Table Of Contents
What Is Kaplan-Meier Estimator?
The Kaplan-Meier estimator is a statistical technique used to estimate the probability of survival over a specific period. It is applicable in the case of time-to-event data. The method aids in calculating the time until a particular event occurs, especially in domains like medical and life sciences.
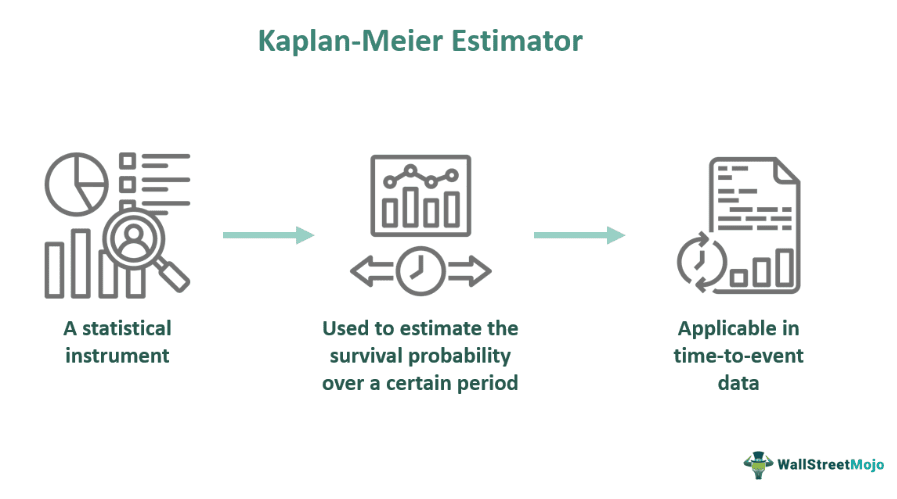
The technique also accommodates incomplete or censored data, where not all individuals or subjects have experienced the event by the end of the study or may have dropped out. The estimator calculates the probability of survival at specific time points by considering the observed survival times and status. Additionally, it offers valuable insights into survival patterns and enables comparisons between different groups or treatments.
Key Takeaways
- The Kaplan-Meier estimator is a statistical method used in survival analysis to evaluate the probability of an event occurring during a particular time frame, especially when applying time-to-event data.
- The estimator is adept at working with censored data. This approach makes efficient use of all the data available and generates reliable survival probabilities even in cases where some data is inadequate.
- However, this approach focuses on one kind of event. It cannot handle analyses in which several types of events are involved.
Kaplan-Meier Estimator Explained
The Kaplan-Meier estimator is a statistical tool employed in survival analysis to estimate the probability of an event occurring over a specific period, especially in the case of time-to-event data. It is extensively used in domains like medical research, social sciences, and engineering, where assessing the time until an event occurs is crucial.
The Kaplan-Meier estimator possesses the ability to handle censored data where not all individuals have experienced the event by the conclusion of the study. It often occurs due to subjects being lost to follow-up or the study ending before all participants encounter the event. The estimator uses the observed survival times and status information to calculate the probability of survival at various time intervals throughout the study.
Assumptions
Some assumptions of Kaplan-Meier Estimator include the following:
- Censoring Uniformity: This assumption suggests that the probability of being censored at any specific time should be consistent across all subjects. It implies that the data is censored randomly and uniformly over time.
- Non-informative Censoring: This assumption states that the reason for censoring is unrelated to the survival prospects of the subjects.
- No Interactions Between Subjects: The estimator assumes that the survival or censoring times of one individual do not impact or relate to the survival or censoring times of others. Each subject's survival time is independent of the others in the study.
