Table Of Contents
What Is Fornell-Larcker Criterion?
The Fornell-Larcker criterion is a discriminant validity test that identifies the degree to which a construct considerably differs from the other constructs in a structural equation modeling (SEM). Hence, it confirms that every construct has its identity, indicating the reliability of the overall model in deterring multicollinearity issues.
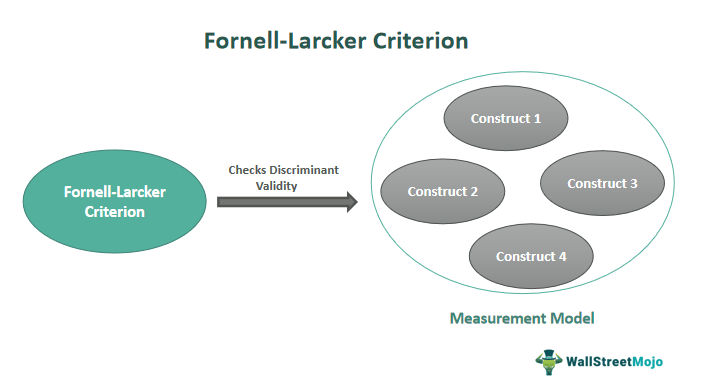
The traditional method involves comparing the square root of a construct's average variance extracted (AVE) with its correlations with other constructs. If the former is higher than the latter, there is a clear distinction between the constructs, and they are said to measure varied concepts.
Key Takeaways
- The Fornell-Larcker criterion is a Structural Equation Modelling (SEM) technique that determines a model's discriminant and convergent validity by verifying that a construct has a separate identity and differs from the other constructs in an SEM.
- If the construct's square-rooted average variance extracted (AVE) is more than its correlation with other constructs. There is a visible difference between the variables, which shows that the chosen model is reliable.
- It is a valid approach for the variance-based SEMs; however, being inefficient in establishing discriminant validity for all types of models, the HeteroTrait-MonoTrait (HTMT) criterion gained popularity.
Fornell-Larcker Criterion Explained
The Fornell-Larcker Criterion is a statistical test portraying discriminant validity of different constructs in a model, ensuring their independence. It was proposed in 1981 by Claes Fornell and David F. Larcker. The idea was to find the discriminant validity in an SEM by comparing each construct's average variance extracted (AVE) square root with the correlation between that construct and others in the model. The difference is validated if the former is greater than the latter. This method is implemented in diverse fields like finance, marketing research, human resources, psychology, education, and healthcare to ensure that the measurement model is reliable and will provide the correct outcome.
However, the discriminant validity Fornell-Larcker criterion was criticized by many statisticians for its inability to properly articulate the discriminant validity for all kinds of Structural Equation Models (SEMs). Therefore, an alternative approach was introduced, the HeteroTrait-MonoTrait (HTMT) ratio of correlations derived from the multitrait-multimethod matrix. It was superior and more accurate than the previous techniques.
Steps
The discriminant validity Fornell-Larcker criterion is a method to confirm the reliability of a structural evaluation model. Below are the steps to implement the Fornell-Larcker criterion technique:
- Compute Construct Averages: The foremost step is to determine the average for each construct using the provided data or software.
- Determine Construct Correlations: Next, the researcher must utilize the constructed averages to find Pearson correlations between different constructs.
- Latent Variables Correlation Matrix: Then comes the formation of a table or matrix representing all the correlations between the various constructs.
- Average Variance Extracted (AVE): The researcher has to extract or evaluate the average variance for all the variables.
- Square Root of AVE: In this step, the researcher computes the square root of all the average variances extracted.
- Update Diagonal Entries: Now, it is time to substitute the diagonal entries (all correlations with value 1) in the previously created table with the square roots of their AVE values.
- Validate Discriminant Validity: In this step, the researcher verifies whether the correlation between each construct and its counterparts is less than the square root of its AVE.
- Interpretation: Finally, the discriminant validity is established if the overall criteria are satisfied. Otherwise, the researcher needs to reconsider the measurement model.
Examples
Now, let us find out how the Fornell-Larcker criterion of gauging the discriminant validity works in a real-world scenario using the following examples:
Example #1
Suppose the manager wants to analyze the relationship between four factors: capital acquisition, investment in fixed assets, productivity, and sales revenue. To ascertain the discriminant validity between the constructs based on their average variance extracted, he used the discriminant validity Fornell-Larcker criterion. Given below is the matrix showing the latent variable correlations:
Key Sections | Capital Acquisition | Fixed Asset Investment | Productivity | Sales Revenue |
---|---|---|---|---|
Capital Acquisition | 1 | 0 | 0 | 0 |
Fixed Asset Investment | 0.815 | 1 | 0 | 0 |
Productivity | 0.779 | 0.802 | 1 | 0 |
Sales Revenue | 0.795 | 0.745 | 0.782 | 1 |
Given below are the Average Variance Extracted for the given constructs:
Construct | Average Variance Extracted (AVE) | Square Root of Average Variance Extracted |
---|---|---|
Capital Acquisition | 0.809 | 0.899 |
Fixed Asset Investment | 0.783 | 0.884 |
Productivity | 0.798 | 0.893 |
Sales Revenue | 0.754 | 0.868 |
Replacing the vertical values in the variables correlation matrix with the square roots of AVE for the constructs:
Key Sections | Capital Acquisition | Fixed Asset Investment | Productivity | Sales Revenue |
---|---|---|---|---|
Capital Acquisition | 0.899 | 0 | 0 | 0 |
Fixed Asset Investment | 0.815 | 0.884 | 0 | 0 |
Productivity | 0.779 | 0.802 | 0.893 | 0 |
Sales Revenue | 0.795 | 0.745 | 0.782 | 0.868 |
Result: In the above matrix, we can identify that when the square root of capital acquisition's AVE, I.e., 0.899, is compared to its correlation with the other constructs, it is more significant and greater for all the variables. Thus, it indicates a clear distinction, confirming the discriminant validity between the constructs.
Example #2
Suppose the regional sales head of ABC Ltd. wants to correlate the three variables, I.e., the sales manager's experience, sales team efficiency, and lead generation. However, she wants to ensure discriminant validity among these variables and employs the Fornell-Larcker criterion to verify it. In her analysis, she found that the constructs do represent a significant difference. Thus, she gains confidence in the reliability of her performance evaluation system.