Table Of Contents
What Is A Log-Linear Model?
A log-linear model is a type of statistical model that is used to analyze relationships between categorical variables. The aim of these models is to provide a statistical framework for exploring and modeling relationships in categorical data.
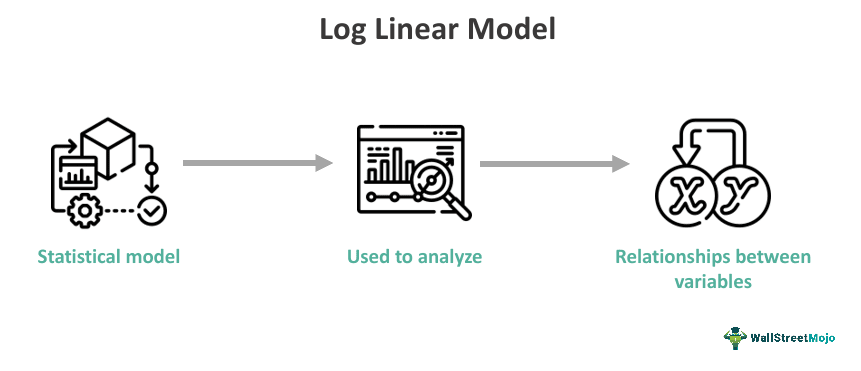
The model enables the analysis of percentage or proportional changes in the dependent variable in response to variations in the independent variables. It facilitates the transformation of data into a linear format, simplifying its analysis through traditional linear regression methods. Moreover, It is a widely applicable and valuable method in fields like economics and epidemiology, where multiplicative relationships are common.
Table of contents
- A log-linear model in econometrics is a mathematical approach that transforms non-linear relationships into linear forms using logarithmic functions. It simplifies the analysis and prediction of data exhibiting exponential growth or decay patterns.
- In diverse fields, such as statistics, economics, finance, market research, environmental science, psychology, and epidemiology, researchers use them to model the relationship between categorical variables in the contingency table.
- While log-linear models address multi-categorical variables, logistic regression handles binary outcomes, and multinomial regression extends logistic regression to multiclass classification.
Log-Linear Model Explained
A log-linear model is a statistical model used to analyze the relationships between categorical variables. It's particularly applicable when working with contingency tables, which display the joint distribution of two or more categorical variables. In situations where the relationships among variables are expected to be multiplicative rather than additive, these models are especially well-suited.
Businesses extensively use it for market research. The model helps analyze consumer preferences and purchasing behavior across different categories and segments. In finance, analysts use them extensively to analyze various financial aspects such as interest rates, stock prices, option pricing, economic forecasting, risk management, and insurance.
The log-linear model interpretation involves understanding the effect of variables on the logarithm of the outcome variable. The coefficients in the model are exponentiated to obtain the multiplicative effect on the original scale.
In the quality control process, these structures are essential for identifying the errors or defects across different batches or categories of manufacturing. Further, these models help tasks like sentiment analysis and topic modeling by extracting patterns and relationships from large text data sets. Furthermore, researchers often express elasticity in terms of the exponential of the estimated coefficients in log-linear models.
In epidemiology, researchers employ it to study disease outbreaks, while in biology and genetics, it is used to gauge environmental patterns and genetic traits. Additionally, psychologists apply these models to study categorical variables related to behavior, cognition, and psychological disorders. Various researchers have improved the log-linear model approach over time, making it a collaborative effort in the field of statistics.
However, some common assumptions of the log-linear model include:
- Independence
- Homogeneity of odds ratio
- No perfect collinearity
- Model appropriateness
- Random sampling
Examples
Moving forward, let us understand how the log-linear model works in the practical scenario with the help of the following examples:
Example #1
Imagine a healthcare researcher conducting a study on patient outcomes after different types of medical treatments. The researcher categorizes patients based on two variables: the type of treatment received (categories: Drug A, Drug B, Placebo) and the severity of the illness (categories: Mild, Moderate, Severe). Hence, the goal is understanding the association between treatment types and illness severity.
Using a log-linear model, the researcher examines the relationships between these categorical variables. The analysis considers the multiplicative nature of the relationships, providing a nuanced understanding of the factors influencing patient outcomes.
Therefore, the results of this model can reveal valuable insights. For instance, if the model indicates a significant interaction term between treatment type and illness severity, it suggests that the effectiveness of treatment might be contingent on the severity of the illness. The findings could inform medical practitioners about the tailored effectiveness of different treatments based on the severity of the condition.
In this example, the model serves as a powerful tool for understanding complex relationships in healthcare data. It facilitates the identification of patterns and associations, providing a basis for evidence-based decision-making in treatment selection and patient care strategies.
