Table Of Contents
What Is Random Sampling?
In statistics and research methodology, random sampling selects a subset of items from a larger population. The primary aim of it is to obtain a representative sample that accurately reflects the characteristics of the entire population.
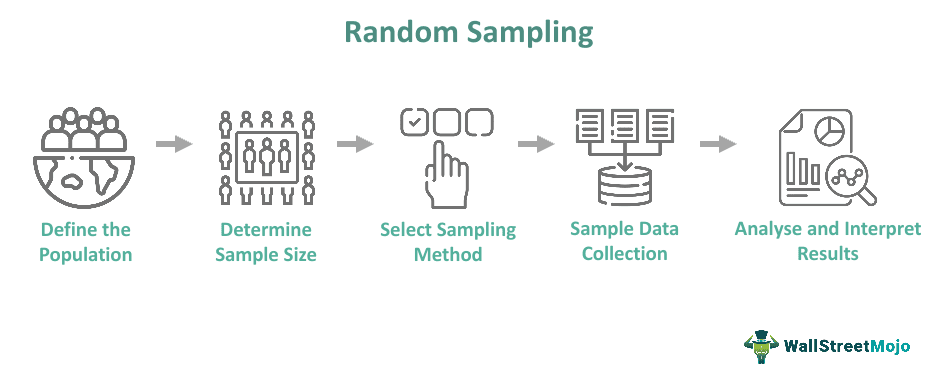
It enables researchers to make valid inferences about the larger population based on the characteristics observed within the sample. In addition, by selecting a diverse and representative sample, researchers can generalize the findings from the model to the people with a reasonable level of confidence.
Key Takeaways
- Random sampling provides a solid basis for statistical analysis, including hypothesis testing, estimation, and constructing confidence intervals.
- It can be more efficient and cost-effective than other sampling methods. In addition, it requires less time, effort, and resources than exhaustive sampling.
- It is fundamental in research design, forming the basis for many research studies, surveys, and experiments. It provides a systematic and unbiased approach to selecting participants or items, ensuring the validity and reliability of the research.
Random Sampling Explained
Random sampling is a fundamental statistical and research methodology concept from probability theory. Its relevance and critical importance stem from its ability to ensure representativeness and reduce bias in sampling processes.
The concept represents an early development of statistics in the 18th and 19th centuries, with notable contributions from mathematicians such as Pierre-Simon Laplace and William Gosset (Student). However, the systematic use of such sampling gained prominence during the 20th century with advancements in statistical theory and the widespread adoption of probability sampling techniques.
Its relevance lies in its ability to address one of the central challenges in research: generalizability. Collecting data from a population is often impractical or impossible when conducting research. Instead, researchers rely on a sample—a smaller population subset—to make inferences and draw conclusions about the whole population. It helps ensure that the model represents the people, enabling researchers to generalize their findings more confidently.
By using randomization methods to select individuals/items, random sampling helps eliminate selection bias. This reduces the risk of distorting the results and allows for more accurate estimates and statistical inference.
Types
Some commonly used types of random sampling:
- Simple Random Sampling: In Simple Random Sampling, each member has an equal chance of selection. For instance, if you have a population of 100 individuals and want a sample size of 20, each individual has a 1 in 5 (or 20%) chance of selection.
- Systematic Random Sampling: Here, elements from the population are selected based on a predetermined pattern or interval. For instance, if you have a population of 500 individuals and want a sample size of 50, you could randomly select a starting point between 1 and 10 and then select every 10th individual after that.
- Stratified Random Sampling: It involves dividing the population into distinct subgroups or strata based on specific characteristics relevant to the research. Then, a sample is within each stratum to select individuals proportionally or disproportionately, depending on the research objectives.
- Cluster Random Sampling involves dividing the population into clusters or groups based on a specific criterion, such as geographical location or organizational units. Then, a random sample of clusters is chosen, and all individuals within the selected groups are included in the model. It is often more practical and cost-effective when accessing the entire population is difficult or impractical.
- Multi-stage Random Sampling: It involves combining different sampling techniques. It is often used when the population is large and complex. The sampling process consists in selecting clusters in the first stage, subclusters within the chosen groups in the second stage, and so on until the final sample is obtained.
Examples
Let us understand it through the following examples.
Example #1
Suppose a research study aims to determine the favorite ice cream flavor among children in a city. The researchers randomly selected 100 children from the city's population and asked them about their preferred ice cream flavor. Using simple random sampling, each child in the population has an equal chance of selection. The findings from this sample can then be generalized to the larger population of children in the city.
Example #2
During the ongoing pandemic, random sampling was used to estimate the prevalence of COVID-19 antibodies in the population, providing insights into the spread of the virus and the level of immunity in different regions.
Health authorities randomly selected individuals from the population and conducted antibody tests to determine if they had previously been infected with the virus. By using such sampling, the aim was to obtain a representative sample that reflected the overall population. The findings from these random samples helped estimate the percentage of individuals infected and developed antibodies, which in turn informed public health policies and strategies.
