Table Of Contents
What Is Model Validation?
Model validation in finance refers to the process of assessing the accuracy, reliability, and appropriateness of financial models used for decision-making within a financial institution. It ensures that the model produces results that are consistent with real-world observations and expectations. This involves comparing the model's outputs with historical data or alternative methodologies to gauge its predictive power.
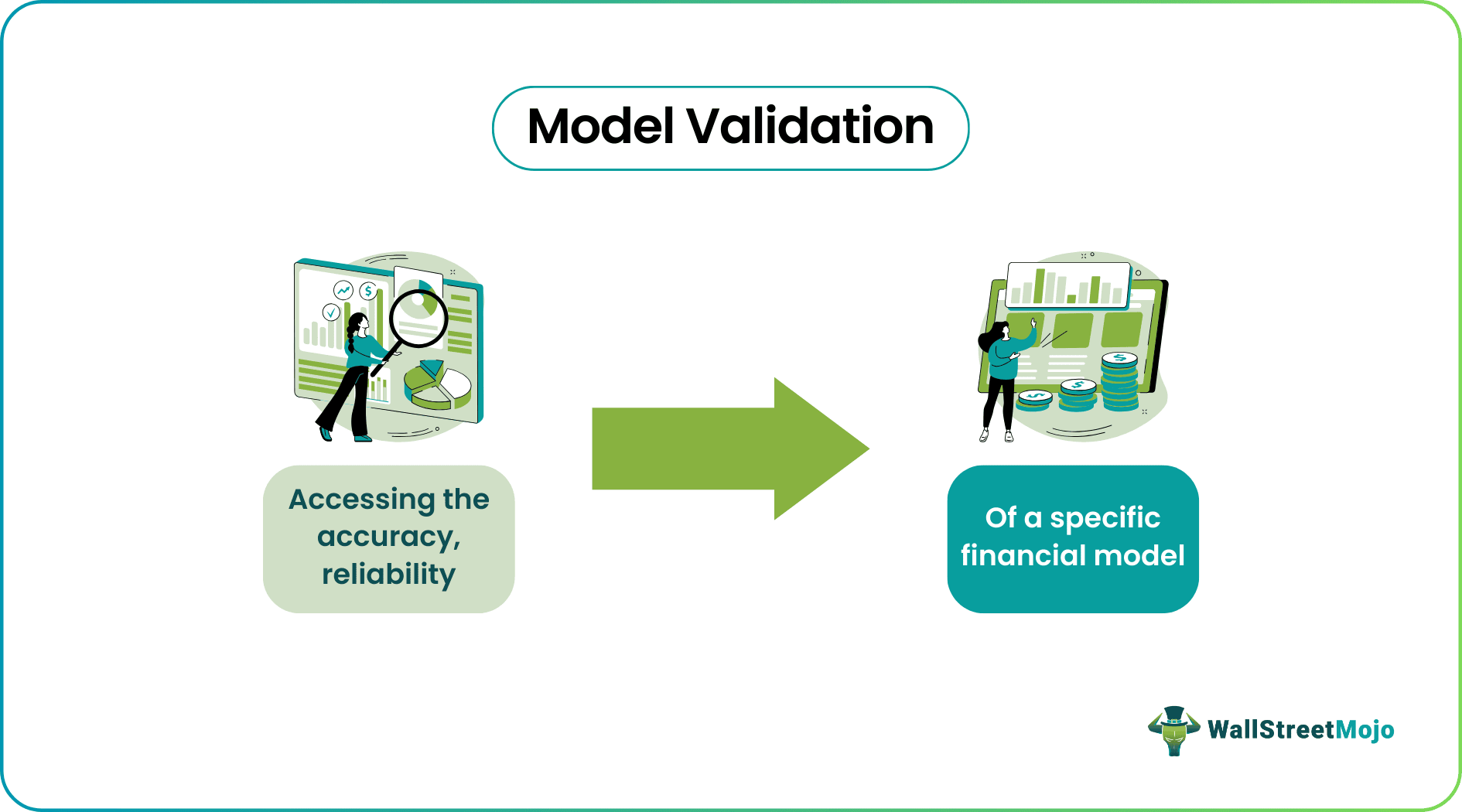
Validating models helps identify and quantify the potential risks associated with using them for decision-making. By understanding the limitations and assumptions of a model, financial institutions can better manage and mitigate risks related to market volatility, credit default, or operational failures.