Example #2
Imagine a financial analyst researching the stock market. Therefore, it mainly focuses on the relationship between stock price movements and the types of market events that influence them. The analyst categorizes market events into three groups: economic indicators (such as GDP reports), earnings releases, and external shocks (such as geopolitical events). Moreover, analysts are interested in understanding how these events impact movements in stock prices.
By employing a log-linear model, the analyst explores the associations between the categories of market events and the changes in stock prices. The results of this model might reveal insights such as whether certain types of market events have a stronger or weaker impact on stock prices. For instance, if the analysis indicates a significant interaction term between the type of event and the magnitude of stock price changes, it could suggest that the effect of an earnings release on stock prices is contingent on the broader economic context or external shocks.
In this example, the model becomes a valuable tool for financial analysts and investors seeking to understand the complex relationships in the stock market. It provides:
- A framework for uncovering patterns and associations.
- Helping stakeholders make more informed decisions about portfolio management.
- Risk mitigation.
- Overall investment strategies.
Advantages And Disadvantages
Log-linear models offer valuable advantages in terms of interpretability and handling certain types of data. They also come with limitations related to interpretation complexity and assumptions about the underlying data structure. Let us understand these pros and cons below:
Advantages | Disadvantages |
---|---|
Log-linear models allow for better interpretation of relationships between variables in terms of percentage changes to gauge the underlying patterns. | Interpreting results from log-linear models can be challenging for non-technical audiences or individuals unfamiliar with logarithmic transformations. |
Such measures stabilize the variance, making it easier to model relationships in data where the variance is not constant. | Log transformations convert values into a relative scale, losing the information about the absolute values. |
These models meet the assumptions of normality and homoscedasticity (constant variance) better than raw data, making them useful in regression analysis. | It cannot be applied to the data where the input values are zero or negative. |
The model establishes multiplicative relationships, which are additive on the logarithmic scale, simplifying the modeling process for certain types of data. | Sometimes, focusing solely on percentage or proportional changes can ignore important information about the absolute changes in variables, leading to incomplete insights. |
It can help in dealing with positively skewed data, making the data more symmetric and more accessible to analyze. | Moreover, it functions on the assumption of a multiplicative relationship between the variables, and in the absence of this relationship, the model fails to represent the actual underlying pattern. |
Log-Linear Model vs Logistic Regression vs Multinomial Regression
Three distinct statistical techniquesālog-linear models, logistic regression, and multinomial regressionātailor themselves to perform different kinds of data analysis. They hold the following comparisons:
Basis | Log-Linear Model | Logistic Regression | Multinomial Regression |
---|---|---|---|
Definition | A type of statistical model that relates two or more non-linear variables using logarithms. | It is a statistical method used for binary classification tasks where the outcome belongs to either of the classes. | An extension of logistic regression that is used for multiclass classification tasks where the outcome variable can have more than two classes. |
Data Type | Categorical data with multiple categories in contingency table - frequency and count data | Binary or dichotomous outcome data - categorical dependent variable with two levels | These are categorical outcome data - multiclass classification (dependent variables) with more than two unordered categories |
Purpose | Gauge relationships between categorical variables, considering interactions and dependencies | Probability of an outcome for a particular category | Predicts probabilities for multiple categories. |
Application | Used in fields like social sciences and epidemiology to study data with multiple categorical variables | Employed in fields like healthcare and marketing | Applied for predicting customer purchase decisions or email spam classification and for processing tasks like text classification in natural language. |
Outcome | Provides expected frequencies in each cell of the contingency table | Furnishes the probability of an event occurring (any real-valued number ranging from 0 to 1) using the logistic function | Determines probabilities for each category, with the highest one being the predicted outcome |
Frequently Asked Questions (FAQs)
Researchers use linear regression to analyze relationships between continuous variables, while log-linear models are specifically designed for categorical variables. Log-linear models transform relationships into an additive form through the use of logarithms.
Researchers often interpret the results in terms of the logarithmic change in odds or probabilities associated with the variables. Exponentiating the coefficients provides elasticity, representing the percentage change.
This model plays a crucial role in contingency table analysis by allowing researchers to explore and quantify associations between categorical variables and identify patterns in the joint distribution of categories.
Log-linear models assume no perfect collinearity among variables. If multicollinearity is suspected, examining variance inflation factors (VIFs) or considering variable reduction techniques may be necessary.
Recommended Articles
This article has been a guide to what is Log-Linear Model. We explain its examples, comparison with logistics & multinominal regressions, and advantages. You may also find some useful articles here -