Table Of Contents
What Is Prescriptive Analytics?
Prescriptive analytics is the application of logic and mathematics to specify a preferred course of action. It outputs a decision rather than a statistic, probability, or estimate of future outcomes. It is used in predicaments where it is hard to discern the best possible move.
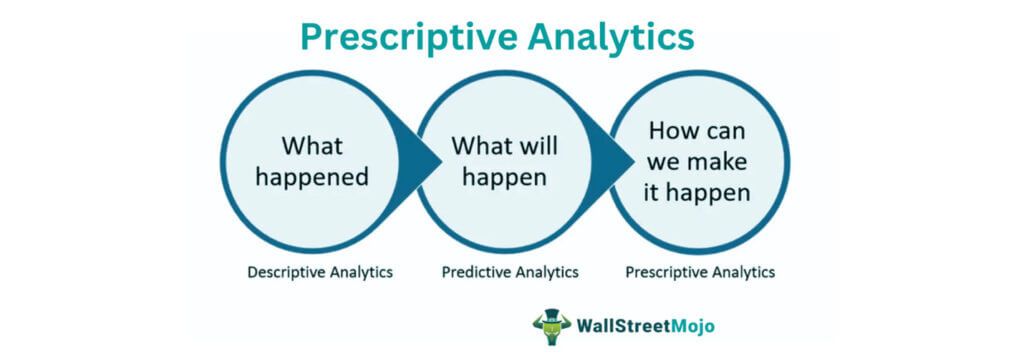
Prescriptive analytics is also known as the "future of data analytics" because it goes beyond realistic explanations or foreseeable predictions. This method employs machine learning algorithms in most applications to accurately process large data sets. Prescriptive analysis absorbs granular details across functions and looks at thousands of scenarios to develop an actionable, optimized plan.
Key Takeaways
- It is also called the future of data analytics and is often referred to as business analytics when applied in business management and decision-making processes.
- Machine learning and operations research are two important prescriptive analytics tools. First, they are used to understand consumers' patterns and behavior.
- More and more businesses are now adding prescriptive analysis to their arsenal. This way, they can research business processes exhaustively and deliver better results.
- Prescriptive analysis optimizes decision-making within a business sector. In addition, it can also be used to optimize decisions across multiple sectors.
Prescriptive Analytics Explained
Prescriptive analytics uses big data to suggest a business's best course of action. The data is in the form of past trends, market patterns, purchase history, buying behavior, likes, dislikes, amount of time spent on something, performance-based reviews, surveys, etc.
It is an important component of data analytics (also known as business analysis). Data analytics is divided into four stages:
- Descriptive
- Diagnostic
- Predictive
- Prescriptive
From the prescriptive analytics definition, we gather that it is used when there are large sets of available data and information (big data). This could be the open feed of Instagram, shifts in advertising, marketing trends, or sales techniques. Firms rely on how consumers respond. In prescriptive analysis, customer response to each firm decision is recorded.
Using prescriptive analysis, firms predict YouTube suggestions, artificial intelligence, sales figures, email marketing, cold calls, election campaigns, voting trends, messaging sprees, and customized playlists. Then, based on the predictions, firm action plans are formulated.
It is important to note that prescriptive analysis should not be confused with predictive analysis. Both sound similar, but they are different. Predictive analytics is used to forecast future trends or what may happen. But prescriptive analytics goes a step further by offering a plan of action to deal with big data.
There are two primary approaches to prescriptive analysis. The first approach leverages optimization techniques like linear programming. The second approach leverages computational logic (say, predefined business rules for decision-making).
Optimization runs through all possible options to achieve the best answer. It respects every constraint and objective outlined by the business. A rules-based approach to prescriptive analysis uses pre-existing business knowledge to ensure that future decisions comply. The rule bases approach is limited and yields only a few options. It works if the decision is simple. But for most real-world applications, optimization is a better fit.
Examples
Let us look at some prescriptive analytics examples to understand prescriptive analytics better.
Example #1
Chloe works in a marketing agency, her boss recently assigned her a new political marketing campaign, and she is very excited about it. Though hardworking, she doesn't want to make any mistakes, so she conducts thorough research on how she should move forward with such a massive campaign.
During her research, Chloe comes across a similar campaign initiated two decades ago. A company with the same plan initiated it. Using historical data, Chloe learns all the steps undertaken by the company.
Further, she tracks her newly assigned campaign's progress—she records every minute detail. With steady progress, Chloe met her target—she was on the right track. Information from the previous campaign helped her decide the best course of action, and she followed it diligently to reach her objectives.
Example #2
Cybercrimes have been on the rise. This has created a new demand for crime prevention and prediction. Thus the market share of prescriptive analytics is going to increase.
Many businesses try to include prescriptive analysis based on IoT (Internet of Things). In addition, firms are also employing machine learning, big data, and real-time analytics to obtain a competitive advantage over the market.
According to Market Research Future (MRFR), the prescriptive analytics market share is expected to reap approximately $16.23 Billion by 2030, with a CAGR of 21.2%.
The prescriptive analysis is further categorized based on vertical, organization, business sector, components, application, and deployment.
Uses
- Data scientists use it to process sets of large data effectively and accurately. However, it is challenging and time-consuming if done manually (without prescriptive analysis). In addition, if done manually, there would be more errors.
- Prescriptive analytics techniques are based on data-driven decision-making to offer the best possible solutions.
- In business, it is used to comprehend consumer behavior and the nature of their buying process.
- It plays a huge role in sales and marketing. It charts the best strategy that could influence the target audience. But, again, this method employs tons of data—customers' mindsets, trending patterns, and market accessibility.
- This method process all the given factors and outputs a roadmap—like a guide to deal with critical scenarios.