Table of Contents
- Model validation is the process of scrutinizing and assessing the accuracy, reliability, and appropriateness of financial models employed for decision-making within the financial sector.
- It ensures that financial models produce accurate and reliable results consistent with real-world observations.
- Validating models helps identify and quantify risks associated with their use, enabling better risk management practices within financial institutions.
- It is essential for meeting regulatory requirements and demonstrating adherence to industry standards and best practices.
Model Validation In Finance Explained
Model validation involves a systematic evaluation process to confirm that a model appropriately represents the underlying financial phenomena it intends to capture. It originated from the need to ensure the reliability and accuracy of mathematical and statistical models used to analyze financial markets, instruments, and risks.
It gets started by assessing the underlying theoretical framework of the financial model. This involves a thorough examination of the model's assumptions, logic, and methodologies. This ensures they align with financial principles and market dynamics. Next step is validating the quality and integrity of the data used to develop and calibrate the model. This involves scrutinizing the sources, accuracy, completeness, and timeliness of the data.
It is to ensure it adequately represents the real-world financial environment. Model validation is rooted in the necessity to uphold quantitative rigor, enhance risk management practices, deepen market understanding, promote transparency and accountability, and foster continuous improvement within financial institutions.
Techniques
Model validation employs a variety of techniques to assess the accuracy, robustness, and appropriateness of financial models. These techniques include:
- Backtesting: This involves comparing the outputs of a model with historical data to evaluate its predictive power and assess how well it captures real-world phenomena.
- Sensitivity Analysis: Sensitivity analysis examines how variations in inputs or assumptions affect the outputs of a model. It helps identify critical drivers of the model's results and assesses its robustness to changes in parameters.
- Scenario Analysis: This technique involves running simulations based on different hypothetical scenarios to understand the potential impact of various market conditions on the model's outputs. It helps assess the model's resilience and ability to adapt to different circumstances.
- Monte Carlo Simulation: Monte Carlo simulation generates thousands of random scenarios based on probability distributions of model inputs to estimate the range of possible outcomes. It provides insights into the distribution of risks and uncertainties associated with the model.
- Comparative Analysis: This involves comparing the outputs of the model with alternative models or benchmarks to evaluate its relative performance and identify areas for improvement.
Examples
Let us understand it better with the help of examples:
Example #1
Consider a bank's credit risk model designed to predict the likelihood of loan default. Through backtesting, the model is validated by comparing its predictions to historical loan performance data. Sensitivity analysis is applied to assess how changes in economic indicators impact the model's outputs. Scenario analysis is used to simulate the impact of a severe economic downturn on loan portfolios.
Monte Carlo simulation generates a range of possible default scenarios. Comparative analysis compares the bank's model with industry benchmarks. Validation metrics, including accuracy and precision, confirm the model's reliability. This comprehensive approach ensures the model's effectiveness in assessing credit risk in diverse market conditions.
Example #2
In a strategic move of 2023, Murex, a leading provider of trading and risk management solutions, has expanded its offerings by partnering with a prominent financial information and analytics firm. This collaboration aims to bolster Murex's ability to validate complex financial models effectively. By integrating the expertise and resources of the analytics leader, Murex seeks to provide its clients with enhanced validation services, ensuring the accuracy and reliability of their trading and risk management models.
The partnership underscores Murex's commitment to staying at the forefront of technological advancements and addressing the evolving needs of financial institutions in an ever-changing market landscape. With access to comprehensive financial data and advanced analytical tools, Murex aims to deliver robust validation solutions that empower its clients to make informed decisions and navigate market uncertainties with confidence.
