Table Of Contents
F-Test Definition
F-Test in statistics is a hypothesis-testing procedure that considers two variances from two samples. The F-Test is used when the difference between two variances needs to be significantly assessed, i.e., when determining whether or not two samples can be taken as representative of the normal population with the same variance.
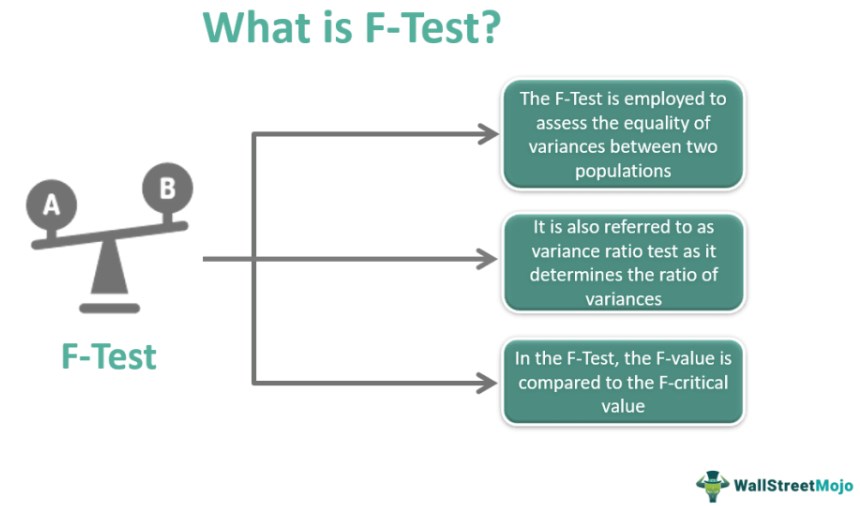
The F-Test helps to determine the overall significance of the regression. It is useful in various situations, such as when a quality controller wants to determine whether the product's quality is deteriorating over time. In addition, it might be useful for an economist to determine whether income variability varies between two populations.
Key Takeaways
- The F-test is a statistical test that evaluates if the variances of the two normal populations are equal.
- One can deem the variance ratio of the test insignificant if F OR = F0.5, and one can assume that the values will be from the same group or groups with similar variances.
- The null hypothesis is rejected, and the variance ratio is considered significant if F> OR = F0.5.
- The F-test vs. t-test: The t-test and the F-test are two separate tests. The T-test compares two populations' means, whereas the other compares two populations' variances.
F-Test in Statistics Explained
F-test in statistics helps to decide whether two populations' variances are equal. This is the variance ratio test because it calculates the ratio of variances. The goal of the test is to determine whether the variance in two populations is equal. It was propounded by British polymath R.A. Fisher and named to honor him. G.W. Snedecor later developed the test.
The following conditions are critical for using the F-test to compare the variances of two populations:
- Normality: the populations must have a normal distribution.
- Independent and random selection of sample items: the selection of the samples' components should be independent and random.
- More than unity: The variance ratio must be one or larger than one; it cannot be less than one. When dividing variance estimates, smaller estimates divide the larger estimates of variances.
- The additive property states that the total of different variance components will equal the total variance, i.e., the total variance is the sum of the variance between samples and the variance within samples.
Formula
1. Sample variances: The formula for calculating sample variances is as follows (an online F-test calculator can make it easier):
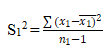
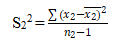
2. Null hypothesis: After the formation of the test, the null hypothesis are either
a) Two samples were from the same group or
(b) The population's variances concerning both samples are equal.
3. To compute the variance ratio, use the formula F = larger estimate divided by a smaller estimate of variance. Regardless of whether S12 or S22, the numerator will always be the larger value.
4. When calculating degrees of freedom, the larger the sample's variance is V1; the smaller variance is V2.
5. Table value of F: the critical value of F is available from the "F-Table" (F-test table) at the determined significance level.
6. Analysis: This involves the comparison of the computed value and the tabulated value. For various levels of significance, there are several F Tables (F-test tables).
(a) The variance ratio is insignificant if F < OR = F0.5. We can assume that the values are from the same group or groups with similar variances.
(b) The null hypothesis is rejected, and the variance ratio is considered significant if F> OR = F0.5.
Calculation Example
Consider the example of the population in a village:
Village | A | B |
Sample size | 10 | 12 |
Mean monthly income | 150 | 140 |
Sample variance | 92 | 110 |
Testing the equality of sample variances with a significance level of 5% with the above-given date.

Variance sample for S12 (sample1) =
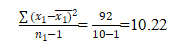
And
Variance sample for S22(sample 2) =
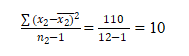
F= S12/ S22 = 10.22/10=1.022
The critical value for v1 (10-1) = 9 and v2 (12-1) =11 and the table value of F at 5% significance level = 2.90. An online f-test calculator can help you in making the calculations easier.
Interpretation
The F statistic helps to decide whether to accept or reject the null hypothesis. The test results must include an F value and an F critical value. The F value is compared to a particular value known as the F critical value. The value derived from the data is the F statistic, or F value (without the "critical" part). In general, one can reject the null hypothesis if the computed F value for a test is higher than the F critical value.
In the example above, F's computed value (1.022) is less than its table value obtained from the F table (2.90). As a result, one can conclude that the null hypothesis is true and that the variance of the two samples is equal.