Table Of Contents
What Is Panel Data Analysis?
Panel data analysis is a statistical method used in social sciences and economics to examine data gathered over time from multiple individuals, groups, or entities. This approach allows researchers to study the differences between individual subjects and the changes within the same subjects over time.
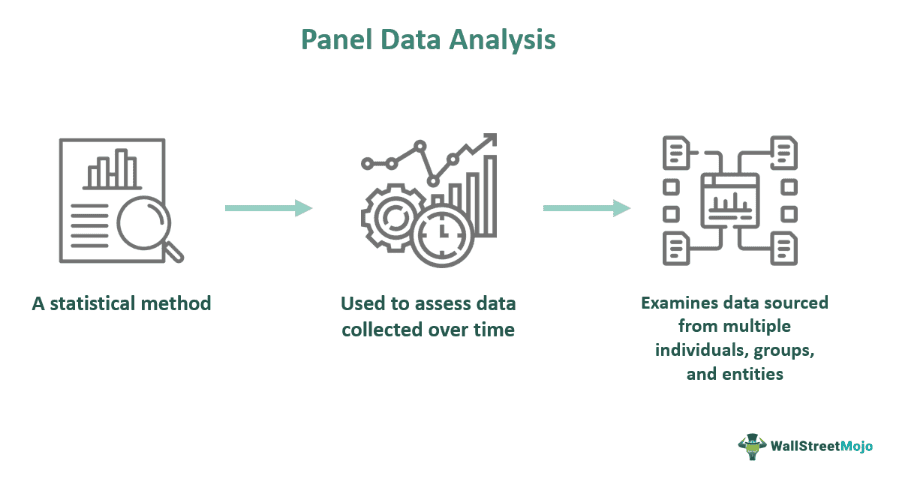
This analysis is also known as longitudinal or repeated measures data. It combines both dimensions and offers valuable insights into individual behaviors and the dynamics of change. The data analysis allows a greater understanding of complex relationships by capturing both within-unit variations and between-unit variations. Moreover, it effectively accounts for individual-specific effects and time-related influences.
Key Takeaways
- Panel data analysis is a statistical tool for thoroughly examining data obtained from several entities over time. It enables researchers to examine changes within the same subjects and variations among the subjects.
- The technique often results in exact estimates and greater statistical power. It optimizes data usage by incorporating information from time and entities.
- As a result, estimators are more effective, and correlations between variables can be identified with more accuracy.
- However, gathering data over time could be costly and time-consuming. Furthermore, imbalanced or missing data may distort the analysis.
Panel Data Analysis Explained
Panel data analysis is a statistical method used to examine data collected over time from multiple entities comprehensively. It is instrumental in capturing within-subject variations and between-subject differences simultaneously. The analysis involves observing the same entities repeatedly over a period, which allows researchers to study the differences between individual subjects along with the changes within the same subjects across different time points.
The versatility offered by this data analysis enables a diverse range of research questions to be addressed. It allows for studying the effects of policies or interventions over time. Additionally, it aids in understanding the impacts of socioeconomic factors on individual behaviors or market trends. Furthermore, it helps in evaluating the influence of external factors on business performance. The method's ability to capture both individual diversity and time-related dynamics allows for robust findings.
Methods
The panel data analysis methods include:
#1 - Fixed Effects Models
These models account for individual-specific effects by introducing dummy variables for each entity in the dataset. This method controls for time-invariant individual characteristics and allows the examination of changes within the same entity across different time points. Fixed effects models help identify individual variations while focusing on within-subject differences over time.
#2 - Random Effects Models
The random effects models assume that the individual-specific effects are random and uncorrelated with the regressors. These models estimate the average effect of variables across the entire sample. They consider both within-group and between-group variations. Random effects models are beneficial when examining changes that affect the entire group or sample under study.
Process Steps
The steps in panel data analysis are:
- Users must collect longitudinal data that includes information on the same entities over time. They must begin by conducting descriptive statistics to understand the data's primary characteristics. Additionally, they must conduct summary statistics, like means, standard deviations, and distributions, to gain insights into the variables involved in the analysis.
