Table Of Contents
What Is The Random Effects Model?
Random Effects Model (REM) refers to a type of hierarchical linear model accounting for variation between groups or clusters unexplainable by the observed variables. It estimates the effect on a dependent variable by an independent variable while considering the variability inside a cluster.
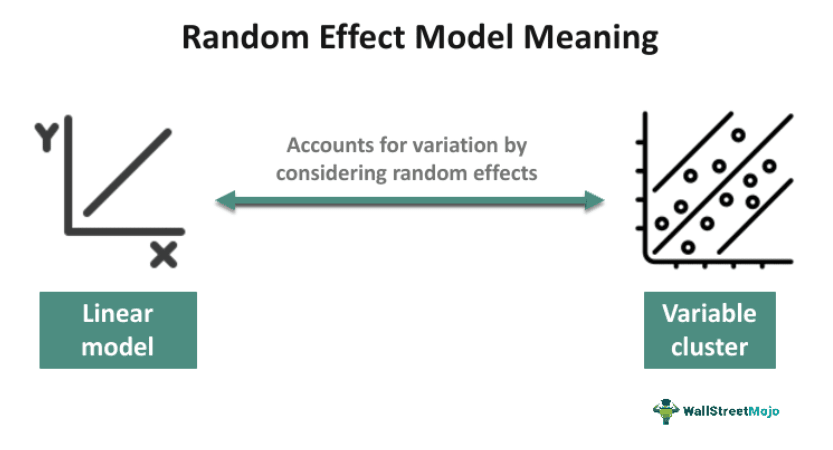
Psychological research commonly uses it to account for individual differences within study participants. It helps in determining the effectiveness of treatments or interventions. The random effects model is a powerful tool for analyzing data having hierarchical or nested structures. It is widely used in multiple fields like economics, medicine, and education.
Key Takeaways
- The random effects model is a hierarchical linear model that accounts for unexplained variation between groups or clusters, estimates the effect of an independent variable on a dependent variable, and considers the variability within each cluster.
- It uses many assumptions to work correctly, like random sampling, independence of observations, homoscedasticity, normality of residuals, linearity, and hierarchical structure.
- It finds application in estimating drug impact, educational program impact, marketing impact, accounting for variability, group-based experiments, etc.
- It has uncorrelated individual-specific effects and independent variables, while in the fixed effects model, they are correlated.
Random Effects Model Explained
The random effects model is a statistical technique utilized in social sciences and econometrics to evaluate panel data involving observations on manifold entities over time. It considers both – between and in-group variations by assuming that individual effects are uncorrelated with explanatory variables while being randomly distributed. It has usages in longitudinal data analysis and evaluation of multilevel data. For complete knowledge of its working, one must know the fixed effects model, too.
For its working, it assumes that unobserved heterogeneity remains randomly spread across groups, clusters or individuals. This means that observed variables have no relation with the unobserved heterogeneity. It also assumes that residuals are identically distributed and are independent. The random effects model starts by treating the individual-specific effects as random variables. Here, individual-specific effects follow a particular normal distribution presumably. Such random effects successfully capture the unobserved heterogeneity throughout individuals.
One cannot explain them using observed explanatory variables. Therefore, including these random effects, the model accounts for the interrelationship between observations amongst every entity while permitting variation across entities. As a result, it has certain associated implications too. First, it offers more efficient determination as compared to other models when dealing with truly random individual-specific effects.
It enables researchers to examine between and in group variations. Thus, it provides insights into the manner in which individual characteristics affect the outcome variable. In this way, outcomes of the random effects model help in generalizing the population as a whole. Moreover, it has effects on the financial world like financial data analysis and using it to estimate an average return on investment of a new financial instrument. It also helps examine the relationship between trading volume and stock returns, the impact of government policy, plus the efficacy of new risk management strategies on the stock.
Assumptions
It has wide applications in many fields but requires many assumptions to work correctly. The assumptions are as follows:
- Random sampling: One has to randomly select the groups or clusters or individuals in the sample out of the selected population.
- Independence of observations: Observations on one group must be free of dependence on another group.
- Homoscedasticity: One must fix the variance related to the outcome variable across all levels of the predictor variables.
- Normality of residuals: One should normally distribute the differences in the values of the observed and the forecasted.
