Distortion Risk Measure
Published on :
21 Aug, 2024
Blog Author :
N/A
Edited by :
N/A
Reviewed by :
Dheeraj Vaidya
What Is A Distortion Risk Measure?
A distortion risk measure in finance and insurance is defined as the expected loss level and measures to mitigate a situation that can occur in the future. It is named so because it is a measure that distorts unexpectedly unfavorable or unexpected contingencies. Moreover, it provides a systematic way of assessing the potential losses or deviations from the expected outcome.
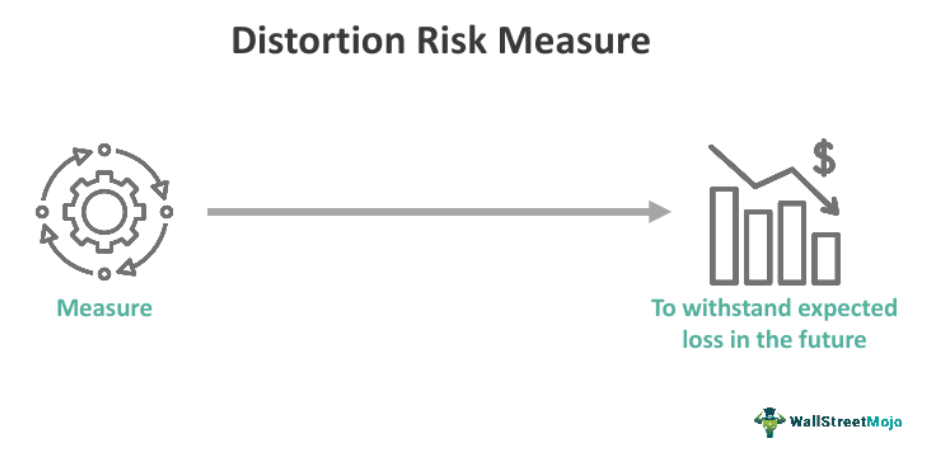
Distortion functions help asset managers deal with client's risk tolerance through the measures they adopt. They operate similarly to general risk measures and have similar attributes. An initial application of these risk measures includes various insurance issues. For instance, calculating insurance premiums, how much capital is needed, and how to allocate money.
Table of contents
- A distortion risk measure in finance and insurance is defined as the expected loss level and measures to mitigate a situation that can occur in the future.
- Distortion functions help asset managers deal with client's risk tolerance through the measures they adopt.
- It provides a systematic way of assessing potential losses or deviations from expected outcomes.
- Some applications of this measure include risk evaluation, risk reporting and management, portfolio optimization, strategic hedging, asset pricing, and risk budgeting.
Distortion Risk Measure Explained
Distortion risk measurement (DRM) is an expected value that is risk-adjusted, and it is a popular method for valuing insurance risks. This value is distorted by a probability weighting function, a distortion function, or a modification projecting the decision maker's risk preference. Moreover, it is used in risk management to quantify and evaluate the risk associated with an investment or portfolio.
Decisions that accurately characterize a decision maker's risk attitude through the distortion function can be difficult. When the decision-making problem involves multiple stakeholders who cannot agree. Second, it is too complex as a function, which may make it difficult to identify or elicit a specific distortion function. Hence, that accurately characterizes a decision-makers risk attitude. In these situations, it might make sense to create a new set of distortion functions using the partial information that is currently available.
Therefore, this helps reflect the uncertainty of a decision-makers risk attitude. It also helps to consider the worst-case function from the set for this measure. Hence, to reduce the risk brought on by the ambiguity of the actual distortion function. Therefore, the distortion function maps the potential outcomes of an investment or portfolio to a distorted space, where the risk measure is calculated.
Making educated decisions requires a thorough understanding of how resilient risk indicators are to changes in the distributions of underlying losses (distributional uncertainty). This measure is a crucial instrument in risk management, particularly for investors concerned about downside risk and needing a more precise assessment of the probability that losses will surpass a specific threshold.
