Table Of Contents
What Is Experimental Data?
Experimental data is collected through experiments or controlled studies to test hypotheses, strategies, or investment models. These experiments are designed to provide empirical evidence and insights into financial markets, investment decisions, trading strategies, and economic phenomena. It is valuable because it allows us to understand better how various factors and variables affect financial outcomes.
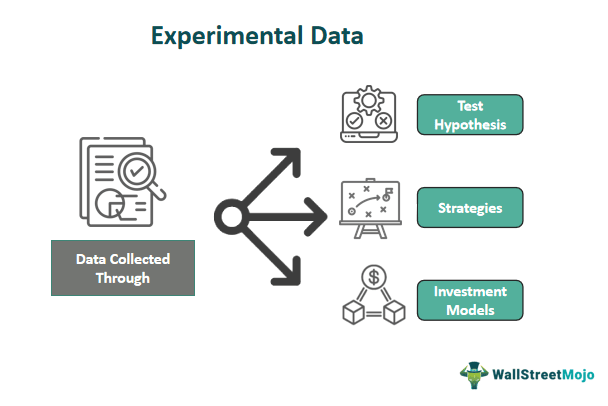
Researchers and financial analysts analyze this data to conclude, make predictions, and inform investment strategies or policy decisions. It can help identify patterns, anomalies, and trends that may not be evident through traditional financial analysis methods, contributing to a more comprehensive understanding of financial markets and behavior.
Table of contents
- Experimental data is information collected through controlled experiments or studies where researchers manipulate variables to observe their effects.
- It is crucial for empirically validating theories, models, and hypotheses, providing evidence for cause-and-effect relationships, informing decision-making, and enhancing our understanding of various phenomena.
- It differs from observational data because it involves controlled interventions to establish causal relationships, while observational data typically explores correlations in natural settings.
- It exists across various fields, such as science (lab experiments, clinical trials), finance (trading experiments, behavioral finance studies), psychology, and medicine (drug trials).
Key Takeaways
- Experimental data is information collected through controlled experiments or studies where researchers manipulate variables to observe their effects.
- It is crucial for empirically validating theories, models, and hypotheses, providing evidence for cause-and-effect relationships, informing decision-making, and enhancing our understanding of various phenomena.
- It differs from observational data because it involves controlled interventions to establish causal relationships, while observational data typically explores correlations in natural settings.
- It exists across various fields, such as science (lab experiments, clinical trials), finance (trading experiments, behavioral finance studies), psychology, and medicine (drug trials).
Experimental Data Explained
Experimental data is information collected through carefully designed experiments or controlled studies to investigate financial theories, strategies, or market phenomena. This data collection method empirically examines and analyzes various aspects of finance, providing valuable insights into market behavior, investment decisions, and economic trends.
The process of generating experimental data in finance involves the following steps:
- Hypothesis Formulation: Researchers formulate specific hypotheses or questions they want to investigate. These hypotheses often relate to financial theories, investment strategies, or market dynamics.
- Experimental Design: Researchers design controlled experiments or studies to test their hypotheses. They carefully define the variables and establish experiment conditions and parameters.
- Data Collection: This may involve conducting surveys, tracking market movements, observing investor behavior, or simulating market scenarios using historical data.
- Controlled Environment: Experiments in a controlled environment isolate the variables of interest. This control allows researchers to establish causal relationships between variables more effectively.
- Statistical Analysis: Collected data is subject to rigorous statistical analysis. Researchers use various statistical techniques to examine relationships, draw conclusions, and determine the significance of their findings.
- Interpretation: Results from the analysis draw meaningful insights. Researchers assess whether the data supports or refutes their initial hypotheses and consider the implications for financial theory or practice.
- Publication and Application: Findings from experimental data inform investment strategies, policy decisions, or financial product development.
Types
Experimental data are of several types based on the nature of the experiments and the data collection objectives. Here are some common types of experimental data:
- Behavioral Finance Data: This data type focuses on understanding how psychological and emotional factors influence financial decision-making. Researchers conduct experiments to investigate biases, heuristics, and behavioral patterns of investors. For example, experiments may explore the impact of loss aversion, overconfidence, or herding behavior on investment choices.
- Market Microstructure Data: Experimental data in market microstructure examines the intricacies of financial markets, including trading mechanisms, order execution, and market dynamics. Experiments in this category often involve simulated trading environments to study how different trading strategies, market designs, or trading algorithms affect market liquidity and price formation.
- Portfolio Management Data: Researchers and practitioners use experimental data to evaluate the performance of various portfolio management strategies, asset allocation models, and risk management techniques. These experiments determine which investment strategies are more effective under different market conditions.
- Risk Assessment and Pricing Data: This experimental data validates and refines risk assessment and pricing models for financial instruments. Researchers conduct experiments to assess the accuracy and reliability of models such as Value at Risk (VaR) or option pricing models.
- Financial Education and Decision-Making Data: Experimental data assesses the effectiveness of financial education programs and tools in improving individuals' financial literacy and decision-making skills. Experiments may involve measuring the impact of financial literacy interventions on investment choices or retirement planning.
