Table Of Contents
What Is Regression Discontinuity Design (RDD)?
Regression Discontinuity Design (RDD) is a quasi-experimental research approach used to evaluate the causal effect of an intervention or treatment. It is applicable when a cutoff point determines the assignment to the intervention. This design enables researchers to conclude an intervention's impact where randomization is not feasible.
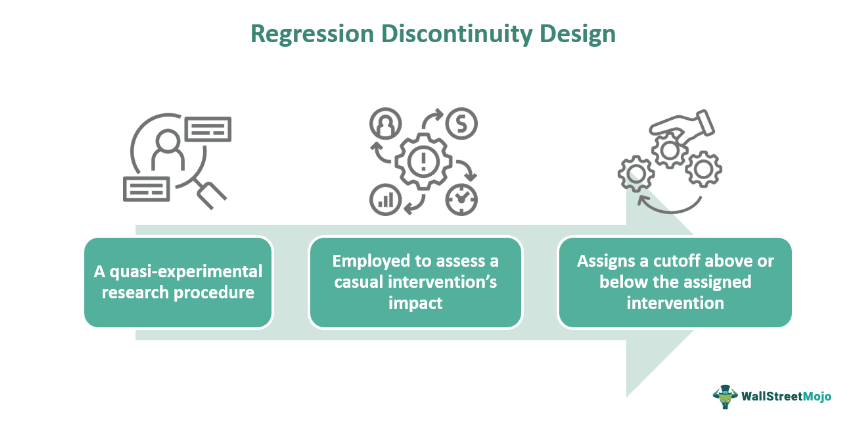
RDD runs on the principle that entities just above or below a predetermined threshold receive different treatment conditions. It compares the outcomes of individuals or groups that are immediately above or below the threshold. The method provides valuable insights into policy evaluations, social programs, and circumstances where controlled experiments are non-viable.
Table of contents
- Regression Discontinuity Design is a quasi-experimental research strategy that facilitates the estimation of causal effects by incorporating naturally occurring cutoffs or thresholds.
- This strategy is beneficial when random assignment to treatment is not viable. It is helpful in a wide range of fields, including policy assessments, finance, and social studies, where ethical constraints restrict the use of randomized control trials.
- However, this method requires a significant amount of data on each side of the threshold for accurate calculations. A scarcity of data close to the cutoff might compromise the preciseness of the treatment effect estimates and increase statistical noise in the results.
Key Takeaways
- Regression Discontinuity Design is a quasi-experimental research strategy that facilitates the estimation of causal effects by incorporating naturally occurring cutoffs or thresholds.
- This strategy is beneficial when random assignment to treatment is not viable. It is helpful in a wide range of fields, including policy assessments, finance, and social studies, where ethical constraints restrict the use of randomized control trials.
- A scarcity of data close to the cutoff might compromise the preciseness of the treatment effect estimates and increase statistical noise in the results.
Regression Discontinuity Design Explained
Regression Discontinuity Design is a quasi-experimental research method that aids in the calculation of causal effects by using naturally occurring cutoffs or thresholds. This design is valuable when random assignment to treatment is not suitable. It compares groups, individuals, or entities just above and just below a specific threshold. Additionally, it assumes that their characteristics are similar, except for their varying closeness to the cutoff.
The basic notion behind this design is that at the threshold, there is a discontinuous change, and the groups on either side are comparable. This approach acts as a credible way to assess a randomized control trial. It is guided by the idea that entities on one side of the threshold closely resemble those on the other side. RDD provides valuable insights into causal relationships in settings where randomization is not possible. Moreover, it is beneficial in several domains like policy evaluations, economics, and social sciences, where ethical limitations prevent the use of traditional randomized control trials.
Assumptions
The regression discontinuity design assumptions include the following:
- Continuity Assumption: This design assumes that the assignment variable is continuously distributed and changes smoothly around the cutoff. It implies that the individuals close to the cutoff are similar in all aspects except for their proximity to the threshold.
- Treatment Assignment: The assignment of the treatment is determined by the threshold. This assumption implies that there is a discontinuity in the probability of receiving treatment precisely at the cutoff.
- No Manipulation Around the Cutoff: The method assumes that entities cannot manipulate their assignment status around the cutoff to receive or avoid the treatment.
- Adequately Large Sample Near the Cutoff: An adequate number of observations on either side of the threshold is required to achieve precise estimates. This assumption is essential to reduce the effects of random noise and variations in the data.
Examples
Let us study the following examples to understand this design:
Example #1
Suppose a university decided to offer scholarships as part of a financial aid program for college students who had GPAs above 4. Researchers could evaluate this GPA requirement's impact on academic achievement using RDD. They were able to determine the effect of the scholarship on academic accomplishment by comparing students who were slightly above and below the 4 GPA criteria, assuming similar qualities apart from academic achievement. When students with 3.9 and 4.1 GPAs showed disparities in their subsequent academic performance, it revealed information on how financial aid influenced academic outcomes.
Example #2
The COVID-19 pandemic had a significant impact on food security worldwide. A study was conducted to examine how the pandemic affected urban households in Bangkok, Thailand, and Manila, the Philippines, with respect to how much a healthy diet cost and how affordable it was. The national statistics agencies offered official food prices, household income, and food expenditure data.
The impact of COVID-19 on food prices was estimated using RDD. The results of this study indicated that the pandemic had a more significant impact on the affordability of healthy diets due to a decrease in poor people's earnings instead of through price hikes.
Advantages And Disadvantages
Some advantages of regression discontinuity design are:
- It provides a robust method to establish causal relationships in situations where random assignment is impractical or unethical. It allows researchers to estimate randomized control trial conditions, which makes it valuable in policy evaluations and real-life situations where controlled experiments are challenging.
- The method benefits from naturally occurring cutoffs and is cost-effective and practical. It enables efficient use of available data. Additionally, it saves time and resources.
- RDD aims to ensure that observed differences in outcomes are likely due to the treatment or intervention by comparing units just above and just below the threshold. This minimizes selection bias.
- This design is versatile and applicable across various domains. It serves as a robust instrument for evaluating the effects of policies and programs in practical settings where conducting controlled experiments is challenging.
The disadvantages of regression discontinuity design are:
- RDD relies on stringent assumptions. Violating these assumptions compromises the validity of the estimated treatment effects and results in biased conclusions.
- The selection of the threshold can impact the estimated treatment effect. Additionally, manipulating the assignment variable near the cutoff by entities might distort the findings.
- Findings from this design might not always apply to different settings. The dependence on specific thresholds restricts the broad application of the findings.
- It demands a large sample on either side of the threshold for accurate estimations. If data near the cutoff is scanty, it may compromise the accuracy of treatment effect calculations and increase statistical noise in the results.
Regression Discontinuity Design vs Difference-In-Differences vs Interrupted Time Series
The differences between the three are as follows:
Regression Discontinuity Design
- RDD examines the causal effects of an intervention by utilizing a predetermined cutoff or threshold.
- It compares units just above and just below the threshold.
- This method is beneficial when random assignment is not feasible as it provides robust estimates at the cutoff.
Difference-In-Differences
- Difference-in-differences compares changes in outcomes between a treatment and control group before and after an intervention.
- It accounts for time-invariant unobserved diversity but assumes parallel trends.
- Its effectiveness is affected when trends vary without a suitable control group.
Interrupted Time Series
- Interrupted Time Series assesses the effects of an intervention by analyzing changes in outcomes before and after the intervention.
- It captures immediate and long-term effects.
- However, it may be influenced by external events.