Generalized Method of Moments
Published on :
21 Aug, 2024
Blog Author :
Wallstreetmojo Team
Edited by :
Ashish Kumar Srivastav
Reviewed by :
Dheeraj Vaidya
What Is the Generalized Method Of Moments (GMM)?
The generalized method of moments (GMM) is a statistical technique that employs observed economic data and population moment conditions to estimate unknown parameters of an economic model. This method is particularly valuable when more moments are available than estimable parameters.
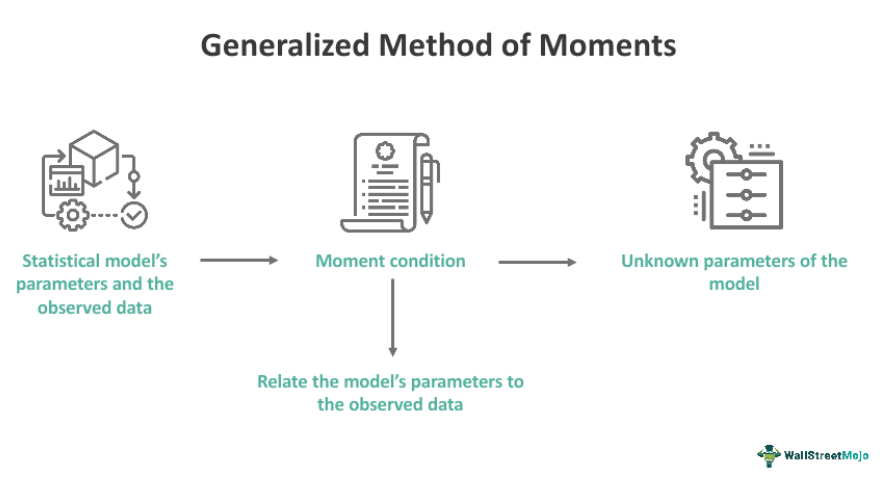
The concept of moments plays a crucial role in describing population features, and the generalized method of moments was developed for econometric applications involving complex models and data structures. GMM may encounter finite-sample problems if excessive moment conditions are introduced. It is widely used because GMM estimators often exhibit greater efficiency than traditional methods, especially in cases where auxiliary assumptions like homoskedasticity fail.
Table of contents
- The generalized method of moments (GMM) is a statistical technique that utilizes observed economic data and population moment conditions to estimate unknown parameters of an economic model.
- GMM establishes regularity conditions for various "moment conditions" by using central limit theorems and laws of large numbers, leading to a broadly applicable class of estimators.
- The generalized method of moments econometrics relies on more general characteristics of a dataset's population, resulting in fewer assumptions than other methods.
- GMM finds applications in various fields, including finance. Its flexibility allows it to handle complex model specifications, making it particularly useful when traditional assumptions are violated.
Generalized Method Of Moments Explained
The generalized method of moments (GMM) is a method in statistics that finds model parameters by matching data patterns with theoretical expectations, often used in economics. GMM estimation, formalized by Hansen in 1982, is widely employed for estimating models in economics and finance. It does not require complete knowledge of the data distribution but only relies on specified moments derived from the underlying model. In cases where moment conditions outnumber a dataset's model parameters, GMM estimation provides a straightforward way to assess the proposed model's specification, a unique feature of GMM estimation.
GMM is an estimation procedure for situations where the investigator lacks complete knowledge of the data's probability distribution to specify an econometric model. It only requires specifying a set of moment conditions derived from the model's assumptions. This method is particularly useful for economists dealing with various orthogonality conditions or moments from their economic models.
This statistical method extends the classical setup by addressing the issue of multiple moment conditions, providing information about unknown parameters and permitting parameter estimation using quantities other than sample moments. By applying central limit theorems and laws of large numbers, GMM establishes regularity conditions for various moment conditions, resulting in a broadly applicable class of estimators.
GMM shares similarities with maximum likelihood (ML) but relies on assumptions about specific moments of random variables, making it more robust than ML, albeit at the expense of efficiency. GMM allows for more moment conditions than parameters, making it more efficient. When there are more moment conditions than parameters, the estimator is considered over-identified, and GMM can efficiently combine these moment conditions.
Assumptions
The generalized method of moments econometrics builds on more general characteristics of a data set's population, resulting in fewer assumptions. One such assumption is that the moment conditions may stem from the assumption of exogeneity in a data set's instrumental variables. Another assumption is that there are enough moment conditions, and among those, there are at least "p" independent ones. It is important to note that the consistency of results depends on the assumption that parameter vectors are identified by combinations of moments taken. Other assumptions may include sample moments converging in probability and sample moments adhering to a central limit theorem.