- Hierarchical Structure: It assumes data to have a hierarchical structure where various populations give different results while their population's differences contribute to overall variation.
- Linearity: Another assumption is that there is a linear relationship between dependent and independent variables.
Examples
Let us use a few examples to understand the topic.
Examples #1
The paper 'Random-effects model for meta-analysis of clinical trials: An update,' published in 2006, proposed new methods for estimating the treatment effect from multiple clinical trials. The random-effects model is a statistical method that combines the results of multiple clinical trials to estimate the overall treatment effect. The model takes into account the different results from each trial and provides a more accurate estimate of the overall effect than any individual trial. The authors of the paper propose two new two-step methods for estimating the inter-study variance, which is a measure of the difference in results between trials.
The new methods are more accurate than the older one-step method. The authors also emphasize the importance of using meta-analysis carefully to avoid misleading inferences about treatment effects. They recommend that researchers carefully consider the methods they use and the statistical inferences they make when combining studies with diverse characteristics. The paper is a valuable resource for researchers conducting meta-analyses in clinical trials.
It provides new methods for estimating the treatment effect and highlights the importance of using meta-analysis carefully.
Examples #2
Suppose a researcher named Noah investigates how a cognitive training program affects elderly persons' memory. He collects information from two groups: those who take part in the program (X) and those who do not. His objective is to ascertain the average memory performance effect of the program while accounting for memory's intrinsic variances. For his analysis, Noah uses a random effects model.
This model accounts for unobservable variations between the two groups to assess the program's average memory impact. The average memory gain for all older persons participating in the program—accounting for different improvement probabilities—is present in the results. When studying longitudinal data, random effects models help psychologists uncover underlying inequalities among people.
Applications
One can estimate the model utilizing different statistical software like stata and R. Hence, they can apply it to various fields and objectives like:
- Analyzing Drug Impact: It can help in calculating the average effect of a fresh drug on human blood pressure.
- Assessing Educational Programs: One can use this model to examine the impact of a new educational program on student performance.
- Measuring Marketing Impact: It is used in evaluating the effect on sales by using a predefined marketing campaign.
- Accounting for Variability: In survey-based research, it accounts for individual differences.
- Conducting Group-Based Experiments: Experiments consisting of different groups or conditions have their data examined using it.
- Performing Longitudinal Data Analysis: It plays a vital role in longitudinal data, which involves examining repeated metrics on the same individuals over time.
- Explaining ANCOVA Findings: The dependence of findings in Analysis of Covariance (ANCOVA) is explained by the REM.
- Modeling Unaccounted Variance: The variance in results that the predictor variables' fixed effects cannot account for is modeled using the REM.
- Considering Cluster-Level Factors: The variance in results that the cluster-level factors can't fully address is taken into consideration by the REM.
Besides these, in the realm of statistical analysis, the random effects model, particularly in the context of meta-analysis or random effects model meta-analysis, has garnered significant attention. Researchers often turn to the random effects model in R and Stata to handle complex data structures, accommodating sources of heterogeneity. Moreover, the Bayesian random effects model is gaining prominence as a powerful tool for estimating uncertainty and capturing the intricate dynamics of data.
Random Effects Model vs Fixed Effects Model
Random effects model
- There is no correlation between individual-specific effects and independent variables.
- Constant variance in individual-specific effects.
- Determines average effects throughout all entities.
- Uses the maximum likelihood estimation (MLE).
- Has less efficacy owing to entity-specific effects pooling.
- Presupposes homogeneity of independent variables with regards to individual-specific effects.
- May experience endogeneity bias in the event that this presumption is broken.
- Assumes that entity-specific effects are randomly distributed
- Permits heterogeneity throughout entities.
- Has the capability to estimate cross-sectional effects
Fixed effects model
- There is a correlation between individual-specific effects and independent variables.
- There is a variance in individual-specific effects.
- Estimates entity-specific effects only.
- Deploys the within-group estimation such as least squares dummy variable.
- Has more efficacy owing to consideration of entity-specific effects.
- Allows endogeneity of independent variables with regard to individual-specific effects.
- Provide reliable estimations even when endogeneity is present.
- Considers homogeneity through approximating entity-specific intercepts.
- Does not permit heterogeneity throughout entities.
- Has no capability to estimate cross-sectional effects