Best Practices
Adhering to best practices in model validation is essential for ensuring the accuracy, reliability, and regulatory compliance of financial models. Some critical best practices include:
- Clear Documentation: Thorough documentation of model development, assumptions, methodologies, and validation procedures is crucial. Clear documentation facilitates transparency, reproducibility, and understanding by stakeholders and regulators.
- Independent Validation: Model validation should be conducted by independent parties, separate from those involved in model development or usage. This independence helps mitigate biases and conflicts of interest, ensuring impartial assessments.
- Robust Validation Framework: Establishing a comprehensive validation framework that covers all aspects of model validation, including data integrity, model conceptual soundness, implementation, and outcomes analysis, is essential. This framework should align with industry best practices and regulatory guidelines.
- Risk-Focused Approach: Prioritize validation efforts based on the level of risk associated with the model and its impact on decision-making. Focus validation resources on high-risk models or those with significant business implications.
- Continuous Monitoring and Review: Implement processes for ongoing monitoring and review of model performance post-implementation. This includes tracking model outcomes against actual results, assessing model recalibration needs, and updating validation procedures as necessary.
Importance
Model validation holds immense importance in finance for several key reasons:
- Risk Management: Validating financial models helps identify and quantify risks associated with using them for decision-making. By understanding the limitations and assumptions of a model, financial institutions can better manage and mitigate risks related to market volatility, credit default, or operational failures.
- Regulatory Compliance: Financial regulations require institutions to validate their models to ensure they meet specific standards of accuracy, reliability, and transparency. Compliance with regulations such as Basel III, Dodd-Frank Act, or Solvency II often necessitates rigorous model validation processes.
- Informed Decision-Making: Validated models provide a sound basis for making critical financial decisions, such as pricing financial instruments, assessing credit risk, or managing investment portfolios. They instill confidence in stakeholders that decisions are based on robust analyses.
- Enhancing Stakeholder Trust: Effective model validation fosters trust among stakeholders, including investors, regulators, and clients, by demonstrating the institution's commitment to integrity and risk management.
- Financial Stability: Inaccurate or flawed models can lead to erroneous decision-making, which may have adverse effects on financial stability. Model validation helps ensure that models produce reliable and consistent results, contributing to the overall stability of the financial system.
Model Validation vs Model Evaluation
Some of the differences between model validation and model evaluation are:
Aspect | Model Validation | Model Evaluation |
Purpose | To assess the accuracy, reliability, and appropriateness of financial models for decision-making. | To assess the performance and effectiveness of a model in achieving its intended objectives. |
Focus | Focuses on confirming that a model appropriately represents real-world phenomena and meets predefined standards. | Focuses on analyzing how well a model performs in terms of its predictive power, accuracy, and efficiency. |
Activities | Backtesting, sensitivity analysis, scenario analysis, comparative analysis, validation metrics, and expert judgment. | Performance metrics analysis, accuracy assessment, efficiency measurement, benchmarking, and user feedback analysis. |
Scope | Covers all aspects of the model development process, including conceptual soundness, implementation, and outcomes analysis. | Primarily examines the outputs and outcomes of the model, often comparing them against predefined criteria or benchmarks. |
Timing | Conducted throughout the model development lifecycle, from initial development to post-implementation monitoring. | Typically conducted after the model has been implemented or deployed, although certain aspects may occur during development. |
Frequently Asked Questions (FAQs)
Common challenges in model validation include data quality issues, model complexity, changes in market conditions, regulatory uncertainty, resource constraints, and the need for ongoing monitoring and updates.
Model validation should be performed regularly, with the frequency depending on the complexity and criticality of the model, changes in market conditions, regulatory requirements, and internal policies.
Not validating financial models can lead to inaccurate predictions, flawed decision-making, regulatory non-compliance, financial losses, reputational damage, and increased operational risks.
To ensure effective model validation, organizations should establish clear validation frameworks, allocate sufficient resources, engage independent validation experts when necessary, prioritize high-risk models, maintain open communication with stakeholders, and continuously monitor and update models as needed.
Recommended Articles
This article has been a guide to what is Model Validation. Here, we explain the techniques, examples, comparison with model evaluation, best practices, & importance. You may also find some useful articles here -