Table Of Contents
What Is Marginal Probability?
Marginal Probability is the possibility of an event occurring without any specific conditions or constraints, given that the event is mutually exclusive, collectively exhaustive, and independent of the other events. It is commonly used in probability theory when dealing with multiple random variables, given that a single event takes place.
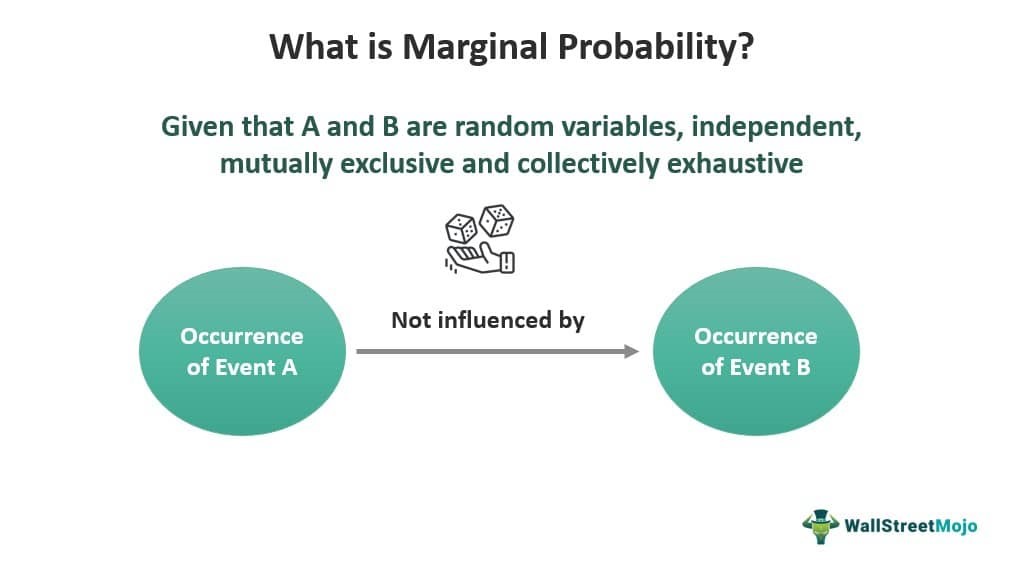
For instance, in the case of two random variables, X and Y, the marginal probability distribution of X, denoted as P(X), represents the probability distribution of X without considering the values of Y. Thus, it allows professionals in various fields, including finance, economics, insurance and marketing to make informed decisions, predict outcomes, and assess risks accurately.
Key Takeaways
- Marginal Probability refers to the chances of a specific event happening without considering any other events. It relies on mutual exclusivity, independence, and collectively exhaustive assumptions.
- It is denoted by p(A) or p(B), respectively, when A and B are two mutually exclusive and independent events.
- Unconditional Probability is applied in economics, statistics, finance, actuarial science, healthcare, and other fields for risk assessment and decision-making.
- While marginal Probability assesses individual events, joint Probability addresses the likelihood of multiple events occurring together, and conditional Probability calculates the chance of an event happening given that another event has occurred.
Marginal Probability Explained
Marginal Probability is used to determine the likelihood of a particular event happening independently without considering other related events. By focusing on individual probabilities in isolation, it provides valuable information for statistical and probabilistic calculations. It is a widely used tool, especially in finance, actuarial science, economics, medical science, and machine learning.
There are a few assumptions associated with marginal probability:
- Mutual Exclusivity: The events being considered must be mutually exclusive, i.e., they must not occur at the same time. If one event happens, the other cannot.
- Collectively Exhaustive: The given set of events must be collectively exhaustive, i.e., these events cover all possible outcomes, and one of the events in the set must occur.
- Independent events: The events should be independent of each other, i.e., the occurrence of an event doesn't influence the probability of the other event occurring.
However, the marginal probability function fails to study the relationships between variables, making it difficult to gain a complete understanding of complex systems. Additionally, the assumption of independence between variables often does not align with real-world scenarios where variables are interconnected, hence narrowing its perspective.
Moreover, calculating unconditional probabilities for a large number of variables can be computationally intensive and impractical. Also, the accuracy of such methods relies on the quality and representation of data, potentially leading to misleading results when dealing with biased or incomplete information.
Formula
The below equation shows how to calculate the marginal probability of two or more independent and mutually exclusive events, A and B:
P(A) = ∑P (A, X) and P(B) = ∑P (B, X)
Where X is the variable.
In other words, we can extract the marginal probability of an individual event A from the joint probability of the random variables A and B. Also, P(A) can never be greater than 1.
Examples
Let us consider the various cases to understand and define marginal probability in a better way:
Example #1
Suppose Paulo is a marketing manager at BM Ltd. To understand the consumer's buying behavior, he calculates the probability of customers buying a product from BM Ltd., regardless of other factors like the competitor's products, price, substitutes, or income level.
The other factors that have no effect on the buying behavior of consumers provide Paulo with a sure-shot number of sales that the company can achieve out of the loyalty of the customers towards their brand. Per his observation, he devises targeted marketing strategies.
This example shows how the concept of marginal probability applies in the field of business, especially in improving sales and marketing strategies.
Example #2
Given below is the table for the probability of annual returns of three different portfolios comprising two stocks each, one from equity and the other from debt class:
Portfolio/Stock | A | B | C | Total |
---|---|---|---|---|
Stock 1 | 27% | 28% | 21% | 76% |
Stock 2 | 7.5% | 8% | 8.5% | 24% |
Total | 34.5% | 36% | 29.5% | 100% |
Hence, the marginal probability of returns from portfolios A, B, and C are as follows:
- P(A) = 34.5%
- P(B) = 36%
- P(C) = 29.5%
Marginal Probability vs Conditional Probability vs Joint Probability
Marginal, conditional, and joint probabilities are the three different forms of probabilities used for drawing statistical inferences in various real-world situations. However, they differ in the following contexts:
Basis | Marginal Probability | Conditional Probability | Joint Probability |
---|---|---|---|
Definition | Marginal probability refers to the probability of a specific event occurring independently without being influenced by other events. | Conditional probability is the likelihood of one event occurring, given that another event has already happened. | A joint probability is the likelihood of two or more events occurring simultaneously. |
Denoted as | P(A) or P(B) | P(A | B) | P(A ∩ B) |
Focuses on | Likelihood of a single event or variable, disregarding the influence of other variables | Calculating the probability of event A occurring under the condition that event B has taken place | Quantifies the probability of two or more events occurring together |
Types of Events | Independent, mutually exclusive and collectively exhaustive | Dependent | Independent |
Calculation | It is derived from the joint probability distribution by summing or integrating the joint probabilities over the other variables. | It is determined using the equation P(A | B) = P(A ∩ B) / P(B), where P(A | B) is event A's conditional probability, given event B has already taken place. | It is calculated by multiplying the probabilities of the individual events if the events are independent. |
Example | On rolling a six-sided die, the marginal probability of rolling a specific number, say 5, is 1/6, assuming a fair die. | It finds the probability of rolling a 3 on a die given that the number rolled is odd. It would be calculated as P(3 | Odd) = P(3 ∩ Odd) / P(Odd). | The joint probability of rolling a 3 on one die, and a 4 on another fair six-sided die is 1/36, assuming the dice rolls are independent events. |