Advantages And Disadvantages
Let us observe some of the advantages and disadvantages of random sampling:
#1 - Advantages Of Random Sampling
- Representative Sample: It ensures that each member of the population has an equal chance of selection for the sample. This helps to create a representative sample that accurately reflects the characteristics of the entire population. In addition, it increases the likelihood of generalizing the findings from the example to the larger population.
- Reduction of Bias: Eliminating selection bias minimizes the risk of systematically over- or under-representing specific population segments. It reduces the influence of the researcher's personal biases and preferences, leading to more objective and unbiased results.
- Statistical Inference: It provides a solid foundation for statistical analysis. It allows for applying various statistical techniques, such as hypothesis testing, estimation, and constructing confidence intervals. In addition, the properties and characteristics observed within the random sample can be used to make valid inferences about the population.
#2 - Disadvantages Of Random Sampling
- Sampling Error: It does not guarantee that the sample will perfectly match the population's characteristics. Due to chance variations, there will always be some sampling error or discrepancy between the model and the people. The larger the sample size, the smaller the sampling error is likely to be.
- Potential Lack of Precision: In some cases, it may result in imprecise estimates or findings, mainly if the sample size is small. The sample's variability may limit the estimates' accuracy, leading to wider confidence intervals and reduced precision.
- Practical Limitations: It may face functional limitations in certain situations. For example, obtaining a complete sampling frame (list of all individuals or items in the population) may take time and effort. In such cases, it becomes challenging to ensure genuinely random selection.
Difference Between Random And Non-Random Sampling
Here's a comparison of random sampling and non-random sampling.
#1 - Convenience
Random sampling does not consider convenience as a factor in the selection process. Therefore, it may require more effort and resources than non-random sampling methods. In contrast, non-random sampling, such as convenience or haphazard sampling, prioritizes convenience and accessibility. Participants or items are selected based on availability or ease of access. As a result, it is often quicker and more convenient than random sampling.
#2 - Biases
Random sampling aims to minimize bias by providing an unbiased population representation. Each member has an equal chance of selection, reducing the risk of systematic over- or under-representation. It helps mitigate selection bias and provides a fair basis for generalizing findings to the population. At the same time, non-random sampling methods may introduce bias because participants or items are not selected randomly. Convenience sampling, for example, may overrepresent easily accessible individuals or entities, potentially leading to biased results.
#3 - Generalizability
While random sampling provides a higher degree of generalizability, selecting a representative sample increases the likelihood of generalizing findings to the larger population. It allows for valid statistical inference and enables researchers to make reliable conclusions about the people. Non-random sampling methods, on the other side, particularly convenience sampling or purposive sampling, may have limited generalizability. The sample may only partially represent the entire population, making it difficult to draw broad conclusions beyond the selected participants or items.
#4 - Cost
Random sampling may require more resources, time, and effort than non-random sampling methods. It often necessitates a complete sampling frame and a larger sample size to ensure representativeness. As a result, the costs associated with data collection, analysis, and logistics may be higher. Whereas non-random sampling methods, such as convenience or purposive, can be more cost-effective. They often require fewer resources and less time compared to random sampling. In addition, researchers can focus on readily available participants or specific subgroups of interest, reducing costs.
Random sampling vs Haphazard Sampling vs Purposive Sampling
Here is a comparison of random sampling, haphazard sampling, and purposive sampling based on various aspects:
#1 - Definition
Random sampling involves selecting individuals or items from a population using a random process, where each member of the population has an equal chance of selection. Haphazard selection, or convenience sampling, involves selecting individuals or items based on convenience or availability without a systematic randomization process. At the same time, Purposive sampling involves selecting individuals or entities based on specific criteria or purposes relevant to the research objectives.
#2 - Aims
Random sampling aims to obtain a representative sample that accurately reflects the characteristics of the entire population. It facilitates generalizability and statistical inference.
Haphazard sampling aims for convenience and practicality by selecting readily available participants or items. It is often used in exploratory studies or when time and resources are limited. At the same time, Purposive sampling aims to select participants or items with specific characteristics or fit particular criteria relevant to the research objectives. It allows for targeted analysis of a specific subgroup.
#3 - Used In
Random sampling is widely used in various research studies, surveys, experiments, and statistical analyses where the goal is to obtain a representative sample from a larger population. Haphazard sampling is commonly used in preliminary or exploratory research, pilot studies, or situations where convenience and accessibility are prioritized over representativeness. At the same time, Purposive sampling is employed in qualitative research, case studies, or research focusing on specific subgroups or characteristics of interest.
#4 - Requirements
Random sampling requires a complete sampling frame, a list, or a representation of the entire population. It often requires a larger sample size to ensure representativeness and minimize sampling error. At the same time, haphazard sampling does not have strict requirements or specific procedures. It relies on the researcher's convenience and accessibility to select participants or items. Whereas, purposive sampling requires careful consideration of the research objectives and the criteria for selecting participants or items. It relies on the researcher's judgment and expertise to identify the relevant characteristics.