Table Of Contents
What Is Nonparametric Statistics?
Nonparametric statistics is a branch of statistics that deals with data that do not follow a specific distribution. The purpose of nonparametric statistics is to provide statistical methods that are robust and applicable in a wide range of situations without the need to assume a specific distribution or estimate parameters.
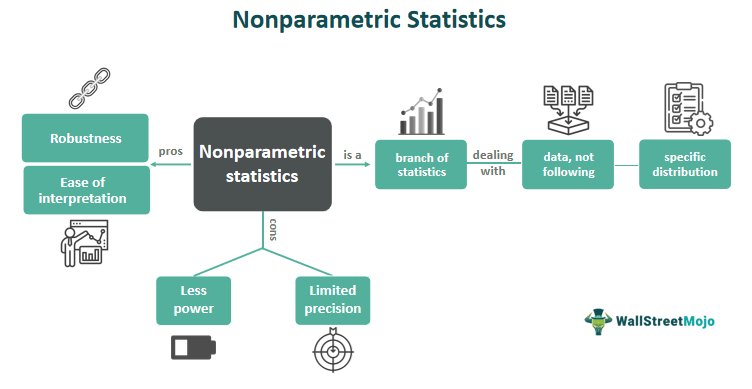
The importance of nonparametric statistics lies in its ability to handle data that is not normally distributed or may have unknown or complex distributions. It is also useful when the sample size is small, or the data is ordinal or nominal. In addition, nonparametric statistics can be used to test hypotheses, estimate parameters, and make predictions.
Key Takeaways
- Nonparametric statistics do not require assumptions about the data distribution or population parameters, making them more flexible than parametric methods.
- Nonparametric methods can be used with nominal, ordinal, interval, or ratio data. These are particularly useful when the data violates the assumptions of parametric methods, such as normality or homogeneity of variance.
- Nonparametric methods are less sensitive to outliers and can produce accurate results with small sample sizes.
- Nonparametric methods are generally less powerful than parametric methods when assumptions are met, but they are still useful in situations where parametric methods cannot be used.
Nonparametric Statistics Explained
Nonparametric statistics is a set of methods that can be used to analyze data without making assumptions about the underlying population. This approach is useful when the data does not follow a specific distribution or when the sample size is small. Unlike parametric statistics, nonparametric methods do not require estimating population parameters, such as mean and variance. Instead, they rely on rank-based tests, such as the Wilcoxon rank-sum test, to compare groups or test hypotheses.
Its methods are often used in social sciences, psychology, medicine, biology, and engineering, among other fields. It is a flexible approach that does not make assumptions about the underlying population and uses methods that do not depend on parameters.
Nonparametric methods can also be used to analyze nominal data or ordinal data, meaning the values cannot be measured on a numerical scale. For example, nonparametric methods can be used to compare the proportion of people who prefer a certain brand of cola or to test whether a certain drug effectively reduces pain.
Assumptions
Assumptions in statistics are conditions or requirements that must be met for a statistical method to be valid. Nonparametric methods do not require strong assumptions about the data distribution or population parameters. However, they still have some assumptions that need to be met, such as random sampling, independence, and symmetry of the distribution. Nonparametric methods also assume that the data is at least ordinal, meaning the values can be ranked or ordered.
Examples
Let us have a look at the example to understand the concept better.
Example #1
Suppose an investor wants to compare Portfolio A's and Portfolio B's returns. The investor collects data on each portfolio's daily returns over one year and tests for normality using a Shapiro-Wilk test. The test reveals that the data is not normally distributed, violating the assumption of parametric methods.
In this case, the investor might use a nonparametric method such as the Wilcoxon rank-sum test or the Mann-Whitney U test to compare the median returns of the two portfolios. These nonparametric methods do not require the data to follow a specific distribution. In addition, they are less sensitive to outliers, making them an appropriate choice for analyzing financial data that may have non-normal or non-symmetric distributions.
Example #2
Suppose a researcher wants to test whether there is a significant difference in the median salaries of employees in two different departments of a company. They collect salary data from a random sample of employees in each department and find that it is not normally distributed. In this case, the researcher can use nonparametric methods, such as the Wilcoxon rank-sum test.
It helps to compare the median salaries of the two departments without assuming any specific distribution or estimating population parameters. The test will give a p-value that indicates whether the difference in median salaries is statistically significant or due to chance. This approach is useful when the data does not meet the assumptions of parametric tests, such as the t-test or ANOVA.
Advantages And Disadvantages
Let us have a look at the pros and cons of the concept:
Advantages of nonparametric statistics include:
- Flexibility: Nonparametric methods can be used with data that does not meet the assumptions of parametric methods, such as non-normal or non-homogeneous data.
- Robustness: Nonparametric methods are less sensitive to outliers and can produce accurate results even with a small sample size.
- Ease of interpretation: Nonparametric methods often use simple statistics, such as ranks or medians, which are easy to understand and interpret.
Disadvantages of nonparametric statistics include:
- Less power: Nonparametric methods are generally less powerful than parametric methods when the assumptions are met. Nonparametric tests may require a larger sample size to detect a true difference.
- Limited applicability: Nonparametric methods are unsuitable for all data types, such as continuous data that follows a normal distribution.
- Limited precision: Nonparametric methods may produce less precise estimates than parametric methods, especially when the sample size is small.
Nonparametric Statistics vs Parametric Statistics
Nonparametric Statistics
- Do not assume a specific distribution or estimate population parameters
- Suitable for data that does not meet the assumptions of parametric methods, such as non-normal or non-homogeneous data
- Use rank-based tests or other nonparametric methods
- Are generally less powerful than parametric methods when assumptions are met
- Are less sensitive to outliers and can produce accurate results with a small sample size
- Produce less precise estimates than parametric methods, especially with small sample sizes.
- Do not require estimating population parameters, such as mean and variance.
- It can be used with nominal or ordinal data
Parametric Statistics
- Assume a specific distribution and estimate population parameters
- Suitable for data that follow a specific distribution, such as normal or binomial
- Use mean-based tests, such as t-tests or ANOVA
- Are generally more powerful than nonparametric methods when assumptions are met
- Are more sensitive to outliers and may require a larger sample size to detect a true difference
- Produce more precise estimates than nonparametric methods, especially with large sample sizes.
- Require the estimation of population parameters
- Require interval or ratio data