Table Of Contents
What Is Historical Simulation?
Historical Simulation involves using past data to model financial scenarios, aiming to understand potential outcomes and risks. Its purpose is to provide insights into market behavior, uncovering patterns and correlations while avoiding reliance on theoretical assumptions.
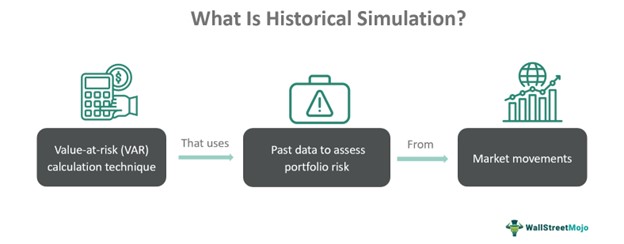
It estimates portfolio or asset losses by simulating future scenarios based on past data and assumes continuity in return patterns, volatilities, and correlations. It is widely used in finance, as it helps capture market complexity and dynamics, offering valuable insights into risk management, particularly in estimating value-at-risk.
Table of Contents
- Historical simulation involves analyzing historical market behavior to simulate future outcomes, providing insights into potential risks faced by financial institutions.
- Utilizes accurate data, captures unexpected events but relies on historical patterns, and may not predict future changes accurately.
- Monte Carlo simulation models risk factors using random number generation, while historical simulation uses actual historical data. Monte Carlo predicts outcomes based on statistical properties, while historical simulation directly simulates past events.
Historical Simulation Method ExplainedÂ
Historical simulation helps assess potential losses of a portfolio or asset based on past data. The concept revolves around projecting future outcomes based on historical patterns. It assumes continuity in the historical trends of returns, volatilities, and correlations, extending them into the future. This approach enables financial institutions to grasp the intricate dynamics of the market by leveraging actual historical data, rendering it a favored technique for estimating value-at-risk.
Now, delving into the intricacies of this method, financial entities undertake a systematic process:
- Data Collection: Initially, relevant historical data about the portfolio or asset is gathered, encompassing metrics like daily or weekly returns alongside pertinent variables such as volatilities, correlations, or interest rates.
- Sorting The Data: Subsequently, the collected data is organized chronologically, commencing with the oldest observation and culminating with the most recent. This chronological arrangement ensures the simulation adequately reflects the temporal evolution of the market.
- Selecting The Time Horizon: Financial institutions determine the period across which they aim to evaluate potential losses, which could span from a few days to several months, contingent upon specific requirements and objectives.
- Calculating Portfolio Losses: It helps in utilizing the sorted data and the designated time horizon, losses for the portfolio are computed for each observation. This involves applying portfolio weights to historical returns and aggregating the results.
- Determining Value-at-Risk: Following the computation of portfolio losses across observations, financial institutions ascertain the value-at-risk by identifying the appropriate percentile within the loss distribution. For instance, opting for the 95th percentile entails estimating the potential loss, which is unlikely to be surpassed with a 95% confidence level.
Examples
Let us look at some examples to understand the concept better -
Example #1
An investment firm aims to assess the value-at-risk for a diversified portfolio of bonds over a one-month time horizon. They gather historical data on the weekly yields of various bond types, including government, corporate, and municipal bonds, spanning the past five years.
After arranging the data chronologically and specifying the one-month time horizon, they proceed to compute portfolio losses for each observation. This involves weighting the historical yields of each bond type according to their allocation in the portfolio.
Subsequently, the firm determines the value-at-risk by selecting a percentile from the loss distribution, such as the 95th percentile. This percentile indicates the potential loss that is not expected to be exceeded with 95% confidence over one month.
By employing this method in this manner, the investment firm gains insights into the potential downside risks associated with its bond portfolio. However, it is essential to acknowledge that this method operates under the assumption of continuity in past market trends, and actual outcomes may deviate due to unforeseen events or changes in economic conditions. Thus, while this method provides valuable insights, it's crucial to complement it with other risk management techniques and remain vigilant to evolving market dynamics.
Example #2
Imagine a commodity trading firm seeking to evaluate the value-at-risk for a portfolio of agricultural commodities over a three-month time horizon. They compile historical data on the daily price movements of various commodities, such as wheat, corn, and soybeans, spanning the past decade.
After organizing the data in chronological order and specifying the three-month time horizon, they proceed to compute portfolio losses for each observation. This entails applying the portfolio weights to the historical price changes of each commodity.
Following this, the firm determines the value-at-risk by selecting a percentile from the loss distribution, perhaps opting for the 90th percentile. This percentile signifies the potential loss expected not to be exceeded with 90% confidence over the three months.
By employing historical simulation in this context, the commodity trading firm gains insights into the potential downside risks associated with its commodity portfolio. However, they must acknowledge that historical simulation relies on the assumption of continuity in past market behavior. The actual outcomes may diverge due to unforeseen events or shifts in supply and demand dynamics.
Advantages And Disadvantages
The advantages and disadvantages of historical simulation are as follows -
Advantages-
- It utilizes accurate historical data, making it reflective of actual market conditions and behaviors.
- By drawing from historical data, it can capture unexpected events and correlations that theoretical models may overlook.
- Unlike some other methods, it doesn't rely on specific assumptions about the underlying probability distributions of variables.
- It's relatively straightforward to explain how this method works, making it accessible to stakeholders and decision-makers.
Disadvantages-
- It assumes that past patterns will continue, potentially leading to inaccurate predictions if market conditions change significantly.
- The accuracy of this method depends on the quality and relevance of historical data, which may only sometimes be comprehensive or fully representative.
- It may need help to accurately predict extreme or rare events that have yet to occur in the historical data. This might lead to underestimation of tail risks.
- Historical simulation models may need to adapt better to changing market dynamics, technological advancements, or regulatory shifts, potentially leading to outdated risk assessments.
Historical Simulation vs Monte Carlo Simulation
The differences between historical simulation and Monte Carlo simulation are as follows -
Historical Simulation | Monte Carlo Simulation |
---|---|
It relies on historical data to simulate potential outcomes. It assumes that past performance serves as a reliable indicator of future performance. | Monte Carlo simulation operates by modeling the distribution of risk factors using a random number generator, without relying on historical data. |
This method directly employs actual past figures or variables that have been previously experienced. Each simulation within a historical simulation involves utilizing specific historical values of random variables to calculate the value of the project or asset. | This method creates a computer-based model that encompasses all relevant random variables affecting the performance of a financial project. It includes any interrelationships, interdependencies, and serial correlations between these variables. |
Frequently Asked Questions (FAQs)
Model building entails creating mathematical or statistical frameworks to represent financial markets, incorporating assumptions and theoretical relationships. Conversely, historical simulation directly analyzes past data to estimate future risks, bypassing theoretical models.
It is a risk management technique that enhances the accuracy of traditional methods by assigning different weights to historical observations. This method acknowledges that not all historical data points are equally relevant or representative of future market conditions.
No, this method is not deterministic. While it utilizes past data to simulate potential outcomes, it does not rely on predetermined or fixed parameters. Instead, it involves analyzing historical market behavior to estimate future risks. Still, the actual outcomes can vary due to the dynamic nature of financial markets and the inclusion of random factors. Therefore, historical simulation provides a probabilistic approach rather than a deterministic one in assessing risk.
Recommended Articles
This article has been a guide to what is Historical Simulation. Here, we compare it with Monte Carlo simulation, examples, advantages, and disadvantages. You may also find some useful articles here -