Table Of Contents
What Are Heteroskedasticity-Robust Standard Errors?
Heteroskedasticity-Robust Standard Errors refer to adjusted standard errors used in statistical models when heteroskedasticity is present. The purpose is to correct for the varying variance of errors across different levels of the independent variables, which helps mitigate the biases and inefficiencies.
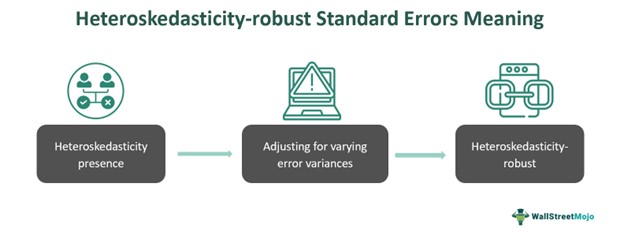
They fix a problem in regression where the error terms have changing variability. This leads to inaccurate estimates of how reliable the results are. By accounting for this, robust standard errors give a more accurate picture of how certain the findings are, even when the error terms are uneven.
Key Takeaways
- Heteroskedasticity-robust standard errors adjust for varying error variance in regression models, providing reliable estimates of parameter uncertainty even when the assumption of constant error variance is violated.
- Homoscedasticity refers to consistent error variance across independent variable values, while heteroskedasticity denotes varying error variance across those values, potentially distorting regression parameter estimates.
- It offers a robust estimation of parameter uncertainties, ensuring the validity of statistical inference in the presence of varying error variance.
Heteroskedasticity-Robust Standard Errors Explained
The heteroskedasticity-robust standard error refers to the varying variance of a dependent variable, indicating unequal variability across different values of an independent variable. This phenomenon is observable in financial data, such as heightened stock price volatility during economic uncertainty. Despite posing challenges, heteroskedasticity offers insights into variable relationships, aiding economists in comprehending how changes in one variable influence the variability of another.
In the realm of regression analysis, the assumption of constant error term variance, known as homoscedasticity, is often made. However, real-world scenarios frequently deviate from this assumption, resulting in heteroskedasticity. Heteroskedasticity manifests when the variability of error terms varies across different levels of independent variables, potentially introducing bias into coefficient and standard error estimations.
To tackle this challenge, researchers have devised methods to obtain robust standard errors that account for heteroskedasticity. Among these approaches, het-robust standard errors stand out as a popular choice. They provide consistent estimates of standard errors even in the presence of heteroskedasticity. This section delves into the intricacies of executing heteroskedasticity-robust standard errors in regression analysis, exploring diverse options and offering insights from various perspectives.
One common test for heteroskedasticity is the Breusch-Pagan Chi-square Test. This test examines whether the squared residuals from a regression model are related to the independent variables. If significant relationships are found, it indicates the presence of heteroskedasticity.
Two common methods to address heteroskedasticity are:
- Robust Standard Errors: Adjusting standard errors to account for heteroskedasticity, often done automatically in statistical software.
- Generalized Least Squares (GLS): Modifying the regression equation to eliminate heteroskedasticity assumptions, followed by estimation under the new conditions.
Examples
Let us look at the heteroskedasticity robust error method examples to understand the concept better -
Example #1
Let us consider a hypothetical example involving the relationship between income and expenditure. In a homoskedastic scenario, it is assumed that the variance of expenditure remains constant across all levels of income. However, heteroskedasticity might occur if, for instance, at lower income levels, people tend to have more erratic spending patterns due to budget constraints or financial instability, leading to higher variability in expenditure. Conversely, at higher income levels, individuals may have more stable spending habits, resulting in lower variability in expenditure. This difference in expenditure variability across income levels would represent heteroskedasticity in the relationship between income and expenditure.
Example #2
Suppose a researcher is studying the relationship between study hours and exam scores. They use a regression model and find a positive correlation, meaning more study hours are associated with higher scores. However, upon closer inspection, they noticed the spread of exam scores for students with similar study hours is uneven. This unequal spread, called heteroskedasticity, can make the standard errors (a measure of the coefficient's reliability) unreliable. To address this, the researcher uses robust standard errors. These account for the changing spread, providing a more accurate picture of how confident they can be in the estimated relationship between study hours and exam scores despite the unevenness in the data.
Advantages
The advantages of heteroskedasticity robust standard error are as follows-
- Enhanced Robustness: Heteroskedasticity-robust standard errors offer increased robustness by accommodating varying error variances, ensuring that statistical inference remains valid even in the presence of heteroskedasticity. This robustness helps mitigate the risk of biased parameter estimates and unreliable hypothesis tests.
- Consistent Inference: Despite potential misspecification or violation of assumptions, it facilitate consistent inference by providing reliable estimates of parameters and their associated standard errors. This consistency ensures that the results of statistical tests remain valid and trustworthy.
- Improved Efficiency under Heteroskedasticity: In cases where heteroskedasticity is present, heteroskedasticity-robust standard errors offer improved efficiency compared to traditional standard errors. By adjusting for the varying variance of errors across different levels of independent variables, they provide more accurate estimates of parameters and their associated uncertainties.
- Straightforward Approach for Addressing Varying Error Variances: Heteroskedasticity-robust standard errors offer a straightforward and practical solution for addressing the challenge of varying error variances in regression models. They do not require the specification of a specific heteroskedasticity structure, making them easier to implement and interpret compared to some alternative methods like Weighted Least Squares (WLS) or Feasible Generalized Least Squares (FGLS).