- Then, they must decide on the specific panel data model best suited for the research question. Users must implement the chosen panel data model using statistical software. They must estimate the model parameters while accounting for individual and time-specific effects. This step involves running regressions or specific panel data models with the selected variables.
- Next, users must conduct hypothesis tests to assess the significance of the variables and parameters in the model. They may also perform robustness checks to ensure the reliability of the findings. Furthermore, users must conduct diagnostic tests to validate the assumptions of the chosen model. They may check for serial correlation, multicollinearity, or other statistical issues that affect the reliability of the results.
- Finally, users may interpret the results in the context of the research question and objectives. They must report the findings, including the methodology, results, limitations, and conclusions.
Examples
Let us study the following examples to understand this analysis:
Example #1
Suppose Jake wanted to analyze the impact of interest rates on the savings of different individuals over five years. The data included information on the savings behavior of 100 account holders in a bank. Jake employed a data analysis to track each account holder's savings over five years and observe fluctuations in interest rates. Using a fixed effects model, he controlled the individual-specific traits, including risk tolerance and income levels, to isolate the impact of interest rates on savings.
Example #2
Conventional spatial models' assumptions are unable to estimate the reactions over time and across space. Taiwan's National Health Insurance Database provided the data for a longitudinal or panel data study. An algorithm was created to determine the patient's place of residence and calculate the ESRD ( End-stage kidney disease) rate for every township.
Corresponding covariates were gathered, such as patient comorbidities, medication usage history, and socioenvironmental factors. Local spatial clustering around a specific area was described using local Indicators of spatial association. Additionally, a geographical panel data model was established to investigate the relationship between risk variables and the incidence of ESRD.
When To Do?
Panel data analysis can be applied in the following cases:
- Time-Series and Cross-Sectional Data: The analysis is ideal when dealing with time-series and cross-sectional data. It allows researchers to study changes over time while considering differences among different entities or individuals.
- Individual-Specific Effects: It is applicable when there is a need to control the individual-specific effects that might impact the analysis, like varying characteristics or traits. The analysis can effectively address these individual differences.
- Longitudinal Studies: This analysis is valuable for longitudinal studies where the same subjects are observed over multiple time points. It enables researchers to explore changes within the same entities and offers a more comprehensive understanding of the evolution of variables over time.
- Policy Evaluation: The analysis can be beneficial for evaluating policy impacts or interventions, including studying the effect of a new law or a change in economic policy. Moreover, it can provide deep insights into the policy effects.
- Market Research and Economic Studies: In economics and market research, studying how different factors impact individuals, firms, or markets over time often employs this data analysis. It helps in understanding the dynamics of economic changes and market behaviors.
Advantages & Disadvantages
Some advantages of panel data analysis are as follows:
- One of the primary advantages of panel data analysis is its capability to control for individual-specific effects. It accounts for individual heterogeneity and allows researchers to isolate the impact of time-varying factors while controlling individual characteristics that remain constant over time.
- The analysis often provides precise estimates and increased statistical power. It maximizes the data use by incorporating information from time and entities. This results in efficient estimators and increased accuracy in identifying relationships between variables.
- The method can accommodate heterogeneity, which is often a challenge in other research designs. The analysis can reduce the impact of unobserved factors that affect outcomes by examining how different entities or individuals respond to changes over time. It aids in providing more accurate and comprehensive results.
The disadvantages of panel data analysis are:
- The analysis demands extensive data on the same entities over time. Data quality, consistency, and completeness are crucial, as missing or unbalanced data can affect the analysis. Moreover, data collection over time may be expensive and time-consuming.
- One of the significant disadvantages of panel data analysis is that it requires advanced statistical knowledge and specialized software. Interpreting the results is also more complex, which can sometimes be challenging to explain and interpret accurately.
- Certain assumptions in panel data models, like the assumption of no serial correlation, might be violated. Moreover, the selection of an inappropriate model might lead to biased or unreliable results.