Table Of Contents
What Is Elastic Net?
Elastic net (also called ELNET) regression is a statistical hybrid method that combines two of the most often used regularized linear regression techniques, lasso, and ridge, to deal with multicollinearity issues when they arise between predictor variables. Regularization aids in solving the overfitting issues with the models.
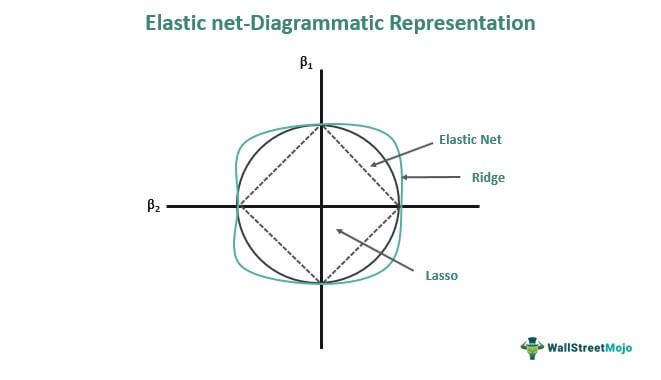
It is also used for regularizing and choosing the essential predictor variables that significantly impact the response variable. Ridge employs an L2 penalty, while lasso employs an L1. Since the elastic net utilizes both the L2 and the L1 models, the question of choosing between either one does not arise.
Key Takeaways
- Elastic net is a combination of two of the best shrinkage regression approaches. They are Ridge regression (L2 penalty) and LASSO regression
- ELNET uses coefficient regression shrinkage towards zero or equal to zero to reduce the occurrence of predictor variables.
- The primary idea underlying the elastic net is regularization. Regularization is considered in situations where the model is overfitting. It is a solution for reducing errors by properly fitting a function to the training dataset.
Elastic Net Explained
Elastic net is a regression technique that simultaneously applies regularization and variable selection. The primary idea underlying the elastic net is regularization. Regularization is considered in situations where the model is overfitting. Overfitting is a problem that arises when the model gives results on the training dataset but produces errors on the test dataset. Regularization is a solution for reducing errors by properly fitting a function to the training dataset. These functions are also otherwise known as elastic net penalties.
The elastic net penalty comes in two varieties: l1 and l2. The lasso and ridge regression models are two types of regularization models that apply l1 and l2 penalties, respectively. The absolute value of the coefficient's magnitude is added as a penalty term in the lasso regression model. The ridge regression adds the coefficient's squared magnitude as a penalty to the loss function.
Thus, it deals with both multicollinearity problems and the selection of regression coefficients. ELNET uses coefficient regression shrinkage towards zero or equal to zero to reduce the occurrence of predictor variables. The tuning parameter (λ1) multiplied by the sum of coefficient variables (l1 norms) absolute values is utilized for this purpose. The tuning parameter (λ2) is multiplied by the l2 norm's squared coefficient variables to handle the high correlation between the predictor variables. The ELNET regression approach helps produce a fitting, interpretable model by minimizing unnecessary variables that do not appear in the final model to improve prediction accuracy. The ELNET manages multicollinearity by maintaining or excluding highly correlated predictor variables from the fitted model.
Formula
An elastic net is a combination of two regressions, lasso, and ridge, and hence the resultant equation to calculate it is:

Examples
Check out these examples to get a better idea:
Example #1
A study gathered the Social Vulnerability Index (SVI) and Democratic vote percentage to determine which county-level sociodemographic parameters significantly correlated with the COVID-19 case rate. ELNET regression was used for the study to deal with issues of model overfitting and variable collinearity. Statistically speaking, it resulted in improved predictions compared to the results of multiple regressions.
Example #2
ELNET and lasso regressions were compared for major element analysis of the rocks and soil using laser-induced breakdown spectroscopy (LIBS). It was intended to be used by "Curiosity," the Mars Science Laboratory rover, to analyze soil and rock.
It was found that the RMSEP (root mean squared errors of prediction) value was lower for seven of the ten main elemental oxide compositions when using the ELNET model. For each of the ten major elemental oxides, the ELNET model had more non-zero coefficient values than the lasso. This results from the elastic net penalty's ability to average. While both methods proved reliable, ELNET projected a more stable model compared to the lasso model.