Discrete Choice Model
Published on :
21 Aug, 2024
Blog Author :
N/A
Edited by :
Rashmi Kulkarni
Reviewed by :
Dheeraj Vaidya
What Is A Discrete Choice Model?
The Discrete Choice Model (DCM) refers to the statistical technique that helps researchers interpret and analyze how individuals make decisions when exposed to limited alternatives to choose from based on their characteristics. It is a suitable research method to make appropriate choices by modeling the surveyed data.
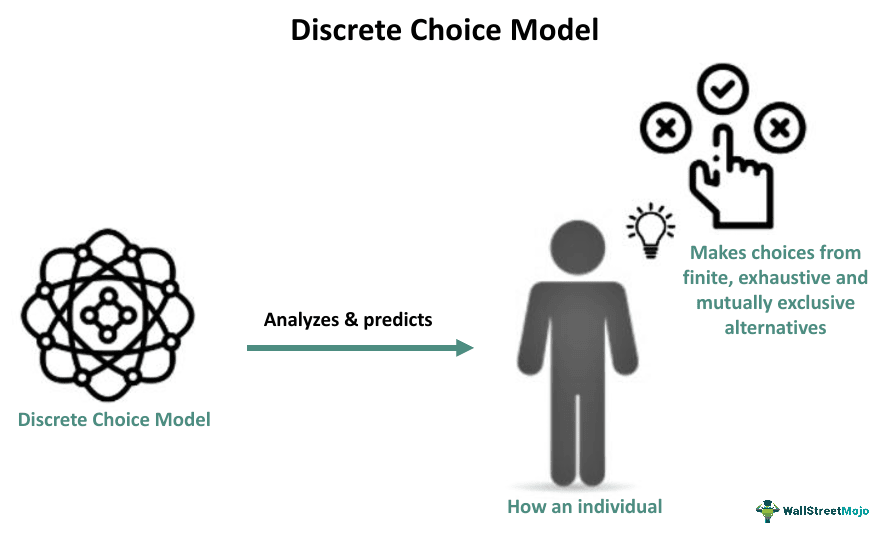
Per this model, individuals are assumed to make decisions by choosing the alternative that provides them with the highest utility or satisfaction. This framework is applicable in econometrics, marketing, governance, computer science, healthcare, psychology, transportation planning, and other fields where critical decision-making is needed. It is also called a Disaggregate Model, as it focuses on individual behavior and not the overall market trends in a given field.
Table of contents
- A Discrete Choice Model (DCM) is a statistical method to determine, infer, and predict the choices individuals are likely to make in situations where they are required to consider a finite number of options during decision-making.
- The alternatives selected for such decision-making and prediction frameworks must be finite, exhaustive, and mutually exclusive.
- These models find applications in varied fields where data-driven decision-making is essential. Some examples include econometrics, marketing, healthcare, transportation planning, environmental science, psychology, and sociology. They provide valuable insights to businesses, economists, policymakers, and researchers.
- Disaggregate models are of various types, such as binary choice, multinomial logit, nested logit, mixed logit, and heteroscedastic extreme value models.
Discrete Choice Model Explained
Discrete choice models (DCM) are used in various fields, such as economics, marketing, transportation, policy-making, and urban planning, to understand and predict choices made by individuals among a set of discrete alternatives. Various discrete choice model courses are available online that enable researchers to enrich their knowledge in this area.
The utility maximization assumption, which states that individuals choose alternatives that they perceive will give them the maximum satisfaction or benefit under given conditions, is a key principle governing the DCMs. It gives crucial input about an individual’s behavior and decision-making process, including the trade-off analysis an individual applies while selecting an option. It highlights a model’s predictive capabilities and limitations under the given circumstances.
These frameworks are employed to analyze various decision-making scenarios, like consumers choosing between different products, travelers selecting transportation modes, or individuals making career choices. Such frameworks help businesses gain knowledge of consumer preferences, their buying behavior, and decisions regarding product design, pricing strategies, and market segmentation. Moreover, companies can tailor their marketing strategies to target a specific segment effectively.
