteristics and research objectives. Robust methods hold relevance for non-normally distributed data or datasets containing outliers. Let us understand its pros and cons.
Advantages
- Robust statistics resist outliers, making them suitable for data sets with extreme values. They work without significantly skewing results.
- Unlike traditional methods, these methods do not rely on the assumption of normality, making them applicable to non-normally distributed data.
- They can be applied to various data types, including ordinal or interval data, enhancing their utility across different research scenarios.
- Robust methods often yield more accurate parameter estimates and statistics, especially in the presence of outliers or non-normal distributions.
- They enable fair comparisons between groups or conditions by reducing the impact of outliers or skewed data, leading to more meaningful and reliable results.
- These tools provide valid statistical inferences, such as hypothesis testing and confidence interval estimation, even when the data do not meet the assumptions of classical statistical methods.
Disadvantages
- Robust statistical methods may not provide accurate results for all normal distributions; in some cases, they may draw less precise estimates than traditional methods.
- Some robust techniques are more complex to compute and interpret, posing challenges for individuals lacking a solid statistical background.
- These measures might not always be the best choice since ignoring outliers that carry meaningful information can result in the loss of crucial insights.
- Specific robust techniques demand larger sample sizes to perform effectively. Hence, they are unsuitable for limited or small data.