Residual Analysis
Last Updated :
-
Blog Author :
Edited by :
Reviewed by :
Table Of Contents
What Is Residual Analysis?
Residual analysis or salvage value refers to a statistical technique for assessing the performance of a linear regression model through residual examination. It serves to debug and evaluate the model's assumption and goodness of fit, plus identify outlier's data points for increased efficiency.
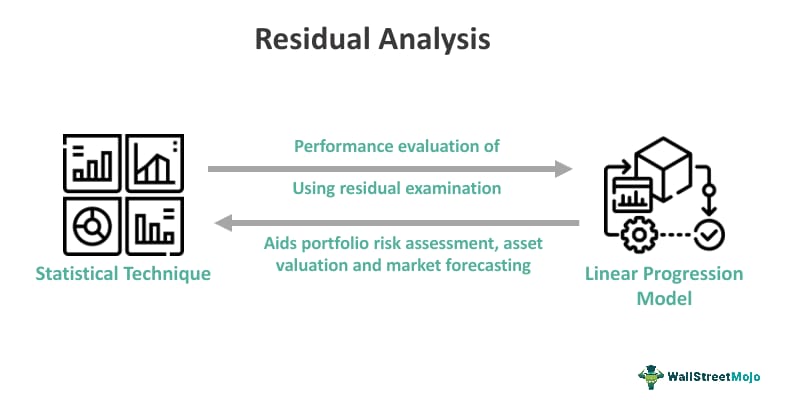
It examines the residual plot graph, providing the metric of the model fit. Moreover, it measures error quantity related to outcomes forecast. Investors use it to examine various investment strategies' portfolio investment performance and efficacy. Financial professionals identify possible risks to assess risk model accuracy. Analyzing these residuals helps evaluate the chosen model's appropriateness and identify areas for improvement.
Table of contents
- Residual analysis, also known as salvage value assessment, is a statistical method used to evaluate a linear regression model's performance by analyzing residuals.
- It helps identify and rectify model issues, assess assumptions, and detect outliers for improved efficiency.
- Moreover, it involves understanding residuals, collecting data, constructing a regression model, calculating residuals, visualizing residuals, analyzing residuals, assessing model assumptions, refining the model if necessary, and interpreting results.
- This analysis aims to assess the goodness of fit of a statistical model to the observed data.
Residual Analysis Explained
Residual analysis is a statistical technique used to assess the quality of a model by examining the differences between observed values and the values predicted by the model. Hence, it is used to authenticate the regression model. By evaluating residuals, one can know whether the assumptions needed for precise forecasts are satisfied by the error term within the model. Furthermore, residual analysis in regression is a critical step in assessing the quality and appropriateness of a regression model.
Moreover, stock market analysts started using it in the financial world. Overvalued and undervalued stocks were analyzed by them. Nowadays, market professionals deploy it in different applications like market forecasting, risk management, fraud detection, portfolio optimization, and asset pricing. However, residual analysis applications span scientific research, industry, and decision-making processes where statistical modeling is employed.
It works as shown below:
1 – The residuals of each data set must be calculated by decreasing the predicted value from the actual value.
2 – After that, a scatter plot is created for independent variables versus residuals.
3 – Then, one must examine the plot, which means a good plot if it shows randomly scattered residuals around the zero line. Other than that, any pattern means unfit for data.
4 – Finally, use the successful model of fit of residual analysis used for testing assumptions of the model like linearity, normality, and homoscedasticity.
Thus, in the financial world, it helps in pricing assets, detecting fraud, forecasting market trends, optimizing investment portfolios, plus developing and validating risk management. Moreover, finance professionals can refine their models & understand relationships within financial data.
Besides, it's crucial to note that this analysis is an iterative process. After adjusting the model, it's essential to repeat the analysis to ensure that the residual analysis assumptions are met.
How To Do?
In the finance sector, residual analysis can be done in the following manner:
- Understanding Residuals - First, familiarize oneself with the residuals concept and the difference between predicted and observed values in the regression model.
- Data Collection - After that, collect appropriate data like asset returns, interest rates, & stock prices relevant to one's analysis.
- Constructing the Regression Model - Use the financial data to construct a regression model. Hence, this will use the relationship between the variables to predict outcomes accurately.
- Calculating Residuals - Next, use the observed value and subtract the predicted value from it inside the regression model.
- Visualizing Residuals - Then, plot a residual plot graph visualizing the patterns and distribution of the residuals. Therefore, this will help identify deviations away from the presumptions of the regression model.
- Analyzing Residuals - After that, carefully analyze the residuals by examining the residual plot. As a result, one could validate the regression model and segregate non-linear relations between variables. Hence, one can quickly know any issues in the model's assumptions.
