Kendall's Tau
Published on :
21 Aug, 2024
Blog Author :
N/A
Edited by :
Ashish Kumar Srivastav
Reviewed by :
Dheeraj Vaidya
Kendall's Tau Definition
Kendall’s Tau or the Kendall rank correlation coefficient refers to a non-parametric test process measuring the direction and strength of the relationship existing between a couple of variables. One can use the statistic to gauge two measured quantity’s ordinal association.
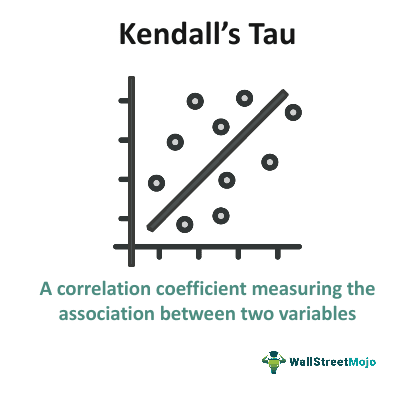
For calculating the Kendall rank correlation coefficient, one must ensure that the data is not normally distributed. Moreover, it is important for the two variables to have an ordinal level of scale. This concept helps measure the rank coefficient, which is the similarity concerning the ordering of data when ranking takes place based on each quantity.
Table of contents
- Kendall’s Tau refers to a statistical technique that measures the relationships between ranked data columns. It is a non-parametric measure.
- This concept has two assumptions. One is that it measures the two variables on an ordinal or a continuous scale.
- There are various benefits offered by this non-parametric statistical method. For example, it is versatile and suits ordinal data. However, it also has limitations. For example, calculating it can be challenging.
- A key difference between Kendall’s Tau and Superman’s Rho is that the latter is less robust and not as efficient as the former.
Kendall's Tau Explained
Kendall’s Tau refers to a non-parametric statistical technique that one can utilize to gauge the direction as well as strength of a couple of variables’ association. It evaluates the similarity in data points ranking between the two variables. This concept is useful for both ordinal and continuous data. It is extremely useful when the data might not have a linear relationship.
One may look at its following features to understand the concept better.
- It is robust to non-linearity and outliers.
- The formula of this non-parametric measure includes data points’ discordant and concordant pairs.
- It gauges the similarity in the ranking order between a couple of variables, where ‘1’ signals or indicates perfect agreement while ‘-1’ and ‘0’ indicate perfect disagreement and no association, respectively.
Although such a test can give negative values ranging from -1 to 0, contrary to a linear graph, a negative relationship does not have much meaning with ranked columns. Hence, one can eliminate the negative sign at the time of interpreting Tau.
Assumptions
The Kendall’s Tau assumptions are as follows:
- The measurement of the two variables must take place on a continuous or an ordinal scale. Examples of continuous variables include intelligence and revision time. One can measure the former in hours while individuals gauge the latter using an intelligence quotient (IQ) score.
- The Kendall rank correlation assumes a monotonic relationship exists between the variables. That said, note that this is not a strict assumption.
Formula
The formula used to compute the Kendall rank correlation coefficient is as follows:
T = (C-D)/(C+D)
Where:
- D is the total number of pairs that are discordant.
- C is the overall concordant pairs
Examples
Let us look at a few Kendall’s Tau examples to understand the concept better.
Example #1
Suppose Company ABC, an online apparel and clothing store, utilizes Kendall’s Tau to understand if there is any relationship between the time taken to deliver the product and the satisfaction of their customers. In this case, delivery time has 3 categories – next day, 3-4 days, and over 4 days. They measure customer satisfaction with regard to the agreement level customers have with the statement, ‘I am fully satisfied with the delivery time,’ where the agreement level has four categories. These categories are ‘strongly agree,’ ‘agree,’ ‘strongly disagree,’ and ‘disagree.’
Example #2
Suppose two portfolio managers rank a couple of their best stocks in ascending order. This table shows the ranks that each portfolio manager assigned the stocks.
