Table Of Contents
What Is The Probability Of Default (PD)?
Probability of Default is a financial term used to measure the likelihood that a borrower will default on their debt obligations within a specific time frame. It is a critical component in credit risk assessment and is crucial in credit risk modeling, loan pricing, and portfolio management.
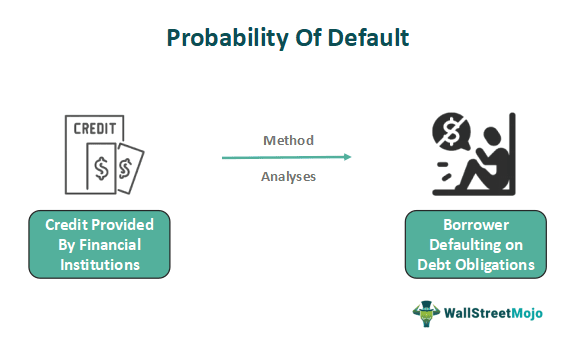
It is often used with other risk measures, such as Loss Given Default (LGD) and Exposure at Default (EAD), to assess a loan or portfolio's overall credit risk. These measures help financial institutions determine appropriate risk management strategies, set risk-based pricing, and allocate capital reserves for potential losses.
Table of contents
- The probability of default measures the likelihood of a borrower defaulting on debt obligations within a specific timeframe. It is an essential tool for credit risk assessment and management. It helps lenders make informed decisions, set risk-based pricing, and allocate capital reserves.
- It is calculated using statistical models and historical data, considering financial health, credit history, and economic conditions. It does not predict future defaults but provides an estimate based on historical data.
- It is a fundamental component of credit risk models and is used in stress testing exercises. Borrowing costs are influenced by it, with a higher probability of leading to higher interest rates or additional collateral requirements.
How Does The Probability Of Default Work?
The probability of default measures the probability of someone defaulting on their debt obligations. Default probability is typically estimated using statistical models and historical data. The approach may vary depending on the institution and the complexity of the analysis, but here is a general overview of how its estimation works:
- Data Collection: Relevant data about borrowers and their credit performance is collected. This includes financial statements, credit bureau reports, loan repayment history, and other relevant factors.
- Data Pre-processing: The collected data is cleaned and prepared for analysis. This involves removing duplicates, correcting errors, and addressing missing values.
- Variable Selection: A set of predictive variables or features is selected based on their relevance to default prediction. These variables include financial ratios, credit scores, industry indicators, macroeconomic factors, and other relevant information.
- Model Development: Statistical models are developed to estimate the probability of default. Commonly used models include logistic regression, decision trees, random forests, and machine learning algorithms. The models are trained using historical data where the default status of borrowers is known.
- Model Calibration: The estimated models are calibrated to improve their accuracy and predictive power. This involves adjusting model parameters and evaluating the model's performance against validation or out-of-sample data.
- Estimation: Once the model is calibrated, it can be used to estimate it for individual borrowers or a portfolio of loans. The borrower's information is inputted into the model, and the model generates a probability of default as an output.
- Validation and Monitoring: The estimated PDs are validated and monitored over time to ensure their accuracy and effectiveness. This involves comparing the predicted default rates with the actual default rates observed in the portfolio.
Formula
The formula for estimating the probability of default varies depending on the modeling technique and approach. Two commonly used approaches are the logistic regression and credit scoring models.
- Logistic Regression Model: The logistic regression model is a statistical technique for estimating probability. It explains the relationship between the borrower's characteristics and the likelihood of default. The formula for the logistic regression model is as follows:
PD = 1 / (1 + e^(-z))
In this formula:
- PD: Probability of Default
- e: Euler's number, approximately 2.71828
- z: The linear combination of borrower characteristics and their corresponding coefficients.
The linear combination (z) is calculated as the sum of the borrower's characteristics (x) multiplied by their corresponding coefficients (β). It can be represented as:
z = β0 + β1x1 + β2x2 + ... + βn*xn
Here:
- β0 is the intercept term.
