Level of Measurement
Table Of Contents
Level of Measurement Definition
The level of measurement in statistics refers to the scale of measurement, which tells one about the manner in which data gets collected and recorded. It serves the purpose of categorizing, ranking, and evenly spacing data having a natural zero by describing information present within data values.
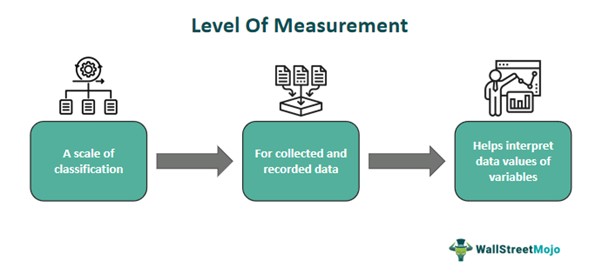
It decides the manner of interpreting correct data using variables. It classifies variables into four levels: nominal, ordinal, interval, and ratio. Each level has implications and significance in describing data sets with a simple to complex hierarchy. For the best outcome, while conducting data analysis plus decision-making, the correct level of measurement has to be there; otherwise, wrong conclusions and inaccurate forecasting may result.
Table of contents
- Level of measurement can be defined as a statistical concept to categorize variables on the basis of the type of data they indicate and their properties.
- Defining the information included inside the data values serves the function of categorizing, sorting, and equally spacing data using a natural zero.
- It has four types - ratio, ordinal, nominal, and interval - each with distinct characteristics and implications, including the presence of a true zero-point, natural ranking, categorical labels, equitable intervals, and limitations in comparison and mathematical operations.
- It plays an important role in statistics by guiding analysis, comparisons, decision-making, and data collection.
Level of Measurement in Statistics Explained
Level of measurement in statistics can be defined as the systematic classification of data into a variety of categories as per their nature, leading to suitable interpretation and appropriate data. It plays a key role in the selection of appropriate statistical analyses, like deciding to use either parametric or non-parametric tests. It acts as a guide to select correct descriptive statistics plus the technique of data visualization while supporting accurate deduction of outcomes.
The level of measurement has certain positives like:
- Summarization and organization of data efficiently
- Guiding in the choosing of correct statistical methods
- Enabling accurate forecasts and meaning contrasts
- Helping the data-analysis-based decision-making process
But it also has certain negatives:
- Inaccurate data analysis of data due to misuse or worn interpretation
- The wrong classification of variables often leads to distorted conclusions
- It becomes challenging to apply the levels for data falling beyond any of the four levels
It finds wide use in the sectors mentioned below:
- Market research – Helps in making informed decisions by categorization of data.
- Healthcare- Facilitates the analysis of patient data to know the effectiveness of medicines.
- Education- Aids in analyzing student performance and learning outcomes
- Social sciences – Helps sociologist to understand human behavior plus social trends
In conclusion, the level of measurement is important for accurate data analysis, suitable statistical methods, and relevant interpretation, which enables researchers and analysts to come to trustworthy findings.
Types
Variables are segregated into four major types due to the measurement level: ratio, ordinal, nominal, and interval. Each of these types has its characteristics, as discussed below:
#1 - Ratio
In the ratio level of measurement, ratio variables have a true zero point besides having all the characteristics of interval variables, denoting the absence of the measured attributes. Meaningful ratios and comparison get their support from it. Height, income, and weight form its examples. Here, the ratio of the same unit of commodity bearing a certain value means one has a value more or less than the other as per the ratio.
#2 - Ordinal
Here, the variables have a certain natural ranking or order. These variables make it possible to compare values, but they do not provide details about their degree of differences. For instance, the Likert scale offers a measurement of preferences or attitudes.
#3 - Nominal
In the nominal level of measurement, the data gets classified as labels or categories as a form of the lowest level of measurement. Data sets containing variables have no quantitative or numerical meaning. One could count or organize the nominal data, but it cannot be used for meaningful comparisons or mathematical operations. Categorical responses, gender, or ethnicity fall under this category.
#4 - Interval
At the level of a measurement interval, the variables present in the data could be compared easily to find their differences due to the presence of inherent equitable intervals amongst the values. However, the true zero point remains absent here. Units for measuring temperatures like Celsius or Fahrenheit have the nature of interval level. Although there can be a fixed difference between the two points here, zero temperature never forms.
Therefore, knowing the significance of various levels of measurement aids in making decisions, identifying suitable statistical metrics, and communicating findings successfully.
Examples
Let us use a few examples to understand the topic.
Example #1
Suppose a school has invited applications for the post of teacher in their high school. Many Americans applied with relevant educational qualifications. They also submitted their high school diploma, bachelor's degree & master’s degree for the post. Hence, the school authority categorizes all the applicants on their educational qualifications. Such categorizations have no numerical significance or inherent order; school authorities created labels based on the different education levels. Such a level is an excellent example of a nominal level.
Here, the school decides to use a scale to assign numerical values like the number of years of education, the number of gaps in education, and the class obtained in the final exam. Hence, using these scales, the school can :
- Compare the educational qualifications of two individuals
- Ascertain the absence of education
- Calculate the record of accomplishment of education as per class obtained
- Segregate applicants based on continuity of education from high school to master’s degree
This illustrates the ratio level of measurement.
Example #2
One real-life example of a level of measurement would be the measurement of income. Income could be measured by usage of the nominal degree of measurement. Here, people get classified into different categories based on income. Hence, various categories like low, high, and middle income exist. One can also measure income as a ratio level of measurement. As a result, income is measured in dollars, which can also be compared using various ratios.
Importance
Due to several reasons, the level of measurement has huge significance in statistics;
- It aids in determining the appropriate technique for use in statistical analysis. As a result, the incorrect statistical methods get eliminated, which may have led to wrong conclusions.
- It allows for conducting meaningful comparisons between variables; otherwise, inferences and valid comparisons may be affected. Ratio and interval-level data provide logical, statistical operations, calculations, and plausible numerical comparisons.
- The level of measurement also aids in the interpretation of data accurately, gives deep insights into the properties and nature of variables, and helps researchers to conclude meaningfully regarding the patterns, relationships, and trends seen in data.
- It helps practitioners and researchers to make informed decisions using data analysis. As a result, they can measure the impacting interventions, evaluate the significance of the findings, and contrast groups.
- It influences the researcher in creating the most suitable measurement scales and collection methods of data to keep it aligned with the statistical analyses and research objectives.
The level of measurement is crucial in statistics as it guides appropriate analyses, enables meaningful comparisons, supports accurate interpretation, aids decision-making, and ensures valid conclusions by influencing data collection and statistical procedures.
Frequently Asked Questions (FAQs)
Age is generally classified as an interval level of measurement, represented by a numerical value reflecting the passage of time. Although the intervals between different ages are uniform, it is important to note that age lacks a true zero point, which means that the absence of age cannot be quantitatively measured.
values to indicate the degree of agreement or disagreement with a statement. Moreover, the scale allows for ranking the responses in order but does not provide information about the exact differences or distances between the response options.
Height is commonly classified as a ratio level of measurement, which is quantified using numerical values that represent the vertical size of an object or person. Moreover, by taking the help of a true zero point, height enables meaningful ratios, comparisons, and calculations; it makes it possible to determine if one height is twice or half the size of another.
Recommended Articles
This has been a guide to the Level Of Measurement and its definition. Here we explain the topic in detail including its examples, types, and importance. You can learn more about it from the following articles –