The differences between both the concepts are given as follows:
Table Of Contents
What Is Logit Model?
The logit model is a model used to predict the relation between explanatory variables and ordinal or binary response probabilities. It is used to model variables for a dichotomous outcome and is also referred to as a logistic regression model.
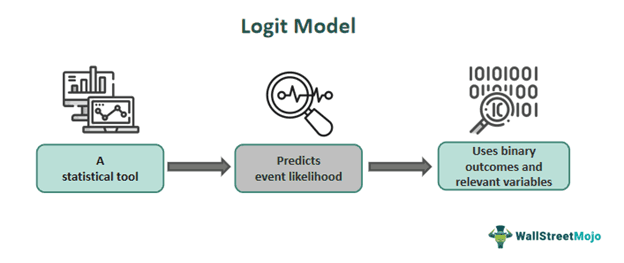
You are free to use this image on your website, templates, etc.. Please provide us with an attribution link.
The biological sciences began to apply logistic regression in the early 1900s. It is now applied in numerous social scientific contexts. Logistic regression is effective when the dependent variable is categorical. It is a type of generalized linear model and is applied to response variables for which standard linear regression is not practical. It is frequently utilized in finance to predict credit risk and evaluate the probability of default for loan applicants.
Key Takeaways
- The Logit Model is used to estimate the probability of binary outcomes based on explanatory variables.
- It finds applications in various fields for predicting events or choices. It could help businesses make informed decisions and improve outcomes.
- The model is a GLM (generalized linear model) that is used when regular linear regression fails.
- It can be applied to churn prediction, illness prediction, and fraud detection. Financial companies can better safeguard their customers by using it to detect anomalies in data that indicate potential fraud.
- Logit and Probit Models are both commonly used, with slight differences in assumptions and interpretation.
Logit Model Explained
Logit model or logistic regression is a statistical measure used to analyze data with a variable of categorical response with two levels. It is used in cases where there are binary dependent variables, where outcomes are either one or zero. The logit regression forces the predicted values or output to take values of either zero or one.
The model is a GLM (generalized linear model) that is used when regular linear regression fails. The response variable in these settings often takes a form that differs from the normal distribution. The error distribution cannot approximate a normal distribution. GLMs are a two-stage modeling approach.
The first step involves specifying the probability distribution for the data's response variable based on the understanding of the data being measured. For zero-one data, the binomial distribution is used. For count data (where positive integers are needed), the Poisson distribution is used. The second step is modeling the distribution parameters using explanatory variables. This approach is especially beneficial for data with non-normal distributions or those with non-negative values. There are different types of models, such as the ordinal logit model, multinomial logit model, nested logit model, and binary logit model.
It can be applied to churn prediction, illness prognosis, and fraud detection. Financial companies can better safeguard their customers by using it to detect anomalies in data that indicate potential fraud. In healthcare, it forecasts the likelihood of diseases in medicine, allowing for preventative intervention. Moreover, it identifies high-performing individuals who are at risk of leaving an organization, which helps identify issue areas and execute retention initiatives to prevent revenue loss.
Examples
Let us look at some examples to understand the concept better.
Example #1
Suppose ABC Limited, a financial institution, uses the Logit Model for fraud detection due to an increase in fraudulent activities. This model handles binary outcomes and estimates the probability of fraud based on relevant variables. Transactions with high probability scores are flagged for further investigation. It leverages historical data and patterns to identify potentially fraudulent transactions, allowing ABC Limited to take proactive measures to prevent financial losses and safeguard customers. Implementation of this model enhances fraud prevention strategies, reduces false outcomes, and improves the efficiency of the fraud detection system.
Example #2
Southeastern Louisiana University, USA, conducted astudy. They used a logit model to investigate the determinants impacting returning and non-returning students. It considered sixteen explanatory variables: academic achievement, credit hours taken, remedial measures, age, gender, Board of Regents core requirements completion, and high school performance and ACT scores. Two logit regression estimates were made: one that included every possible variable and another that included only the significant ones.
According to the findings, there is a greater chance that students are more likely to return for the fall semester (2008) if they have higher overall GPAs, better SE 101 marks (3-course credit first-year success course aimed to increase retention.), or were returning students the previous semester. It was concluded that students with low GPAs may be provided with extra attention and academic advising to increase student retention.
Logit Model Vs. Probit Model
Term | Logit Model | Probit Regression |
---|---|---|
1. Definition | Also called logistic regression models, it is a type of statistical model used to predict the likelihood of an event happening. The models are based on the logistic function (also known as the sigmoid function), which helps analyze situations with two possible outcomes. | Probit Regression measures how the strength of a stimulus relates to the proportion of cases showing a specific response to the stimulus. It's useful in cases of yes-or-no outcomes influenced by certain independent variables, especially in experimental data. |
2. Assumptions | It assumes the errors follow a logistic distribution | It assumes the errors follow a normal distribution |
3. Usage | Not suitable when there are more than two possible outcomes | It becomes difficult to estimate mathematically when there are more than 4 to 5 choices. |
4. Outliers | It is more resistant to outliers because it uses a logistic function | It is more sensitive to outliers |