- β1, β2, ..., βn are the coefficients associated with each borrower characteristic.
- x1, x2, ..., xn are the values of the borrower's characteristics.
The coefficients (β) are estimated using statistical techniques like maximum likelihood estimation during the model training process. These coefficients represent the impact of each borrower's characteristic on the probability of default. By plugging the borrower's elements into the logistic regression formula, we can estimate the likelihood of default.
- Credit Scoring Model: Credit scoring models are based on statistical techniques that assign scores to borrowers based on their characteristics and relationship to the probability of default. The formula for the credit scoring model is as follows:
PD = (A - B * Score)^C
In this formula:
- PD: Probability of Default
- Score: The credit score assigned to the borrower based on their characteristics
- A, B, and C: Parameters estimated during the model calibration process.
The A, B, and C parameters are determined through statistical analysis and calibration to match the scores with historical default rates.
Calculation Examples
Let us understand it in the following ways.
Example #1
Imagine a lending institution wanting to estimate the PD for a borrower based on their credit history and financial ratios. Consider two borrower characteristics: credit score and debt-to-income ratio.
- Data Collection: The lending institution collects data from a sample of borrowers, including their credit scores, debt-to-income ratios, and whether they have defaulted on their loans within a specific period (e.g., one year).
- Data Preprocessing: The collected data is cleaned, removing duplicates or errors and handling missing values.
- Model Development: The lending institution uses a logistic regression model to estimate the PD. The model uses historical data where the default status of borrowers is evident.
Assuming the logistic regression model yields the following coefficients:
Intercept (β0): -2.5
Credit Score (β1): 0.08
Debt-to-Income Ratio (β2): 0.5
- PD Estimation: Let's say we want to estimate the PD for a borrower with a credit score of 700 and a debt-to-income ratio of 0.4.
Using the logistic regression formula, we can calculate the linear combination (z):
z = β0 + β1 * Credit Score + β2 * Debt-to-Income Ratio
= -2.5 + 0.08 * 700 + 0.5 * 0.4
= -2.5 + 56 + 0.2 = 53.7
Next, we can plug the value of z into the logistic function to estimate the PD:
PD = 1 / (1 + e^(-z))
= 1 / (1 + e^(-53.7))
= 1 / (1 + 0) = 1 / 1 = 1
In this example, the estimated PD for a borrower with a credit score of 700 and a debt-to-income ratio of 0.4 is approximately 1 or 100%. This indicates a high likelihood of default based on the historical data and the logistic regression model.
Example #2
According to a report by MSCI, U.S. companies' Probability of Default (PD) has reached its lowest level since January 2023. The data indicates a positive outlook for credit risk in the United States, reflecting improving economic conditions and financial stability.
The report highlights that the PD for U.S. companies declined across various sectors, signaling a reduced likelihood of borrowers defaulting on their debt obligations. This trend aligns with the ongoing economic recovery and the resumption of business activities following the disruptions caused by the COVID-19 pandemic.
MSCI's analysis utilizes statistical models and historical data to estimate PD for various companies. The findings reveal that the credit risk landscape in the United States has improved, indicating greater confidence among lenders and investors.
The declining PD can have significant implications for businesses and financial institutions. Lower default probabilities may result in reduced company borrowing costs, enabling them to access capital at more favorable terms. It also signifies enhanced creditworthiness, making it easier for companies to secure funding and expand their operations.
The positive PD trend in the United States aligns with broader global economic recovery efforts. As countries strive to bounce back from the pandemic-induced downturn, the improved credit risk outlook is an encouraging sign for investors and market participants.
While the current low PD levels are promising, businesses and financial institutions must remain vigilant and continue monitoring credit risk. Despite the positive trend, unforeseen challenges could arise, warranting ongoing risk management practices and prudent lending decisions.
