Table Of Contents
What Is Reliability Analysis?
Reliability analysis examines the credibility and consistency of a measurement scale, assessing its ability to produce consistent and relevant results when the measurement process is repeated multiple times. Researchers aim for high reliability through this test because it ensures that the outcomes can be trusted.
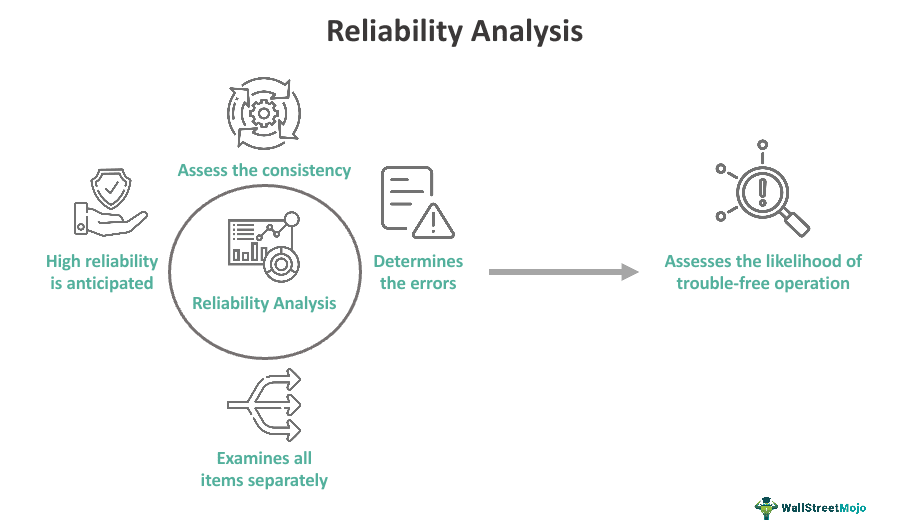
The analysis not only offers multiple measures to assess reliability but also allows analysts to study the individual components of the scale and the relationships between them. With the help of reliability analysis, a scale can be tested for consistent or inconsistent results, which is crucial before applying the scale in any statistical model.
Key Takeaways
- Reliability analysis assesses a scale's consistency by examining results when measurements are repeated multiple times.
- Researchers typically desire high reliability to ensure the scale is trustworthy and produces credible outcomes.
- The four main techniques in reliability analysis methods include test-retest, parallel forms, split-half, and inter-rater reliability methods.
- If reliability analysis fails to demonstrate the scale's consistency, researchers may need to consider alternative approaches.
Reliability Analysis Explained
Reliability analysis is the process of evaluating the consistency of a scale to determine the trustworthiness of its outcomes. This scale can refer to various entities such as models, machines, equipment, surveys, or frameworks. The analysis assesses to what extent the results can be relied upon, ensuring that subsequent calculations based on the underlying model remain relevant, accurate, and credible. In essence, it involves examining the scale's reliability before incorporating it into further analysis.
The definition of reliability analysis emphasizes that, regardless of what the scale is intended to measure, be it productivity, knowledge, weight, efficiency, or distance, it is essential to first test the scale for consistency. Suppose a scale exhibits varying levels of reliability. In that case, it will adversely affect the overall study or research by providing unreliable results, undermining the research objective and wasting time, energy, and resources.
Reliability analysis can be implemented using different programming languages and software tools. For instance, in SPSS (Statistical Package For Social Sciences), reliability analysis aids in assessing the extent to which the scale values consistently represent the intended attribute and outcome. It is important to consider several assumptions during the test, as each model may require a different approach, and certain aspects should not be overlooked or excluded.
Methods
There are four methods of reliability analysis:
- Split-half reliability: This method primarily focuses on errors resulting from poorly constructing the mechanism, scale, or system. It involves splitting the model into two parts and evaluating them separately. The correlation of the outcomes is then calculated. A higher correlation indicates greater consistency.
- Inter-rater reliability: This method assesses each component of the model individually. It judges each aspect separately and calculates the overall percentage agreement between them. A higher percentage signifies greater reliability.
- Test-retest reliability: This method helps identify errors in the scale due to administration issues. It assumes the model is correct, but errors may occur due to handling and administrative problems. The scale is tested with a variable group, withdrawn for a specific time frame, and retested. The correlation between both tests is compared, with a correlation of at least 0.80 or higher considered a sign of good reliability.
- Parallel forms reliability: This method explores external factors that may influence the reliability of the model or scale. It also assumes the model is correct but that external factors cause contingencies. In this technique, a variable group is examined using one version of the test, and then the same group is tested using an alternative version. The obtained correlations are then compared.
Examples
Below are two examples of reliability analysis:
Example #1
Consider a financial analyst assessing the consistency of quarterly earnings reports from a company. The analyst selects a sample of consecutive quarterly reports and applies reliability analysis to ensure the reported financial figures are stable over time. This examination is crucial for investors and stakeholders who rely on the company's financial data to make informed decisions. A high-reliability outcome would instill confidence in the accuracy and consistency of the financial reporting, enhancing the trustworthiness of the company's performance indicators.
Example #2
Imagine an economist studying the reliability of inflation data provided by a government agency. The economist selects a representative sample of monthly inflation reports and employs reliability analysis to assess the consistency of the reported inflation rates. A high-reliability outcome would indicate that the inflation figures are consistently measured and reported over time, providing policymakers and businesses with reliable information for economic decision-making. Conversely, a low-reliability outcome may prompt a closer examination of the data collection process and potential external factors influencing the reported inflation rates.
Advantages And Disadvantages
Some of the important advantages and disadvantages are the following:
Advantages
- Helps identify problems or components within the system that can be excluded.
- Aims to confirm the reliability of the system through repeated measurements.
- Allows for deducing internal consistency and relationships between variables.
- Provides a backup plan for researchers in case of failure, enabling them to consider a different scale or model for analysis.
- Ensures that the model is well-suited and can produce consistent and accurate results.
Disadvantages
- Reliability analysis relies on several assumptions, which may not always hold in all cases.
- It may not be suitable for systems with varying failure rates over time.
- When working with exponential distribution, external effects are not taken into account.
- There is no single universal method for reliability analysis. Each scale or system may require a different technique.
Reliability Analysis vs Factor Analysis
Reliability Analysis
- Tests the consistency of a measuring scale
- Assesses how well a group of variables go together
- Helps choose a model or scale for future calculations
- Aims for high reliability
Factor Analysis
- Describes the variance in observed correlated factors
- Defines the correlation between observed variables and groups them
- Reduces a large number of variables to a few factors
- Identifies the maximum common variance