Propensity Score Matching
Published on :
21 Aug, 2024
Blog Author :
N/A
Edited by :
Ashish Kumar Srivastav
Reviewed by :
Dheeraj Vaidya
What Is Propensity Score Matching (PSM)?
Propensity score matching (PSM) is a statistical technique used in observational studies to reduce bias by mimicking a randomized experiment when randomization is not feasible. It aims to balance the observed covariates between treatment and control groups, making the comparison more reliable.
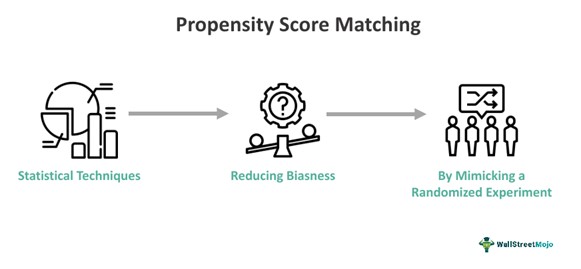
It can help in assessing the impact of specific financial interventions or policies by comparing groups with and without the intervention. For instance, it can be used to evaluate the effect of a specific investment strategy on risk and return by matching similar portfolios or companies. It can aid in comparing individuals or entities with similar characteristics to predict creditworthiness based on past data and behaviors.
Table of Contents
- Propensity score matching aims to reduce bias in observational studies by creating balanced comparison groups, mimicking the randomized assignment of treatments. It balances observed covariates between treated and untreated groups, making them more comparable.
- Propensity scores represent the likelihood of an individual receiving a treatment based on observed covariates. These scores are used to match treated and untreated individuals with similar propensities, facilitating a more accurate comparison.
- It helps to imitate the random assignment of treatments in situations where conducting actual randomized controlled trials is not feasible or ethical. It allows researchers to draw more reliable causal inferences from non-experimental data.
Propensity Score Matching Explained
Propensity score matching is a statistical method used to balance the characteristics of participants in observational studies, minimizing the effects of confounding variables. It calculates the likelihood (propensity score) of an individual receiving a particular treatment or intervention based on their observed characteristics. This score matches individuals with similar propensities.
Originating in the field of econometrics and biostatistics, PSM was popularized by Paul Rosenbaum and Donald Rubin in the 1980s. They introduced the concept as a response to the challenge of assessing causal effects in observational studies. Here, random assignment was not feasible or ethical. The methodology gained traction in various disciplines, including economics, public health, and social sciences.
The technique's core principle involves estimating the probability of receiving a treatment. It creates comparable groups and reduces the impact of selection bias. PSM facilitates a more controlled and accurate assessment of the treatment's actual effect. It resembles a randomized experiment in non-experimental data, thus enhancing the reliability of causal inferences drawn from observational studies.
Steps
Propensity score matching involves several key steps to create balanced treatment and control groups for observational studies:
- Define the Research Question: Begin by formulating a straightforward research question and identifying the treatment or intervention under study. Determine the variables and the outcomes of interest.
- Estimate Propensity Scores: Use statistical methods, such as logistic regression. This helps to estimate the likelihood of receiving the treatment based on observed covariates. The propensity score is a single value representing the probability of treatment assignment for each individual in the sample.
- Matching Individuals: Match treated and untreated individuals with similar or identical propensity scores. Various matching techniques, like nearest neighbor matching or caliper matching, pair participants from the treatment and control groups.
- Assess Balance: Assess the balance of observed covariates between the treatment and control groups to ensure similarities in characteristics. Statistical tests or graphical representations evaluate the success of the matching process.
- Estimate Treatment Effects: Analyze the outcome of interest by comparing the treated and control groups post-matching. This allows for a more accurate estimation of the treatment effect while minimizing bias due to confounding variables.
- Sensitivity Analysis: Conduct sensitivity analysis to test the robustness of results by varying matching techniques or criteria. It ensures the reliability and validity of the findings.
Examples
Let us understand it better through the following examples.
Example #1
Suppose an education research organization aims to evaluate the impact of a new online learning program on student performance. Using propensity score matching, they collect data on students' demographics, previous academic performance, and participation in the program. By estimating propensity scores for each student to join the online program, they match students who have similar scores but differ in program participation. This matching creates a balanced group of students who either participated or did not participate in the online program.
Subsequently, they compare the academic performance (such as exam scores or grades) between the two groups. Through propensity score matching, they find that the online program group shows a statistically significant improvement in performance compared to the non-participant group. This suggests a positive impact of the online learning program on student academic outcomes.
Example #2
In a recent study of 2023 investigating the correlation between household wealth gaps and mental health in China, research from the 2012–2018 China Family Panel Survey has uncovered significant findings. Conducted by employing the two-way fixed effects model and accounting for endogeneity, the study used two-stage least squares and propensity score matching (PSM) to examine the impact of wealth inequality on mental health.
