Omitted Variable Bias
Published on :
21 Aug, 2024
Blog Author :
N/A
Edited by :
Shreeya Jain
Reviewed by :
Dheeraj Vaidya
What Is Omitted Variable Bias (OVB)?
Omitted variable bias (OVB) refers to the bias created by researchers where one variable gets left out or rejected during a study. This type of bias usually occurs when the researchers need to provide proper specifications of the linear regression model and related variables.
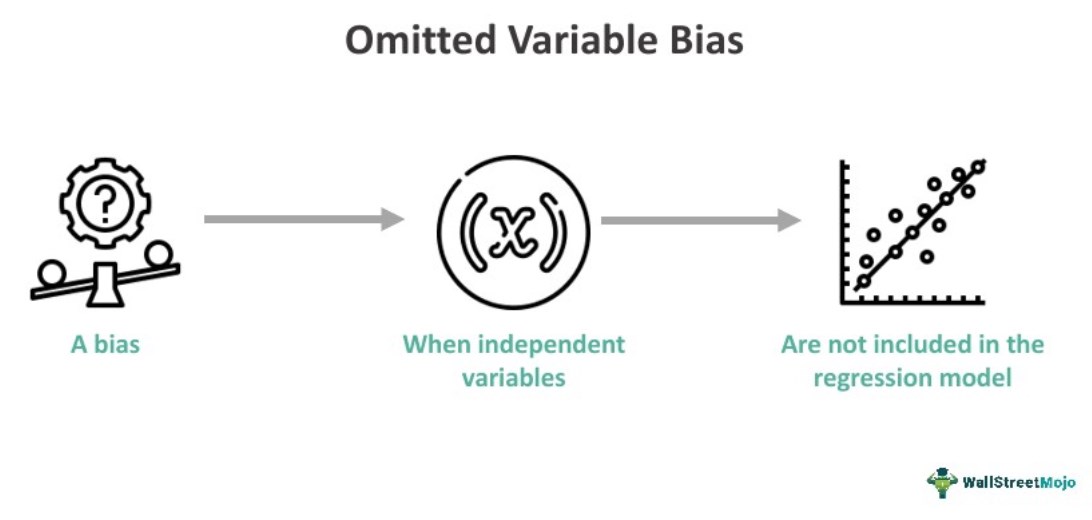
The source of omitted variable bias direction depends on the confounding variables. They are responsible for creating bias on omission. As a result, a distortion occurs within research. Also, there is a hidden effect visible on the other variables present. Due to this, a significant change might affect their relationship, too.
Table of Contents
- Omitted variable bias is a distortion created when one variable is either omitted or ignored within research. It is a type of bias observed in linear regression models.
- Moreover, it also occurs due to the presence of confounding variables in the study. They are highly responsible for cause-and-effect relationships with other variables.
- The formula for OVB can be simplified as β0 + β1∗ X + β2 ∗ u + ε, where β is the coefficient of the plane. X is the independent variable, and u refers to the omitted variable.
- However, there are many ways to avoid the presence of such variables in the research.
Omitted Variable Bias Explained
Omitted variable bias refers to a concept that focuses on the variables omitted during the study. Therefore, this bias usually exists due to the presence of confounding variables. These variables try to influence the cause-and-effect relationship in research. So, if a researcher tries to exclude them, the process will alternatively correlate its effect on other variables. As a result, the effect of the confounding will be visible, even though the variable is absent. Likewise, a bias will also emerge in such a situation. However, if the researcher continues to follow it, there can be severe consequences to the data findings.
Furthermore, for a particular variable to create such bias, it is necessary to fulfill some conditions. Let us look at them:
Firstly, the omitted variable must be a determinant of the dependent variable.
Hence, the omitted variable must be correlated to more than one independent variable in the linear regression model. It means one or more such variables exist already in the model.
If the above conditions are met, the confounding variable counts as an omitted variable bias proof. For instance, a total population study has variables like age, region, and gender. However, the exclusion of occupation (confounding variable) widely affected the final observation. In the later stages, it also led to endogeneity. It refers to a situation where the variable in the error term also correlates to the independent variable. So, if an omitted variable links to both independent and dependent variables, endogeneity occurs. As a result, the data findings witness a similar effect. Thus, the linear regression model's ambiguity is the cause of this bias.
Formula
In contrast to the above biases created, it is vital to identify them before they occur in the model. Also, if they already exist, it is necessary to understand their cause-effect relationship. So, let us derive the omitted variable bias equation for better understanding:
Omitted variable bias = ^β1p → β1+ρXu (σu /σX)
Here, in the above equation, β1 refers to the coefficient (or slope) of the regression plane. Also, ρXu is the correlation of variables X and u. σ refers to the standard deviation of both variables.