In urban planning and transportation, the dynamic discrete choice models are used to interpret the impact of various policies and infrastructure changes on people's travel behavior. It serves as a crucial input for designing efficient transportation systems. Further, such techniques allow researchers to perform predictive modeling based on different scenarios.
In the healthcare sector, healthcare providers apply this method to gain insights into the patients' preferences for different treatment options, enabling them to make informed decisions about treatment strategies. Also, environmentalists and eco-friendly businesses often gauge an individual's environmental behavior, such as predicting their household choices regarding energy-efficient appliances or transportation modes for environmental policy planning.
Types
Discrete choice models are widely used in different fields to analyze decision-making processes where individuals select from a finite set of options. These include:
- Binary Choice Model: It is a basic model that involves decisions between two alternatives, like buying or not buying a product.
- Multinomial Logit Model (MNL): Extending the binary choice model, MNL assumes choice probabilities follow a multinomial logistic distribution where the individual has multiple alternatives.
- Nested Logit Model: The nested discrete choice model allows for correlated choices within groups of alternatives, where one selection of one option depends on not selecting the others, thus providing a flexible representation of decision-making. In this model, alternatives are organized into “nests”, with choices that are closely related to each other grouped in a set.
- Mixed Logit Model: It is also called the Random Parameters Logit Model, and it accommodates varying parameters across individuals, capturing preference heterogeneity. A key aspect of this model is that it assumes that the parameters of the utility function are not fixed across the population. This is because individual preferences for different attributes of the alternatives vary.
- Heteroscedastic Extreme Value (HEV) Model: This model addresses cases where the variance error terms increase with the size of the alternatives, handling issues related to scale differences. It is based on the assumption that choices with larger scales than normal typically have more uncertainty or randomness attached to them.
Examples
In real life, we come across various instances where many products or services are designed just right to cater to our needs and preferences. It is possible because of the many dynamic discrete choice models. Let us study some examples.
Example #1: Predictive Modeling for Transportation Planning
Suppose a city is planning to introduce a new public transportation system, such as a bullet train or bus rapid transit. To plan the routes and estimate potential ridership effectively, transportation planners can employ discrete choice models like Multinomial Logit (MNL) or Nested Logit. These models help predict how individuals will choose between various transportation modes (car, bus, train, walking, etc.) based on factors such as travel time, cost, comfort, and convenience.
By collecting survey data and applying discrete choice models, planners can estimate residents' preferences and choices. It assists city planners in making informed decisions about designing and implementing new transportation systems, optimizing routes, and estimating potential ridership. It leads to the development of more efficient and user-friendly public transportation systems tailored to residents' preferences and needs.
Example #2: A Global Healthcare-related Study
The rise of global health issues, notably COVID-19, has blurred the distinction between global and national health. Due to this, the need to onboard experts in the field, primarily through involvement in health-related international organizations, has gained great prominence. However, because of the underrepresentation of certain member states, like Japan, in these organizations, improving the system has become important.
The study was designed to understand the specific job preferences of Japanese experts interested in undertaking assignments across healthcare-related international organizations. Additionally, it sought to propose policies for enhancing capacity development and facilitating international assignments.
Analysts used a Discrete Choice Experiment (DCE) survey, classifying participants into Seekers (those aspiring to work), Workers (current employees), and Resignees (former employees). Job preferences were analyzed using a binary logit primary effects model. Across all groups, factors like duty station, salary, Work–Life Balance (WLB), and job satisfaction were identified as pivotal.
In addition to the above, Seekers and Resignees emphasized the importance of return posts and employer contributions to welfare benefits. Both exhibited tendencies to avoid uncertainty, suggesting a need for tailored support strategies. Addressing social challenges linked to the undervaluation of such international experiences could be achieved through initiatives such as sabbatical leaves or reemployment programs.
Additionally, actively promoting favorable conditions (duty station, salary, WLB, and job satisfaction) through incentive packages at international organizations, especially at career development events, can be instrumental. This shows how DCMs facilitate research and analysis.
Advantages And Disadvantages
Discrete choice models are widely used in various fields to model choices made by individuals between different options. However, researchers must consider the following benefits and limitations before using this model.
