Table Of Contents
What Is Factor Analysis?
Factor analysis is used in big data as the data from a large number of variables may be condensed down into a smaller number of variables. Due to this same reason, it is also frequently referred to as "dimension reduction." Such dimensions of data can be collapsed into one or more super-variables depending on needs.
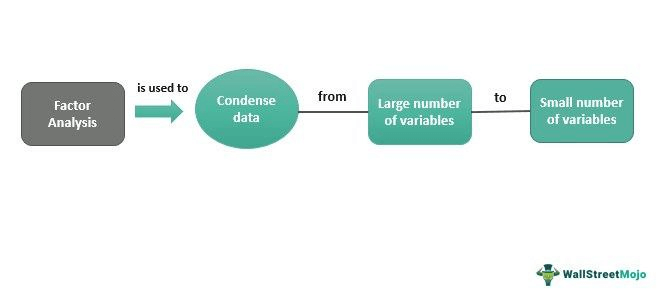
The hidden structure of a group of variables can be uncovered with the use of a factor analysis. It brings the number of variables in the attribute space down to a more manageable level, making it a method that is not dependent on any other variables. Principal Component Analysis is the approach of factor analysis that is most frequently used.
Key Takeaways
- Factor analysis as a method may be utilized to reduce the number of variables that contain the data from a big number of variables to a more manageable number of variables.
- Since factor analysis helps reduce the variables to work with, some people call it "dimension reduction," which means decreasing the size of anything.
- It can be used across various filed like data mining, machine learning, marketing, etc. It has useful applicability anywhere data needs to be reduced for further operations.
- Two types of factor analysis, namely Principle component analysis, and common factor analysis, are widely used by researchers.
Factor Analysis Explained
Factor analysis is widely used in the studies on segmentation. It is used to segment customers or clients directly, or it could serve as an intermediary step before KMeans to minimize the number of variables and prepare them for segmentation.
After simplifying the situation by minimizing the number of variables, factor analysis can help. The sheer quantity of variables may become manageable when conducting lengthy studies that include significant portions of Matrix Likert scale questions. The analysts can better focus on and understand the results by simplifying the data using factor analysis.
When researching customer satisfaction in relation to a product, researchers will typically use surveys to ask a number of questions regarding the product in question. These questions will cover various topics related to the product, such as its features, how easily it can be purchased, how it can be used, its price, how appealing it looks, and so on. On a regular basis, they are quantified using numerical scales. On the other hand, a researcher is looking for the "factors" or already present characteristics that contribute to overall consumer happiness. Most of these are mental or emotional reactions to the product, and they cannot be assessed in a straightforward manner. In factor analysis, variables from the survey are used to derive the factors in a roundabout way.
TypesÂ
When doing factor analysis on a data set, variety of types, including the following can be used:
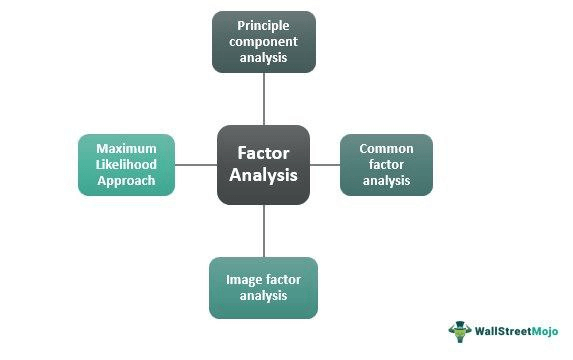
#1 - Principal Component Analysis
It is the methodology used by researchers most of the time. In addition, it takes the factors with the highest variance and places them in the first factor. After that, it takes out the variation that can be accounted for by the first component and then isolates the second factor. In addition, this continues right up to the final consideration.
#2 - Common Factor Analysis
In terms of popularity among researchers, this method comes in at number two. In addition, it separates the elements that contribute to the most prevalent variation. This method, which is utilized in SEM, does not take into account the interpretation of all of the variables.
#3 - Image Factor Analysis
In order to generate an accurate prediction of the factor in image factoring, it utilizes the OLS regression approach and is based on the correlation matrix as its foundation. Image analysis is a typical factor analysis method used to determine the variability of a group of variables.
#4 - Maximum Likelihood Approach
In addition, it operates on the correlation matrix, but it factors using the maximum likelihood technique. Maximum likelihood estimation, is a technique used in statistics to estimate the parameters of an assumed probability distribution based on specific observed data. This is accomplished by optimizing a likelihood function in such a way that, according to the statistical model that is being assumed, the observed data has the highest probability.
Applications
Factor analysis has its applications in many fields. Following are a few examples of the applications.
#1 - Marketing
Marketing promotes products, services, and brands. This statistical technique might aid marketing factor analysis. Businesses use this analysis to establish the link between marketing campaign aspects to improve their long-term performance. It also links customer satisfaction to post-campaign feedback to quantify campaign efficacy and audience impact. Thus, factor analysis may improve marketing input and consumer happiness, increasing sales.
#2 - Data Mining
Factor Analysis can rival artificial intelligence in data mining. FA simplifies data mining by filtering out variables that are linked. Data scientists have long struggled to uncover links and correlate variables. This statistical strategy has improved data mining.
#3 - Machine Learning
Data mining and machine learning go together. Factor Analysis may be a Machine Learning tool because of this. Machine learning algorithms employ Factor Analysis to minimise the number of variables in a dataset to get a more accurate and enhanced collection of observable factors. They are well trained with massive data to make room for additional applications. It is a popular unsupervised machine learning technique for dimensionality reduction. Machine learning and Factor Analysis may create data mining methods and speed up data investigation.
Example
A post written by ‘Seeking Alpha’ demonstrates how factor analysis may be used to determine when it is a good time to invest. They have conducted factor analysis for Vanguard's High Dividend Yield ETF.
They say that factor analysis is a simulation method used to determine what makes an asset tick. For example, when investors primarily put their money into growth securities, value bonds, momentum equities, and so on, does the asset outperform or under perform the respective category of securities?
They concluded the factor analysis that the ETF is most likely suitable for large-cap, value, and quality investing environments, which is in line with the market's appetite at present. In addition, the ETF is a wager against momentum, which could be a positive development considering that momentum assets are now performing worse than other market categories.
Advantages And Disadvantages
The following are some advantages of factor analysis:
- It is possible to make use of both objective and subjective qualities.
- It can be utilized to determine the hidden dimensions or limitations, which may or may not be obvious via direct analysis.
- It is relatively easy to carry out, and at the same time, it does not cost a lot of money and produces reliable results.
- The process of naming dimensions and applying them is up to interpretation.
The following are some of the drawbacks of factor analysis:
- The researcher's capacity to generate a comprehensive and accurate list of product characteristics determines the utility. The value of the technique will be lowered proportionally if crucial characteristics are overlooked.
- It may be challenging to name the components because numerous characteristics may be significantly connected for no obvious reason.
- The factor analysis is unable to provide a meaningful pattern if the variables that have been examined are completely unrelated to one another.
- It is only possible to know what the factors reflect; theorizing is the only way for researchers to gain insight into this topic.