Latent Variable Model
Last Updated :
-
Blog Author :
Edited by :
Reviewed by :
Table Of Contents
What Is A Latent Variable Model (LVM)?
Latent variable models(LVM) are statistical techniques used to explain and investigate correlations between larger collections of observed variables by incorporating one or more unobserved (latent) variables. These latent variables are often employed in structural equation modeling (SEM), a statistical method for testing intricate theoretical models.
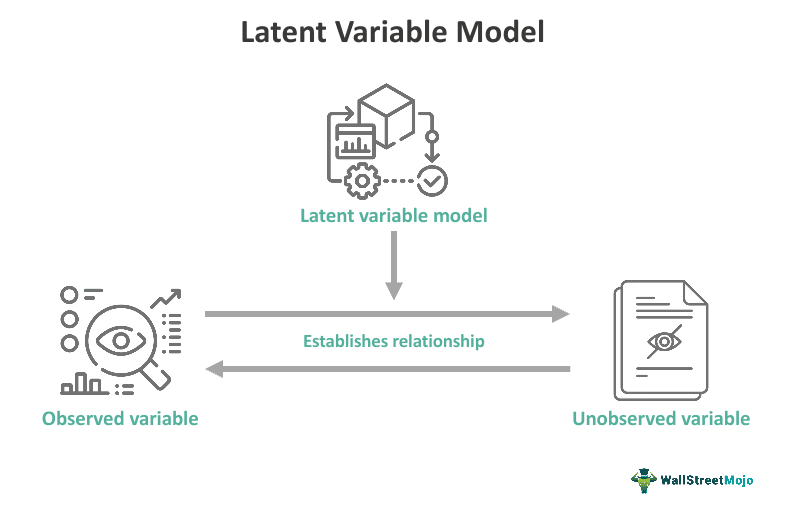
Latent variables, which are unobserved, are often represented through one or more indicator variables that encapsulate their impact. These models are particularly useful for capturing abstract or complex characteristics of a system that are difficult to measure or describe precisely. They play a crucial role in identifying underlying links and patterns that may not be readily apparent. Latent variable models are essential in data analysis, research, and statistics.
Table of contents
- Latent variable models are statistical techniques used to explore and explain correlations among large collections of observed variables by incorporating one or more unobserved (latent) variables.
- They play a crucial role in uncovering underlying links and patterns that may not be readily apparent. These models are important in data analysis, research, and statistics.
- Examples of LVM include the Gaussian process LVM and Bayesian LVM.
- LVMs can be categorized based on various factors, including the nature of response variables (continuous or discrete), the characteristics of the latent variables (discrete or continuous), and the inclusion or exclusion of individual covariates.
Latent Variable Model Explained
A latent variable model (LVM) is a statistical model that encompasses both observed and unobserved variables by establishing connections between statistical properties of observable variables and latent variables. These models are a subset of latent structure models. Different statistical analyses are applied to different variables. For instance, continuous variable distributions are often assumed to be normal, while categorical variable distributions are assumed to be binomial or multinomial.
Typically, latent variables serve two distinct purposes within econometric or statistical models. First, they account for unobserved heterogeneity among subjects, with latent variables representing the effect of these unobservable factors. This approach allows for measurement errors, where manifest variables represent the "disturbed" versions of the "true" outcomes, while latent variables represent the "true" outcomes. The second purpose is to aggregate various measures of similar, directly unobservable traits, making it easier to organize or categorize sample units based on these attributes, as represented by the latent variables.
LVM finds extensive use, particularly when dealing with multilevel, longitudinal panel data and repeated observations. These models are typically categorized based on the nature of the response variables (continuous or discrete), the continuous or discrete character of the latent variables, and the inclusion or exclusion of individual covariates.
Examples
Let us look at a few examples to understand the concept better:
Example #1
Suppose Dan is an investor who wants to understand how well his portfolio of stocks is performing. He can use an LVM to determine what factors affect stock returns, like market risk, company-specific factors, and investor sentiment. Dan can learn about the sources of risk and opportunities for diversification by estimating these hidden factors and their connection to observed stock return.
Example #2
Suppose a company wants to know what consumers prefer for a new product. The company can find hidden factors that drive consumer preferences and group customers based on these factors by using an LVM. They can conduct a survey with questions about different product attributes, like price, quality, and design, to gather data and use the model. This information can help develop products, plan marketing strategies, and target advertising efforts.
Applications
Some of the important applications are listed below:
- In the social sciences and psychology, LVM is frequently employed to explore concepts such as personality traits and intelligence, assessing their impact on factors that are challenging to measure directly.
- They play a crucial role in consumer preference analysis and customer segmentation in marketing research, where latent factors help uncover hidden patterns and trends.
- The financial industry utilizes it to model and forecast asset returns or assess portfolio risk, enhancing decision-making and risk management.
- In healthcare research, these models are instrumental in evaluating the effectiveness of treatments and analyzing patient outcomes, aiding in evidence-based healthcare practices.
- In econometrics, these models are applicable to analyze economic phenomena and estimate unobserved variables, such as economic growth or inflation, providing insights into complex economic systems.
- Additionally, they have applications in machine learning, offering valuable tools for various tasks. Similarly, latent variable models and factor analysis provide a comprehensive analytical framework that spans multiple fields.
Advantages And Disadvantages
Some of the model's advantages and disadvantages are the following:
Advantages
- It helps in the application of path analysis. Using these models across different other model types is typically the idea of path analysis.
- The latent variable model offers the advantage of using fewer features, which helps reduce the dimensionality of an otherwise massive dataset.
- It exhibits flexibility in terms of the types of data that can be analyzed. LVMs and factor analysis can further bring a comprehensive and unified approach to the results.
Disadvantages
- Challenges related to measurement can arise, particularly in the context of generalized latent variable modeling, measurement invariance, and exploratory structural equation modeling.
- The estimation process can be computationally demanding, especially with large datasets.
- Interpreting the meaning of latent variables can be complex.
- The selection and validation of models can be subjective and reliant on the choices made by researchers.
Latent Variable Model vs Structural Equation Model
The differences between the two are as follows:
Latent Variable Model | Structural Equation Model (SEM) |
---|---|
A subset of latent structure models that link statistical properties of observable variables with latent variables. | A statistical analysis technique that examines relationships between variables, combining multiple regression analysis and factor analysis to analyze structural connections between observed variables and latent constructs. |
Estimating and analyzing hidden factors (latent variables) underlying observed variables. | Expands on latent variable modeling by explicitly defining causal relationships between latent and observed variables. |
It can include measurement models and structural models but does not necessarily specify causal relationships. | Incorporates both measurement models (linking latent variables to observed variables) and structural models (relating latent variables to each other). |
Frequently Asked Questions (FAQs)
LVMs in machine learning are statistical models that capture unobservable or hidden variables that impact observed data. They are essential for representing complex relationships and dependencies in the data, enabling tasks such as dimensionality reduction, clustering, and model generation. An example of such a model is the Gaussian process LVM.
A simple LVM assumes the existence of an unobserved variable that explains the observed data. It is a fundamental model that forms the basis for more complex LVMs.
An LVM for quality of life measures hidden factors contributing to an individual's overall well-being and satisfaction. It involves assessing various aspects of quality of life, including physical health, social relationships, and psychological well-being, using observable indicators.
Assumptions of an LVM include unobserved variables that influence the observed data and a known relationship or structure between the observed and latent variables, among other assumptions.
Recommended Articles
This article has been a guide to what is a Latent Variable Model. We explain its examples, applications, advantages, and comparison with structural equation model. You may also find some useful articles here -