Table Of Contents
What Is The Power Of A Test?
The power of a test is a concept in statistics that signifies the possibility of rejecting a null hypothesis when it is false. Measuring this power before conducting the test enables the user to ensure that the test's sample size is extensive enough for the aim of the test.
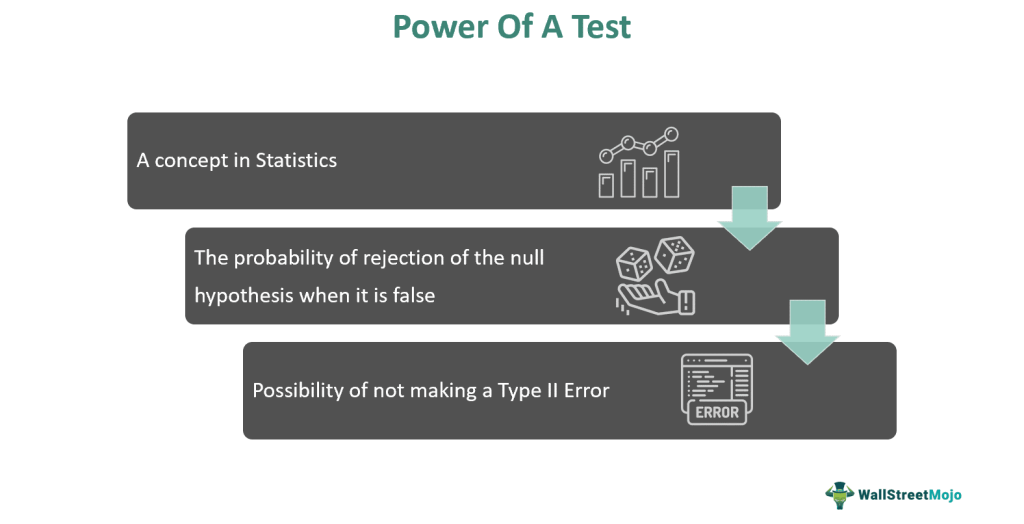
This power shows the likelihood of not making a Type II error. It assists in quantifying the probability that it will result in the rejection of a null hypothesis when it is actually false. It implies that the power is the test's capability to reject the null hypothesis accurately.
Key Takeaways
- The power of a test is a statistical concept that signifies the chances of rejecting a null hypothesis when it is false. It evaluates the possibility of not making a Type II error.
- The power aids in quantifying the possibility of the test to reject the null hypothesis when it is false. It depicts the test's ability to reject the null hypothesis accurately.
- The factors influencing the test's power include standard deviation, significance level, and the test's sample size. The power can be increased by decreasing the variability in the sample or reducing its significance level.
Power Of A Test Explained
The power of a test is a measure of a test's potential to reject the null hypothesis correctly. Consequently, it determines whether the test has the potential not to make a Type II error. This measure aids in quantifying the possibility that the test will reject the null hypothesis when it is indeed false. This is a significant concept used in statistics.
A hypothesis test can be conducted without using the power of a test in statistics. However, if users measure it beforehand, it will allow them to determine if the sample size of the test is sufficiently extensive for the purpose of conducting the test. Otherwise, the test may turn out to be inconclusive, resulting in the waste of valuable resources. Sometimes, users may calculate a test's power after the test is conducted in a follow-up study. It helps to ensure that the sample size is adequate for the test.
Factors
The factors that impact a test's power are as follows:
- Sample size of the test: The test's sample size can influence its power as a larger sample size ensures a higher test power. The user has control over the sample size, so they can increase it to increase the power.
- Standard deviation: A test's power is higher when the standard deviation is smaller instead of significant. Users can manage the standard deviation by decreasing random measurement errors and by ensuring that the experimental methods are applied with consistency. They may also control it by taking samples from a homogeneous population.
- One- and Two-Tailed Tests: The power is higher in a one-tailed test as compared to a two-tailed test. However, the hypothesized direction must be accurate.
- Significance Level: When the significance level is lower or more stringent, the test's power is lower. If the evidence required to reject the null hypothesis is strong, then the odds of the rejection of the null hypothesis will be low.
Formula
The power of a test formula is as follows:
Power = 1- β = P(Reject H0 | H0 is false)
Examples
Let us understand this concept with the help of the following examples:
Example #1
Suppose a company decided to give an increment to its customer service team if at least 80 percent of the company's customers were very satisfied. It surveyed 100 out of its 1,000,000 customers using the random sampling method. The company wanted to evaluate if the employees would get a raise in their salaries or not. The null hypothesis stated that the fraction of very satisfied customers is at least 0.80. If the null hypothesis cannot be rejected, with a significance level of 0.05, the company would pay the increment. The true fraction of satisfied customers is 0.75.
The null hypothesis tests the hypothesis that the fraction of very satisfied customers is 0.80. The company focussed on estimating the probability that the hypothesis test will reject the null hypothesis if the true satisfaction level is 0.75. Therefore, the critical parameter value established was 0.75. The power of a test formula is the probability of rejecting the null hypothesis with the assumption that the true population fraction is equal to the critical parameter value. The region of acceptance is 0.734 to 1.00. Therefore, the null hypothesis will be rejected when the sample proportion is less than 0.734.
Example #2
Suppose a study revealed that the average time per week kids spend playing is 2 hours. The alternative suggested that it is greater than 2 hours. The study decided to calculate the power of a test for a specific value under the alternative hypothesis, for instance, 3 hours. The Null Hypothesis is H0: = 2 hours. The Alternative Hypothesis is H1: = 3 hours. μ represented the average time per week that kids spent playing. Under the null hypothesis, μ is depicted as μ0, and under the alternative, it is depicted as μ1. So μ0 = 2 and μ1 = 3. The standard deviation from previous data was 1 hour. The study used a sample size of 1,000 kids to conduct the test.
How To Increase?
The test's power can be increased in the following ways:
- Increasing the difference between the null and alternative values or the effect size that is to be detected.
- Increasing the test's sample size. With an increase in the sample size and a decrease in the standard error or an increase in the difference between the sample statistic and the hypothesized parameter, the p-value decreases. Therefore, the possibility of rejecting the null hypothesis becomes high.
- Decreasing the variability in the sample.
- Increasing the test's significance level.