The results revealed a negative effect of household wealth gaps on individuals’ mental health, supported by robustness tests. Heterogeneity analysis indicated that this impact was more pronounced among middle-aged and elderly individuals, those with lower education levels, and rural residents.
PSM played a crucial role in reducing bias, facilitating a more accurate evaluation of the relationship between wealth inequality and mental health. Mechanism analysis also suggests that household wealth gaps influence mental health by affecting an individual's health insurance investment and neighborhood relations. Importantly, these effects were observed not only in the short term but also in the medium to long term.
Benefits
Propensity score matching offers several critical benefits in observational studies and research:
- Reduction of Bias: It helps mitigate selection bias by balancing observed covariates between treated and control groups, making the groups more comparable. This enhances the accuracy of the comparison, leading to more reliable and less biased estimates of treatment effects.
- Mimics Randomized Experiments: In situations where randomization is impractical or unethical, PSM imitates the randomized experiment by creating groups that resemble those resulting from random assignment. This allows researchers to draw causal inferences similar to those from experimental studies.
- Utilizes Existing Data: It can work with existing observational data, making it a cost-effective and efficient approach. It doesn't require additional data collection and can utilize available datasets to derive meaningful insights.
- Flexibility in Study Design: It allows for the analysis of large observational datasets and provides flexibility in studying various treatments, interventions, or policy changes without the constraints of randomization, thereby expanding the scope of research possibilities.
- Enhanced Validity of Findings: By reducing the impact of confounding variables, it enhances the validity of findings, allowing researchers to estimate the actual effects of treatments or interventions more accurately.
Limitations
While propensity score matching is a powerful tool for addressing bias in observational studies, it has several limitations that researchers should consider:
- Assumptions and Model Specification: It assumes that all relevant variables affecting treatment assignment and outcomes are included in the model. The propensity score model needs to be revised to avoid biased estimates.
- Difficulty in Finding Matches: Depending on the quality and quantity of available data, finding suitable matches for participants with similar propensity scores might take a lot of work. This could result in unmatched individuals or a limited pool of matches, reducing the study's precision and generalizability.
- Inherent Selection Bias: While it reduces observable differences between treatment and control groups, it doesn’t account for unobserved or unmeasured variables that might influence both treatment assignment and outcomes. This residual bias could affect the reliability of the estimated treatment effects.
- Sensitivity to Choices in Matching Techniques: Different matching methods (e.g., nearest neighbor, caliper, kernel) might yield varying results. The choice of matching algorithm or criteria can influence the outcomes, leading to potential inconsistencies.
- Loss of Sample Size: The matching process can reduce the sample size, limiting statistical power and potentially affecting the study's ability to detect significant treatment effects accurately.
Propensity Score Matching vs Coarsened Exact Matching
Following is a brief description of the differences between Propensity Score Matching and Coarsened Exact Matching:
Aspect | Propensity Score Matching (PSM) | Coarsened Exact Matching (CEM) |
Matching Technique | Matches individuals based on their estimated propensity scores, aiming to balance observed covariates between treatment and control groups. | Matches individuals based on a coarsened version of observed variables, seeking exact matches on the grouped values of variables. |
Balancing Covariates | Balances observed covariates between groups by approximating conditional independence, reducing selection bias. | Creates exact matches on selected categorical variables or grouped continuous variables, ensuring balance without requiring estimation of propensity scores. |
Complexity | It involves less complexity in terms of modeling, as it focuses on creating exact matches based on grouped or coarsened variables, simplifying the matching process. | Involves less complexity in terms of modeling, as it focuses on creating exact matches based on grouped or coarsened variables, simplifying the matching process. |
Handling Continuous Data | Requires estimation of propensity scores through modeling, involving the selection of variables and model specification. It can involve more complex statistical procedures. | Categorizes continuous variables to create exact matches based on predefined intervals or ranges, thus simplifying the handling of continuous data. |
Sensitivity to Assumptions | Sensitive to model specification and assumptions, which might impact the accuracy of estimated propensity scores and subsequent matching. | Less sensitive to model specification as it focuses on exact matching, reducing the influence of assumptions made in the estimation process. |
Frequently Asked Questions (FAQs)
Yes, It can be applied to large datasets. However, matching becomes more complex and computationally intensive with larger sample sizes. Efficient algorithms and computational resources may be necessary for handling big data in PSM.
Overfitting can be a concern in PSM, mainly when the propensity score model is overly complex. Regularization techniques or reducing the number of covariates included in the model can mitigate overfitting issues.
The adequacy of the propensity score model can be assessed by examining the balance achieved in the covariates after matching. Additionally, diagnostic tests, such as checking for overlap in the propensity scores between treatment and control groups, can help validate the model.
Recommended Articles
This article has been a guide to what is Propensity Score Matching. We explain its examples, comparison with coarsened exact matching, limitations, benefits, & steps. You may also find some useful articles here -