Table Of Contents
What Is A Factorial Design?
Factorial design is a statistical experimental design used to investigate the effects of two or more independent variables (factors) on a dependent variable. By manipulating the levels of the characteristics and measuring the resulting impact on the dependent variable, researchers can identify each element's unique contributions and their combined or interactive effects.
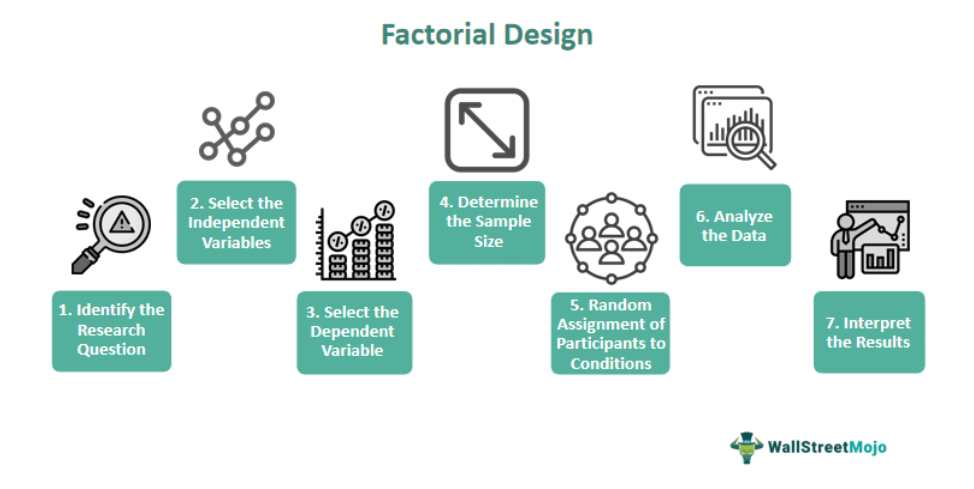
These are beneficial when investigating interactions between variables. They allow researchers to explore how one factor's effects may depend on another element's levels. This can provide valuable insights into the underlying mechanisms. It further drives the observed impacts and helps identify potential moderators or mediators of the relationship between variables.
Key Takeaways
- Factorial designs allow researchers to investigate the impacts of independent variables on a dependent variable in a single experiment. This can save time and resources and provide a nuanced understanding of the relationships between variables.
- It can identify the main effects and interaction effects between independent variables. This provides insights into the unique contributions of each variable and how they interact with one another.
- It can increase the statistical power of a study by manipulating multiple independent variables. This improves the likelihood of detecting meaningful effects.
Factorial Design Explained
Factorial designs are robust and widely used experimental designs in research. The origins of factorial designs trace back to the 20th century. This was with the development of analysis of variance (ANOVA) and the work of Ronald A. Fisher.
These are relevant because they allow researchers to investigate the effects of factors on a dependent variable in a controlled setting. By manipulating the levels of the elements, researchers can determine the unique contributions of each component, as well as any interactive effects that may exist between them. This information helps develop interventions or treatments tailored to a population's needs or context.
For example, a 2x2 factorial design would involve testing two factors, each with two levels. This would result in four different conditions (2x2=4), representing all possible combinations of the levels of the two factors. By manipulating the stories of the factors in each state and measuring the effect on the dependent variable, researchers can determine the main results of each element and any interaction effects between them.
Types
It can be classified into several types. Based on the number of independent variables (factors) and levels used in the experiment. Some common types of it include:
- 2x2 factorial design: It involves two independent variables, each with two levels. It is popular in psychological research to investigate the effects of two factors on behavior or outcome.
- 3x3 factorial design: It involves three independent variables, each with three levels. It helps investigate the effects of multiple factors on behavior or outcome and can be particularly useful in medical research.
- Mixed factorial design: This design involves at least one independent variable manipulated within subjects (i.e., each participant experiences all levels of the variable). And at least one independent variable between subjects (i.e., each participant experiences only one level of the variable).
- Nested factorial design: This design involves one independent variable that is in alignment with another independent variable. For instance, a study on different types of therapy for depression might have one independent variable that represents the type of therapy (cognitive-behavioral therapy, psychoanalytic therapy, etc.) and another independent variable that represents the therapist administering the treatment.
- Fractional factorial design: This design involves testing only a subset of possible combinations of levels of the independent variables. This can be useful when resources are limited or when trying all possible combinations would be impractical.
Examples
Let us understand it better with the help of some examples:
Example #1
Suppose a study on the effects of different types of online learning environments and study strategies on academic performance in college students is carried out. The study could use a 2x2 factorial design, with two independent variables (learning environment and study strategy) and two levels of each independent variable.
The learning environments could be synchronous online learning (i.e., live classes with real-time interaction), asynchronous online learning (i.e., pre-recorded lessons with discussion boards), or a combination. The study strategies are self-regulated learning (i.e., self-paced and self-directed study), collaborative learning (i.e., group work and peer feedback), or a combination.
Participants would be assigned groups: synchronous learning with self-regulated study, asynchronous understanding with collaborative study, synchronous and asynchronous learning with self-regulated study, or synchronous and asynchronous learning with collaborative research. The dependent variable would be the participants' academic performance, measured by their grades in a specific course or course.
By manipulating the levels of the learning environment and study strategy and measuring their combined and individual effects on academic performance, this study could provide valuable insights into the most effective approaches to online learning for college students.
Example #2
Recently a study was published in The Lancet medical journal in 2021. The study investigated the effects of different COVID-19 vaccines on the immune response in older adults at higher risk of illness and death from COVID-19.
The study used a 2x2 factorial design. Participants were assigned to groups based on two independent variables: vaccine type (Pfizer-BioNTech or Oxford-AstraZeneca) and dosing interval (3 or 12 weeks between doses). The dependent variable was the level of antibodies against the SARS-CoV-2 virus in the participants' blood.
The study results showed that the Pfizer-BioNTech vaccine produced a higher antibody response than the Oxford-AstraZeneca vaccine, regardless of the dosing interval. However, the dosing break significantly affected the antibody response, with a longer gap between doses resulting in higher levels of antibodies in both vaccine groups.
Advantages And Disadvantages
The advantages are as follows:
- Ability to investigate multiple factors: These allow researchers to investigate the effects of various independent on a dependent variable in a single experiment, which can save time and resources.
- Identification of main effects and interactions: These enable researchers to identify the main products of each independent variable and any interaction effects between them, providing a more nuanced understanding of the relationships between variables.
- Increased statistical power: By manipulating multiple independent variables, factorial designs can increase the statistical power of a study and improve the likelihood of detecting meaningful effects.
- Flexibility: These adapt to various research questions and uses in multiple fields, including psychology, education, medicine, and engineering.
The disadvantages are as follows:
- Increased complexity: Using multiple independent variables can interpret results more complexly, mainly when interaction effects are present.
- Increased sample size requirements: A larger sample size is preferable to a more straightforward design with fewer independent variables to power such a design adequately.
- Potential for confounding: The presence of interaction effects can make it challenging to determine which independent variable is responsible for observed effects, potentially confounding the results.
- Limited generalizability: The specific conditions of it may not be generalizable to other contexts.