How To Analyze?
Analyzing experimental data is a systematic process that involves several critical steps to derive meaningful insights and conclusions. These steps ensure the analysis of data for informed decision-making:
- Data Preparation: The initial step involves cleaning and organizing the collected data to ensure its quality and integrity.
- Descriptive Statistics: These statistics provide an initial overview of the data's central tendencies and variability, helping to identify key characteristics.
- Data Visualization: Visual representations, such as charts, graphs, histograms, and scatter plots, depict the data visually.
- Hypothesis Testing: Tests like t-tests, ANOVA, chi-squared tests, or regression analysis determine whether the data has statistically significant differences or relationships.
- Correlation Analysis: The relationships between variables, through correlation analysis, calculate correlation coefficients like Pearson's correlation. This is particularly valuable in understanding how variables interact within financial contexts.
- Regression Analysis: For more complex relationships, regression analysis applies to model the impact of independent variables on a dependent variable. Regression quantifies and predicts relationships within the data, providing valuable insights.
- Time Series Analysis: Time series data uses moving averages, exponential smoothing, or ARIMA models. These methods help uncover trends and seasonality and provide forecasting capabilities.
- Statistical Software: Specialized software packages like R, Python, SAS, or MATLAB are usually for advanced data analysis and calculations, streamlining the analytical process.
- Interpretation: The results interpret the research objectives. The findings' practical significance and contribution to understanding financial markets or behavior are key areas for interpretation.
Examples
Let us understand it more with the help of examples:
Example #1
Suppose a financial institution is experimenting to test the effectiveness of a new algorithmic trading strategy designed to maximize returns in a volatile market. They collect experimental data over six months, during which the algorithm makes buy and sell decisions based on predefined criteria. The data includes daily trading volumes, price movements, and portfolio returns.
Analyzing this experimental data involves calculating daily returns volatility measures and comparing the algorithm's performance against a benchmark index, such as the S&P 500. Statistical tests, like the Sharpe ratio or Jensen's alpha, assess risk-adjusted performance.
Example #2
A recent report by Synthace in 2023 unveiled vital challenges and opportunities in life science research and development (R&D) experiments. The report highlights the critical issues researchers face in this field and offers insights into potential solutions.
Synthace's findings underscore the growing complexity of life science experiments and the need for more efficient and reproducible processes. The report also emphasizes the importance of data management and analytics in optimizing R&D outcomes.
Additionally, it sheds light on the growing role of automation and digitalization in life science R&D, emphasizing their potential to streamline workflows and enhance productivity.
Furthermore, the report discusses the challenges associated with data integration and research collaboration. It suggests that greater collaboration and data sharing could lead to breakthroughs in life science research.
Experimental Data vs Observational Data
Following is a brief comparison of experimental data and observational data:
Aspect | Experimental Data | Observational Data |
---|---|---|
Data Collection Process | Data is collected through controlled experiments or studies where researchers manipulate variables to observe their effects. | Data is collected by observing naturally occurring events or behaviors without intervention or manipulation by researchers. |
Control Over Variables | Researchers have control over independent variables and can manipulate them to isolate cause-and-effect relationships. | Researchers have limited or no control over variables, making it challenging to establish causal relationships. |
Research Purpose | Typically used to test hypotheses, validate theories, and investigate causal relationships by intentionally varying factors of interest. | Often used for descriptive analysis, exploring correlations, and generating hypotheses, as researchers simply observe existing phenomena. |
Experimental Bias | Researchers can control and minimize bias through randomization and blinding techniques. | Observational data may be subject to various biases, including selection bias, confirmation bias, and observer bias. |
Data Collection Setting | Data is collected in a controlled environment designed to replicate specific conditions of interest. | Data is collected in natural settings or real-world contexts, reflecting real-life conditions. |
Research Design | Researchers design experiments with predefined protocols and procedures, often using control and treatment groups. | Observational studies can be cross-sectional (data collected at a single point in time) or longitudinal (data collected over time). |
Experimental Data vs Theoretical Data
Below is a comparison of experimental data and theoretical data:
Aspect | Experimental Data | Theoretical Data |
---|---|---|
Data Source | Collected through real-world experiments, observations, or simulations where actual data points are obtained from practical scenarios. | Generated based on theoretical models, mathematical equations, or assumptions, often without direct empirical validation. |
Origin of Data | It may be highly idealized or simplified, making it less suitable for direct application to real-world scenarios without empirical validation. | Based on theoretical constructs and assumptions, and may not necessarily reflect real-world conditions accurately. |
Data Variability | Exhibits inherent variability and may include noise or unexpected factors due to real-world complexities. | Generally free from variability and uncertainties as it is constructed according to specific theoretical premises. |
Validity and Realism | Represents real-world situations, allowing for direct applications to practical problems and decision-making. | May be highly idealized or simplified, making it less suitable for direct application to real-world scenarios without empirical validation. |
Data Collection Method | Involves empirical data collection through experiments, surveys, observations, or simulations in controlled or natural settings. | Generated through mathematical or analytical modeling, often using assumptions and simplifications to derive data points. |