Properties
Any measure of distortion risk must adhere to the following rules:
#1 - Additivity For Comonotonic Risks
All random variables Xi and any distortion function "g." take the form of
Wg(S c ) = Wg(Xi).
#2 - Positive Homogeneity
It takes the form of Wg(a X) = a Wg(X) for any distortion function, namely "g," any random variable, namely "X," and any non-negative constant, namely "a."
#3 - Translation Invariance
It takes the form of Wg(X+b) = Wg(X) + b for any distortion function "g," any random variable such as "X," and any constant such as "b."
#4 - Monotonicity
It takes the form of Wg(X)£ Wg(Y) for any distortion function, namely "g" and X and Y as any two random variables where X £ Y and with the probability 1.
Examples
Check out these examples to get a better idea:
Example #1
One example of this measure is the expected shortfall (ES), also known as conditional value-at-risk (CVaR). Let's consider an example to illustrate the calculation of ES as a distortion risk measure:
Suppose Michael has a portfolio with a distribution of returns represented by the random variable X. He wants to calculate the ES at a 95% confidence level (α = 0.95), which corresponds to the 5th percentile of the distribution.
- Determine the VaR: Calculate the 5th percentile of the distribution, which represents the VaR at the specified confidence level. Let's assume the 5th percentile is $10,000.
- Calculate the Expected Shortfall: Integrate over-the-tail losses beyond the VaR level to obtain the average loss.
ρ(X) = (1/(1 - 0.95)) ∫ F_X^(-1)(q) dq.
The integration captures the expected losses beyond the 5th percentile.
Moreover, the resulting value of ρ(X) represents the Expected Shortfall, which estimates the average loss beyond the VaR threshold.
It's important to note that the distortion function used in this example is the integration of the quantile function. However, other distortion functions, such as the Tail Value-at-Risk (TVaR), can also measure the tail risk.
This example demonstrates how the ES distortion risk measure incorporates both the shape of the distribution and the investor's risk preferences by considering the expected losses beyond the VaR threshold.
Example #2
Let's say, Dan, an investor, has a portfolio of stocks that he expects to give a return above 10%. However, recent events got him worried about downside risk, and he wanted to estimate the likelihood of his losses exceeding the threshold of 10% and to know the lower of his return distribution. He decides to employ a value-based distortion risk measure as it is more suitable to find out the lower end of the distribution.
Applications
Some of the applications of distortion risk measures are given below:
- Risk evaluation: The risk measures estimate the risk of investments and the losses they potentially incur.
- Risk reporting and management: These risk measures can give investors a more precise idea of the risk involved in their investments. It helps detect and reduce risk exposures in the portfolio.
- Strategic hedging: Planning hedging strategies are possible through these measures. It can help reduce the likelihood that losses surpass a specific threshold.
- Asset pricing: Depending on an asset's projected risk and return characteristics, its price can be determined using the distortion risk measure.
- Risk budgeting: Risk budgets can be distributed among various portfolio components according to their predicted risk and returns using the distortion risk measure.
Frequently Asked Questions (FAQs)
Distortion Risk Measures come in various forms and are frequently employed in the financial industry. Among the most common varieties are: Value-based, Entropic, Expected Shortfall-based, and Worst-case Distortion Risk Measures.
The first step is selecting a distortion function. Estimating expected return and volatility will be the second step; setting the distortion function will be the third step; and assessing the likelihood of losses surpassing a predetermined threshold will be the fourth step in evaluating the measure.
The risk preferences and objectives of the investor will determine the best distortion risk measure. Risk tolerance, the nature of the portfolio, market conditions, etc., is a few factors that can be considered.
Traditional risk measures, such as standard deviation, quantify the dispersion of returns around the mean but do not specifically focus on extreme events or tail risks. Distortion risk measures, on the other hand, prioritize the extreme tail of the distribution and provide a more comprehensive assessment of potential losses during adverse market conditions.
Recommended Articles
This article has been a guide to what is Distortion Risk Measure. Here, we explain it in detail with its properties, examples, and applications. You may also find some useful articles here -