Stock | Portfolio Manager 1 | Portfolio Manager 2 |
---|---|---|
A | 1 | 3 |
B | 2 | 1 |
C | 3 | 4 |
D | 4 | 2 |
E | 5 | 6 |
F | 6 | 5 |
Two know if there is any correlation between the selections utilizing Kendall’s Tau, one can do the calculation utilizing the last column only, i.e., the ranking of the second manager.
One needs to look at each of the ranks and note if the values between are larger or smaller than themselves.
Portfolio Manager 2 | |
---|---|
3 | |
1 | - |
4 | + |
2 | - |
6 | + |
5 | + |
As one can find in the above figure, we begin with the first rank, corresponding to the first value or 3. Since 1 is less than 3, it gets a minus. On the other hand, since 4 is larger, it gets a plus. Similarly, we assign – or + to the remaining ranks.
Next, we do the same thing for the next rank corresponding to 1’s value. Hence, we will have + in every row. After that, we’ll proceed with the next three ranks — 4, 2, and 6. After doing so, we can easily compute the overall discordant and concordant pairs. For the latter, we will count all the plus signs. In this example, the total concordant pairs will be 11.
Then, we’ll get all the discordant pairs by counting every minus sign. In this example, the total will be 4.
Therefore, considering C is 11 and D is 4, we can use Kendall’s Tau formula.
T = (C-D)/(C+D)
Or T = 0.47
Advantages And Disadvantages
Let us look at the benefits and limitations of Kendall’s Tau:
Advantages
- It is robust to the outliers. Moreover, this concept works well with normally distributed or skewed data.
- The Kendall rank correlation coefficient is versatile because it does not involve assuming any particular data distribution.
- One can apply this concept to data with ordinal or ranked categories.
- This concept’s interpretation with regard to observing non-agreeable or discordant and agreeable or concordant pairs is direct.
- In a lot of situations, Spearman’s Rho and the Kendall rank correlation coefficients are similar. As a result, they invariable result in identical inferences.
Disadvantages
- This concept might not be able to provide better results than Pearson’s correlation when evaluating linear associations.
- In the case of large data sets, computing the Kendall rank correlation coefficient can be a complicated procedure.
Kendall's Tau vs Spearman's Rho
Individuals new to statistics often find the concepts of Kendall’s Tau and Superman’s Rho confusing. To fully understand them, one must find out how these correlation concepts differ. In that regard, looking at their distinct characteristics is imperative. Hence, the table below highlights the features.
Kendall’s Tau | Spearman’s Rho |
---|---|
It is more efficient and robust. | This concept is less efficient and not as robust. |
Individuals prefer it when there are small samples. | People prefer it when there are large samples. |
This correlation coefficient is usually smaller. | It is usually larger. |
Kendall's Tau vs Pearson's Correlation
Let us look at some key differences between the Kendall rank correlation coefficient and Pearson’s correlation.
- Pearson’s correlation utilizes information concerning the deviation from the mean and the mean. On the other hand, non-parametric correlations, for example, Kendall’s Tau, do not use such information.
- In the case of Pearson’s correlation, the variables’ distribution must be normal and continuous. On the other hand, in the case of the Kendall rank correlation coefficient, the variables can be ordinal or continuous.
Frequently Asked Questions (FAQs)
People often use this concept for correlation on continuous data in case the data comprise outliers. Note that a coefficient of 0.9 or above is very good, according to experts.
This statistical measure can capture the strength of a nonlinear relationship where the conventional or traditional linear association measures do not pass. Its value ranges from -1 to 1.
It is better than Spearman’s Rho because of the following two reasons:
- It is more efficient.
- The concept is more robust.
- It has better statistical properties.
Lastly, unlike Spearman’s Rho, the interpretation of the Kendall correlation coefficient with reference to probabilities of observing agreeable and disagreeable pairs is extremely direct.
The p-value refers to the probability of observing this kind of value solely because of random variation.
Recommended Articles
This article has been a guide to Kendall’s Tau and its definition. We explain its formula, comparison with Spearman's Rho & Pearson's correlation, and examples. You may also find some useful articles here -