- Assessing Model Assumptions - Next, check if the error term of the regression model successfully complies with assumptions of normality of residuals, independence, linearity, and homoscedasticity. Thus, if the residual analysis assumptions are fully complied with, then the model is correct; otherwise, it is not.
- Model Refinement - In case of non-compliance of error terms with assumptions, the mode needs refining.
- Interpreting Results - Finally, the results obtained from the regression model are carefully observed and interpreted. It will help increase one's interrelationship of financial data. Such an understanding will further lead to more precise forecasting and sound financial decision-making.
Thus, this analysis in regression is a crucial step in statistical modeling with tools like residual analysis calculator & chi-square residual analysis simplifies the assessment of model fit and error quantification.
Examples
Let us use a few examples to understand the topic.
Example #1
Let's consider an analyst aimed at predicting stock returns based on various financial indicators. After fitting the regression model, the analyst performs a thorough residual analysis to validate the model assumptions. Hence, the residuals, representing the differences between predicted and actual stock returns, are first examined through scatter plots against predicted returns and key financial indicators such as earnings per share and market volatility. Therefore, the residuals and predicted returns plot shows a seemingly random distribution, suggesting that the linearity assumption is met.
Furthermore, the standard Q-Q plot of residuals indicates deviations from a normal distribution, signaling a need for caution. In-depth statistical tests assess outliers and influential data points that could significantly impact the model's predictions. The analyst may need to explore alternative functional forms, incorporate additional variables, or address outliers to enhance the model's robustness and ability to capture complex dynamics within financial markets. Thus, this hypothetical example underscores the importance of residual analysis in financial modeling, where the stakes are high, and accurate predictions are crucial for effective investment decision-making.
Example #2
Suppose Noah recently bought a studio apartment in New Jersey for $300,000 with a 20-year lease. An in-depth analysis indicates that the property's value is expected to be 55% of its original price at the end of the lease. This estimate is based on market trends and comparisons with similar local properties. To calculate the final residual value, we consider a $10,000 disposal fee and follow these steps:
1. Salvage Value = $300,000 x 0.55 = $165,000
2. Cost of Disposal: $10,000
3. Residual Value = $165,000 - $10,000 = $155,000
After 20 years, the property is projected to have a residual value of $155,000. Hence, this figure guides property owners in making informed decisions about rental rates and whether to retain or sell their properties for the best financial outcome.
Importance
The ability to evaluate the suitability of linear regression models and improve comprehension of the underpinning connections in financial data are two key benefits of residual analysis in the financial sector. Hence, it is necessary to know the
importance of the analysis using the table below:
Aspect | Details |
---|---|
Investment Analysis | It plays a crucial role in property valuation and real estate investment decision-making. |
Lease Determination | These help to prepare lease agreements for vehicles and machinery while calculating lease payments. |
Loan Calculation | In loan assessment, it is pivotal as it impacts loan terms and interest rates. |
Model Validation | Validating the accuracy of regression models ensures trustworthy financial predictions. |
Assumption Verification | Normality and linearity model assumptions are verified by it, which are critical for legitimate financial decisions. |
Risk Assessment | This analysis identifies potential model flaws and outliers, which are essential for managing market anomalies and financial risks. |
Decision-Making | Moreover, it helps finance professionals make well-judged decisions concerning portfolio management, investments, and risks. |
Continuous Improvement | Hence, it facilitates ongoing model refinement, assuring the relevancy and accuracy of regression models in financial landscapes. |
Frequently Asked Questions (FAQs)
The four primary residual analysis techniques are:
- Residual plots
- Statistical tests
- Leverage and influence analysis
- Lastly, residual patterns and trend analysis
To perform residual analysis in Excel:
1. Input data.
2. Create a scatter plot.
3. Add a trendline.
4. Compute residuals (actual - predicted).
5. Build a residual histogram.
6. Examine the histogram for normality and randomness.
7. Conclude model adequacy based on the histogram's distribution.
Residual analysis within regression serves as a statistical tool to evaluate the soundness of a regression model. Residuals represent the disparities between the regression line and actual data points. Scrutinizing the residuals' distribution enables the assessment of the model's suitability for the dataset.
Residual analysis provides valuable insights into the regression model, encompassing:
1. Suitability of the model for the data.
2. Presence of bias in the model.
3. Risk of overfitting the data by the model.
4. Identification of potential outliers within the dataset.
Recommended Articles
This article has been a guide to what is Residual Analysis. Here, we explain it in detail with its examples, how to do it, and importance. You may also find some useful articles here -