Data Sampling
Published on :
21 Aug, 2024
Blog Author :
N/A
Edited by :
Alfina
Reviewed by :
Dheeraj Vaidya
What Is Data Sampling?
Data sampling is a statistical method that analyzes a representative subset of data points to identify patterns and trends in the broader data set. It involves selecting a specific group from a population to examine that whole population. It gives meaningful insights into the largest data set.
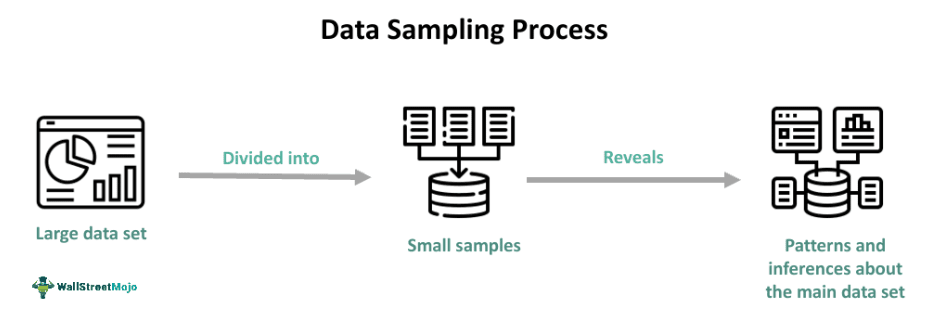
Sampling is a method used to obtain a representative sample from a larger population, allowing researchers to study small groups and make accurate predictions. It allows data scientists and analysts to use smaller, manageable data for analytical models, enabling quicker results and informed decisions. Being time-efficient and resource-efficient, this technique reduces the need for extensive analysis.
Table of contents
- Data sampling is selecting a representative subset of information from a larger quantity of data for analysis.
- It involves defining the target population, determining sample size, selecting the sampling technique, collecting and analyzing the data, and generalizing the findings.
- Different data sampling methods such as simple random, cluster, convenience and stratified data sampling are employed to carve out inferences.
- It offers advantages such as cost and time efficiency, feasibility, and accuracy but also has limitations related to sampling error and potential bias.
Data Sampling Explained
Data sampling is the process of choosing statistical samples from a population to estimate the characteristics of the full population. It is the primary method for data collection. This makes it easier to analyze and conclude statistical judgments about the population. Data sampling makes data analysis more effective, lowers computational complexity, and facilitates decision-making. It helps forecast, identify trends, and form meaningful inferences or judgments.
Additionally, it provides information used to make decisions in the social sciences, government policies, healthcare issues, and key business decisions. Sampling guarantees the validity of the research, assuring realizable, usable, and accurate data while minimizing biases. It also helps with population comprehension and supports evidence-based policymaking by offering trustworthy data for the evaluation, planning, and implementation of policies.
Sampling makes drawing conclusions and judgments about a wider population possible, which is essential in many research domains. It optimizes resources by lowering the time, expense, and effort needed for data collection and processing. One can obtain reliable sampling data through representative samples, using proper methodologies, maintaining a sufficient sample size, minimizing biases, and accurately documenting the sampling procedure. The use of statistical metrics and results validation against the used population data both help to increase reliability.
Process Steps
Data sampling involves several steps, which are as follows:
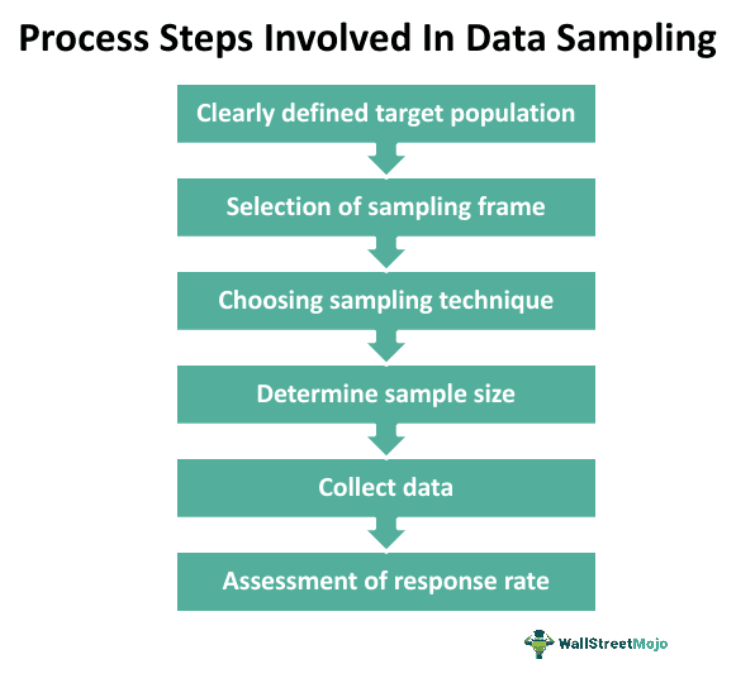
#1 - Clearly Defining Target Population
The first step of the sampling process is clearly defining the target population. It is the number of people in a defined area or under a category. One should clearly define it as an accurate representation of the target population.
#2 - Selection Of Sampling Frame
A sample frame is the list of or number of actual cases that will serve as a base for drawing a sample. It must be a representation of the population.
#3 - Choosing Sampling Technique
Sampling is selecting a subset from a larger population or sampling frame. Using sampling, one can conclude a population or make generalizations about an accepted theory. This depends on the sampling method chosen. It is important to define sampling and consider the rationale behind sample selection in research before analyzing the various sampling techniques. Sampling methods can generally be classified into two categories: Probability or random sampling and Non-random or non-probability sampling.