Advantages
- Flexibility: The discrete choice model can handle different types of choice scenarios, making it a versatile mechanism for analysis across various applications such as transportation, marketing, and healthcare.
- Facilitates Decision-making: These models are used to predict the likelihood of individuals preferring specific alternatives; thus, they help make crucial business and policy planning decisions.
- Interpretability: The model parameters often have clear interpretations, allowing researchers to understand the factors influencing choices.
- Policy Analysis: Such frameworks are valuable for policymakers to examine the potential effects of policy changes on consumer choices and market dynamics.
- Accounting for Heterogeneity: It considers individual-level or disaggregate responses, capturing differences in preferences and behaviors among individuals.
Disadvantages
- Extensive Data Requirements: Discrete choice models need a significant amount of data, especially when modeling complex choice scenarios with multiple alternatives and factors influencing these preferences.
- Based on Assumptions: Like many statistical models, these methods also rely on certain assumptions, without which researchers cannot draw accurate conclusions.
- Computationally Intensive: Evaluating disaggregate models, especially when dealing with a large number of alternatives and individuals, can be exhaustive and time-consuming.
- Challenging Estimates: Estimating parameters in these methods, especially in cases of mixed or nested logit models, can be complex and may require advanced statistical techniques.
- Limited to Categorical Choices: These models are suitable for scenarios where choices are categorical. However, they are unsuitable for modeling continuous outcomes or situations where choices are vague.
Discrete Choice Model vs Conjoint Analysis
Both discrete choice models and conjoint analysis are techniques used in market research to understand consumer preferences, but they have certain dissimilarities, as discussed below.
Basis | Discrete Choice Model | Conjoint Analysis |
---|---|---|
Meaning | These are statistical frameworks used to analyze and predict choices made by individuals when they are exposed to finite alternatives. | It is a market research technique used to understand how different attributes of a product or service influence consumers' preferences and decision-making. |
Significance | It applies broader statistical frameworks compared to other models. | It is a specific market research method. |
Methodology | Choices are based on the personal preferences of individuals from a finite set of exhaustive and mutually exclusive alternatives, where each alternative has a set of attributes and associated preference levels. | It involves presenting respondents with different product profiles, each with varying levels of attributes, and asking them to rank or rate their preferences. |
Key Focus | The focus is on understanding the factors influencing individual choices and estimating the probabilities of different alternatives being chosen. | The focus is on determining the relative importance of different attributes and their impact on overall preference. |
Analyzes Behavior of | DCM analyzes the behavior of individuals, taking into account the factors that influence their choices between different options. | Conjoint analysis facilitates the study of markets by examining consumer preferences and their influence on product/service offerings. |
Applied in/for | It is used across fields like transportation, marketing, econometrics, governance, computer science, healthcare, sociology, and psychology. | It is used in product design, pricing strategy, market segmentation, product positioning, and competitor analysis to optimize products or services. |
Frequently Asked Questions (FAQs)
Discrete choice modeling is a systematic statistical procedure that includes the following steps:
- Defining the research query,
- Gathering pertinent data regarding choices and their attributes,
- Preparing data,
- Selecting a model (such as logit or probit),
- Estimating or identifying parameters,
- Evaluating performance,
- Interpreting coefficients,
- Generating predictions,
- Validating the model, and
- Disseminating the result.
The choices available to an individual must align with certain assumptions or properties to be treated under this framework:
The alternatives or options must be exhaustive;
They must be mutually exclusive and
They must be finite in number.
Linear regression is used when the outcome variable is continuous, meaning it can assume any numeric value within a range, and it aims to predict this variable based on one or more predictor variables. In contrast, discrete choice models are employed when the outcome variable is categorical, representing distinct choices among a finite set of options. It estimates the odds of choosing specific alternatives, making them ideal for situations where decision-making processes involve discrete alternatives rather than a continuous spectrum of values.
Recommended Articles
This article has been a guide to what is a Discrete Choice Model. We explain its examples, comparison with conjoint analysis, types, advantages, and disadvantages. You may also find some useful articles here -