Examples
Let us look at some examples to gain better insights into the generalized method of moments:
Example #1
Suppose Dan, a financial economist, employs the generalized method of moments to estimate asset pricing model parameters. He aims to uncover the relationships between stock returns and various factors, including interest rates, market volatility, and company-specific indicators. GMM is Dan's method of choice due to its adaptability and capability to handle complex models. To achieve this, Dan assembles historical data on stock returns, interest rates, market volatility, and financial indicators for a sample of companies. GMM's ability to handle more moment conditions than necessary parameters proves invaluable in obtaining efficient estimates, even in situations with limited data.
Example #2
Ravi Jagannathan, Georgios Skoulakis, and Zhenyu Wang conducted a study on GMM's applications in finance. The findings demonstrated the utilization of methods for asset pricing, and investors can apply this knowledge to their investments.
The general summary is as follows:
GMM is a relevant tool in financial applications, particularly in asset pricing. The study found that in most asset-pricing models, the value of a financial claim equals the expected discounted present value of its future pay-offs. However, since investors have access to a larger body of knowledge than economists do, the value calculated might not match the market price.
Using moment conditions, which are typically larger than the total number of model parameters, GMM can be useful to estimate model parameters. Using the GMM statistic, overidentifying restrictions offers a natural test for model misspecification. In finance, continuous-time models are important, particularly when utilizing arbitrage arguments to value contingent claims. They consider the prices of primitive assets to have an exogenous stochastic performance. The study thus concludes that GMM estimates can serve as baselines for more effective estimation techniques.
Applications In Finance
GMM has enabled the econometric evaluation of asset-pricing models under realistic assumptions about the stochastic process governing exogenous variable evolution. Understanding why various assets yield different returns on average and at different times is the main goal of finance research. To solve these problems, several asset pricing models have been put forth.
The stochastic process regulating information arrival in financial markets, the type of transactional technology for transferring real and financial claims amongst economic players, and assumptions about investor profiles all contribute to their differences. Using observable variables and model parameters, each model describes the predicted returns on the assets.
Its application is important in asset pricing models, panel data analysis, risk management, market microstructure, event studies, and financial econometrics. System generalized method of moments, an extension of panel data models, also finds a use case in finance. GMM helps researchers and portfolio managers examine the relationships between risk factors, asset returns, and premiums.
Generalized Method Of Moments vs Maximum Likelihood
The distinctions between the Generalized Method of Moments (GMM) and Maximum Likelihood Estimation (MLE) are as follows:
#1 - Concept
GMM: GMM is a method for constructing estimators. It leverages observed economic data and population moment conditions to estimate unknown parameters of economic models.
MLE: MLE involves estimating the parameters of a statistical model by identifying the values that maximize the likelihood of the given data.
#2 - Adaptability
GMM: GMM is famous for its flexibility and ability to work with models that make fewer assumptions. It's particularly useful when strong assumptions about the data's distribution may not hold.
MLE: MLE relies on more specific assumptions about the probability distribution of the data. It works best when the assumed distribution closely matches the true data-generating process.
#3 - Probability
GMM: In GMM, the estimation process involves calculating the maximum probability of categorization by utilizing the product of Gaussian distributions. This approach is useful in situations where the data may not conform to a single specific distribution.
MLE: MLE seeks to identify the distribution with the highest likelihood of generating the observed data. While it can involve Gaussian distributions, it also accommodates other distribution functions as necessary to model the data accurately.
Frequently Asked Questions (FAQs)
Researchers employ the generalized method of moments (GMM) when traditional estimation methods prove inadequate for analysis. This situation typically arises when assumptions are violated or when the number of moment conditions surpasses the known parameters in a model.
GMM panel data, short for the generalized method of moments panel data, applies when researchers collect observations over time for multiple individuals or entities. It encompasses the "system generalized method of moments", an extension of panel data models.
The Generalized Method of Moments in linear regression involves using GMM estimation techniques to estimate parameters in linear regression models. It allows for the modeling of relationships between one or more explanatory variables and a scalar response.
The primary distinction between GMM and OLS (Ordinary Least Squares) lies in their assumptions and adaptability. OLS makes more specific assumptions, typically assuming a particular distribution in linear regression. In contrast, GMM is more flexible and suitable for models with fewer restrictive assumptions.
Recommended Articles
This article has been a guide to what is the Generalized Method of Moments. We explain its examples, assumptions, and comparison with maximum likelihood. You may also find some useful articles here -