Overall, the declining Probability of Default for U.S. companies, as reported by MSCI, indicates a positive shift in credit risk dynamics. As the economy recovers, businesses and investors can expect a more favorable lending environment, potentially facilitating growth and investment opportunities.
Probability Of Default vs Loss Given Default
Here's a difference analysis between the Probability of Default (PD) and Loss Given Default (LGD) are:
Aspect | Probability of Default (PD) | Loss Given Default (LGD) |
---|---|---|
Definition | Likelihood of a borrower defaulting on debt obligations | Amount or percentage of loss incurred in the event of default |
Measurement | Expressed as a percentage or decimal value between 0-1 | It involves the estimation of expected credit losses |
Purpose | Assess credit risk and default likelihood | Assess potential loss severity in the event of default |
Importance | Assists in assessing the overall credit risk of a portfolio | Described as a percentage or decimal value between 0-1 |
Calculation | Statistical models, historical data, credit scoring | Historical data analysis, recovery rates, valuation adjustments |
Factors | Borrower's financial health, credit history, variables | Collateral value, loan-to-value ratios, security, recovery assumptions |
Relationship | Used in conjunction with LGD and Exposure at Default | Used in conjunction with PD and Exposure at Default |
Impact on Risk | Higher PD indicates higher default risk | Higher LGD indicates higher potential loss severity |
Risk Management | Helps set risk-based pricing, loan provisioning | Guides allocation of capital reserves, risk mitigation strategies |
Portfolio Management | Affects the estimation of expected credit losses | It affects the estimation of expected credit losses |
Probability Of Default And Credit Default Swaps
Some of the critical comparisons between Probability of Default (PD) and Credit Default Swaps (CDS):
Aspect | Probability of Default (PD) | Credit Default Swaps (CDS) |
---|---|---|
Definition | Probability of a borrower defaulting on debt obligations | Financial derivative contract to transfer credit risk |
Measurement | Expressed as a value between 0-1 | Price of the CDS contract or spread (in basis points) |
Focus | Individual borrower or portfolio default probability | Transfer of credit risk associated with specific entities |
Calculation | Statistical models, historical data, credit scoring | Market-based pricing and trading of CDS contracts |
Purpose | Assess credit risk, loan pricing, risk management | Hedging, speculation, credit risk mitigation |
Market Function | Part of credit risk assessment and modeling. | It does not involve direct transfer of risk |
Risk Transfer | Not a contract but a risk measurement or estimation | Transfer of credit risk through the CDS contract |
Parties Involved | Borrowers, lenders, credit rating agencies, regulators | Buyer (protection buyer), seller (protection seller) |
Contract Structure | Not applicable, as PD represents the likelihood of default | Contractual agreement specifying credit protection terms |
Cost and Payment | Not directly associated with cost or payment | Premium payments by the buyer to the seller of the CDS |
Settlement upon Default | An active and liquid market for CDS contracts | An active and liquid market for CDS contracts |
Liquidity and Market | Generally estimated internally by financial institutions | Active and liquid market for CDS contracts |
Regulatory Consideration | PD-based models may be subject to regulatory requirements | Regulatory oversight and reporting for CDS market activity |
Frequently Asked Questions (FAQs)
It directly affects borrowing costs for borrowers. A higher PD indicates a higher perceived risk of default, leading lenders to charge higher interest rates or requires additional collateral or guarantees to compensate for the increased credit risk. Borrowers with lower PDs can generally secure loans at lower interest rates.
While it is commonly used in finance for assessing credit risk, it can also be applied to non-financial entities, such as companies or individuals, to evaluate the likelihood of default on contractual obligations. This modeling and analysis can help non-financial entities make informed decisions regarding creditworthiness and risk management.
This model estimates the likelihood of default based on historical data and borrower characteristics. While they can provide insights into credit risk, it's important to note that these are not crystal ball predictions of future defaults.
Recommended Articles
This has been a guide to what is Probability Of Default. We explain its formula, comparison with loss given default & credit default swaps, and examples. You can learn more about it from the following articles –