Formula
The Kaplan-Meier estimator formula is as follows:
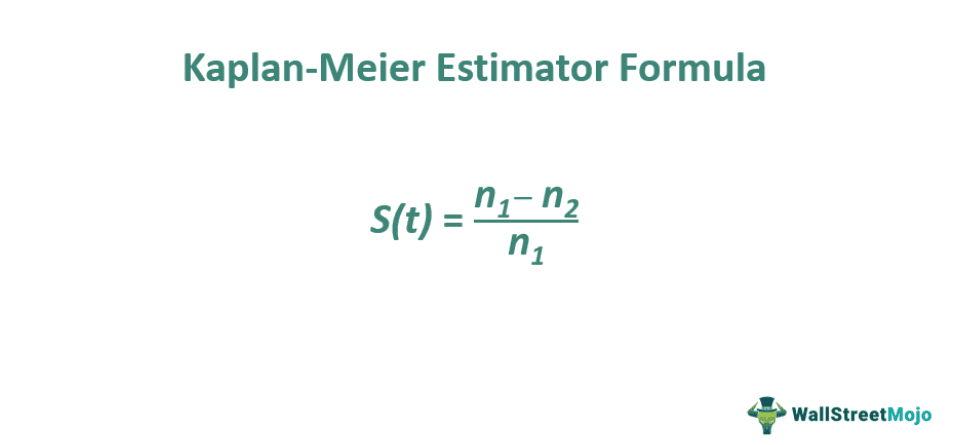
In the formula,
- S(t) is the survival probability at any particular time
- n1 is the number of subjects living at the start
- n2 is the number of subjects who died
Examples
Let us study the following examples to understand this method:
Example #1
Suppose Jenny, an analyst at Good Health Hospital, recorded the survival time of a small group of patients after a treatment. The data was as follows:
- Ryan: 10 months (died)
- Jim: 15 months (censored)
- Jake: 18 months (censored)
- Amy: 20 months (died)
To calculate the estimator, Jenny first arranged the observed times: 10,15,18, 20. Then, she calculated the survival probabilities at each observed time. Then, Jenny applied the Kaplan-Meier estimator formula where S(t)= (n1-n2)/n1. At the start (t=0), all patients were alive, so the survival probability was (4-0)/4 = 4/4 = 1. When t=10, Ryan died, and three patients were remaining. Thus, the survival probability was (4-1)/4 = 3/4. At t=15, Jim censored, maintaining the same survival probability of 3/4. At t=18, Jake censored, which kept the survival probability the same. At=20, Amy died, resulting in 1 patient remaining. It made the survival probability 1/2.
Finally, Jenny plotted the probabilities results in the Kaplan-Meier survival curve. It visually represented the estimated survival probabilities over time in this small patient group after treatment. This is a Kaplan-Meier estimator example.
Example #2
Let us assume David was monitoring the performance of loans in a portfolio to track the default occurrences. The loan of Apex Ltd. defaulted after six months, while that of Legend Software was censored at nine months. Finally, the loan of Creative Salon defaulted after twelve months. Using the estimator, David calculated the survival probabilities at observed times. At the start, all loans were performing, resulting in a survival probability of 1.
When the loan of Apex Ltd. defaulted at six months, two loans remained, creating a survival probability of 2 out of 3. At nine months, the loan of Legend Software was censored, maintaining the same survival probability of 2 out of 3. At twelve months, the second default occurs, leaving one active loan and resulting in a survival probability of 1 out of 1.
Pros And Cons
The pros of the Kaplan-Meier Estimator are as follows:
- The estimator is proficient at handling censored data. It is a common occurrence in studies where not all subjects experience the event in focus or the study ends before all events occur. This method effectively utilizes all available data and provides reliable estimates of survival probabilities even when some information is incomplete.
- The method is specifically designed for estimating survival probabilities over time. It generates a survival curve that visually illustrates the probability of an event not occurring up to a specific time. This enables researchers to understand and compare survival experiences between different groups or treatments.
- This estimator does not assume any specific data distribution. It is robust and does not require assumptions about the shape of the survival function. This makes it highly versatile in various research or practical scenarios.
- It allows for meaningful comparisons between different groups. The technique helps to assess if there are significant differences in survival experiences and provides valuable insights into the effectiveness of treatments or interventions.
The cons of Kaplan-Meier Estimator are:
- The estimator faces limitations in handling time-dependent variables or changing risk factors over time. It assumes that the risk of an event remains constant over time, which might not always be the case in real-life scenarios.
- This method primarily focuses on a single type of event. It does not accommodate analyses where there are multiple types of events occurring.
Kaplan-Meier Estimator vs Nelson-Aalen Estimator
The differences between the two are as follows:
Kaplan-Meier Estimator
- This estimator aids in estimating and visualizing survival probabilities in the presence of censored data, especially in medical and life sciences.
- The estimator is valuable for comparing survival experiences between different groups or treatments.
- It assumes non-informative censoring, implying that the reason for censoring is unrelated to the possibility of experiencing the event. Additionally, it doesn't require any assumptions about the underlying distribution of data.
Nelson-Aalen Estimator
- The Nelson-Aalen estimator helps estimate the cumulative hazard function. It provides the cumulative sum of the hazard rates up to a particular time point, indicating the expected number of events at that time.
- It is advantageous for time-dependent analysis and can handle changes in hazard rates over time.
- It does not assume constant hazard rates and is more flexible in accommodating varying risks.