However, for easy calculation, it is further derived as below;
OVB = β0 + β1∗ X + β2 ∗ u + ε
Here, the β0 acts as an intercept of the true regression line (the value of y is prominent when x is zero). X refers to the independent variable in the linear regression model. And u is the omitted variable that should have been included in the model. Similarly, β2 is the associated coefficient of variable u. Lastly, ε is the error term that explains the variation created.
Examples
Let us look at some examples and applications of omitted variable bias to comprehend the concept better:
Example #1
Suppose Kevin is a researcher who is working on a postgraduate project. He wishes to know the employee's income based on experience (X), skills (u), and education (Y). While plotting the linear regression model, Kevin included experience and education. But, during this process, he forgot to consider the skills. As a result, the coefficient obtained focused on X and Y and not u.
Later, when Kevin's professor pointed out his mistake, he sat to analyze the model and found the omitted variable. Thus, with the help of an omitted variable bias direction formula, he figured out the effect of this variable. Following is the calculation:
Error term (ε) = β3 * ρ
= 0.5 * 2 = 1
OVB = β0 + β1∗ X + β1∗ Y + β3 ∗ u + ε
(since it has three variables)
= 0 + 0.5 + 0.6 + 0.66
= 1.36
The above value obtained indicates that the inclusion of skills would have resulted in more accurate results, followed by education and experience.
Example #2
A recent research paper, "Effect of flourishing on suicidal ideation in midlife," published in October 2023, speaks about the different variables affecting the active lives of individuals. From a group of 1619 participants, half represented flourishing (X), and the rest were of suicidal ideation (Y).
In this case, W represents the omitted or confounding variable. It includes different factors like binge drinking, health status, personality factors, health insurance, socioeconomic factors, psychological distress, depression, anxiety, and chronic pain. The researchers cross-check the results with an omitted variable bias equation, IV (Instrumental variables), and sensitivity analysis.
Implications
Since an omitted variable acts as an alternate element, there are many consequences or implications related to it.
Omission variable bias proof also leads to upward or downward bias of the independent variables. Here, the bias created leads to overestimation or underestimation of the coefficients. For instance, the results derived may need to be more rated or underrated. As a result, the research may see a wide deviation. Also, the associated coefficients need to be more reliable.
Likewise, this bias can also provide a hidden effect to the study. It means that the omitted variable may affect the research even if not included. Hence, a profound effect will still prevail in its absence.
In addition, it may also lead to loss of efficiency of the study performed. Relying on misrepresented models can cause inaccurate predictions. Moreover, excluding these variables for a long time may lead to poor decision-making.
How To Avoid?
There are various ways to avoid omitted variable bias proof in the linear regression model. Researchers can deploy control variables in the absence of data during the study. It acts as a constant to avoid any undue influence on the model. Similarly, even proxies could be helpful in the same process.
Another way to avoid such bias is to determine the omitted variable bias direction. It will help in estimating the mode where the research is more biased. For instance, signing the bias as positive and negative can avoid the presence of such variables. In addition, checking the background of the study can help identify prominent variables that can affect the research.
However, sometimes, it may need to be clarified or tricky to detect such bias. At such points, it is necessary to check the residual plots for any confounding variables.
Omitted Variable Bias vs Selection Bias
Although omitted variables and selection bias have a similar bias created, they do have differences in their functions. Let us look at them:
Aspect | Omitted Variable Bias | Selection Bias |
---|---|---|
Meaning | It refers to the bias created when one variable gets ignored or left out. | Selection bias is caused by a non-random or intentional bias during the sample selection. |
Occurrence | This bias occurs due to the omission of variables. | Moreover, it occurs in the sample selection itself. |
Type of bias | These are a type of internal bias that exists in the regression model itself. | Here, it is a form of systematic error. |
Frequently Asked Questions (FAQs)
Following are the ways to detect OVB in research. Let us look at them:
1 Here, the variables are often visible in the observational study.
2 However, the inclusion of instrumental variables can help in such detection.
3 In addition, consultation with experts for pre-detection of omitted variables.
Omitted variable bias generally occurs when the model fails to incorporate one or more variables in the research. As a result, it may lead to the exclusion of an important factor, which may significantly impact the model. In short, it acts like an opportunity cost that could give positive results.
The direction of the omitted variable depends on the correlation with the independent variable. If B (independent) and U (omitted) have a positive correlation, the latter may create a positive bias on A (another independent variable). Likewise, a negative correlation creates a negative bias.
The foremost way to fix OVB is to include the omitted variable if it has a higher coefficient. Likewise, random assignment can also disperse the confounding effect.
Recommended Articles
This article has been a guide to what is Omitted Variable Bias. We explain its formula, examples, how to avoid it, comparison with selection bias, and implications. You may also find some useful articles here -