#4 - Determining Sample Size
One should take a random sample, which shall be sufficient to prevent biases or sampling errors. This size depends on the population's complexity, the researcher's objectives, and the statistical techniques utilized in the data analysis. Larger samples lessen the possibility of bias; however, declining results may arise at a certain sample size, which one must weigh against the researcher's resources.
#5 - Collecting Data
The following phase is data collection of the target population, sampling frame, sampling procedure, and sample size after their determination. This entails using the proper techniques, such as surveys, questionnaires, interviews, or data extraction from existing databases, to collect information from the chosen sample.
#6 - Assessing Response Rate
The response rate is the number of cases who agreed to participate in the study. These cases were selected from the initial sample. Response rate is crucial because each absence could skew the final sample. In some ways, a large sample size, using the appropriate sampling method, and clearly defining the sample can lessen the possibility of sample bias.
Types
Data sampling can be categorized into probability sampling techniques and non-probability sampling techniques.
Probability data sampling methods consist of simple random sampling, stratified random sampling, systematic random sampling, cluster sampling and multistage sampling.
Non-probability data sampling methods consist of convenient, purposive, quota, snowball, and consecutive sampling.
1. Probability Data Sampling
- Simple random sampling: Each component has an equal chance of being chosen. A complete list of the components is necessary, but the choice of components is random.
- Systematic random sampling: In this method, one chooses the elements for sampling in regular intervals (they could be related to time, order, or space). An exhaustive list can be optional or mandatory during the grouping of a homogeneous population.
- Stratified random sampling: One can use stratified data sampling when a population is heterogeneous.
- Cluster sampling: It is employed in place of elements when the target population is homogeneous yet dispersed over a large geographic area. The population is divided into clusters, each assigned a number, and then the predetermined number of clusters is randomly chosen to enable probability sampling for a large population.
- Multistage sampling: Multistage sampling takes samples from within a larger sample using two (or more) probability sampling procedures. Initially, a sample is randomly chosen, and another sample is taken.
2. Non-Probability Sampling
- Purposive sampling: Purposive sampling, or deliberate or judgmental sampling, selects members for a sample based on the study's purpose.
- Convenience sampling: Convenience sampling selects members depending on their accessibility, which is often useful in pilot testing.
- Snowball sampling: Snowball sampling (chain or sequential sampling) is useful when one respondent identifies others from friends, relatives, or known sources.
- Quota sampling: Quota sampling selects members based on certain characteristics chosen by the researcher, serving as a quota for selection.
- Consecutive sampling: Consecutive sampling is a non-probability method where the researcher selects samples based on ease or convenience. This method involves selecting a group for research, collecting samples, and providing results. After completing the research, the researcher moves on to the next group, allowing them to refine their research work and work with multiple samples.
Examples
Let us look at a few examples to understand the concept better.
Example #1
Suppose a statistics major, Dan, aims to start an entrepreneurial journey through a financial venture. He decided to use data sampling to validate his idea and understand the preferences and behaviors of potential customers. He executed data sampling in Excel to make it easier for him.
Dan targets individuals aged 25–35, as he thinks they are the population that wants financial stability. He uses stratified sampling to select 500 participants from different income brackets. These 500 people will give him a general idea of how the masses think (here, the 25-30-year-olds).
He collected data through online surveys about financial goals, investment preferences, and spending habits. His inferences told him that most prioritized long-term financial stability over short-term gains. It also revealed that most 30-year-olds were working toward achieving financial freedom through various methods.
Based on this, Dan refines his entrepreneurial idea to focus on long-term investment strategies with his inferences from data sampling in Excel. Additionally, he plans to use Google Analytics data sampling on his website to create financial education programs tailored to his target audience's needs. Using Google Analytics data sampling could further help him develop his ideas.
Example #2
The American Housing Survey (AHS) is a biennial survey conducted in odd-numbered years. It is conducted by the US Census Bureau and funded by the U.S. Department of Housing and Urban Development (HUD). The survey covers residential housing units and uses computer-assisted personal interviews. It provides data on vacancies, housing inventory, the physical condition of housing units, occupant characteristics, neighborhood quality, home values, mortgages, and recent mover characteristics.
Advantages And Disadvantages
Some of the advantages and disadvantages of such sampling are as follows:
Advantages
- It saves time and money by providing faster results due to its small sample size compared to the whole population.
- Trained and experienced investigators perform sampling so the results are reliable.
- Sampling allows the estimation of sampling errors, providing information about population characteristics.
- Sample analysis requires less space and equipment due to their smaller size and works with limited resources.
Disadvantages
- Sampling carries the risk of bias, especially when certain groups are underrepresented or excluded.
- If the sampling process is inaccurate, the sample's findings may not represent the entire population.
- The collection process may be tedious, and the calculation may be tiring. The method used may also contribute to the complexity.
- In addition to this, choosing the sampling method and size may require statistical knowledge.
Frequently Asked Questions (FAQs)
In the CRISP-DM ((Cross-Industry Standard Process for Data Mining))methodology, data sampling happens when we're getting the data ready for analysis or the "Data preparation" stage. We choose the dataset we want to work with and make changes to it so that we can analyze it better.
In machine learning, data sampling means picking a smaller group of data from a larger dataset to train and test machine learning models. It helps work faster, reduces biases, and makes the analysis more efficient.
Some of the principles of sampling include a random selection of the data; the bigger the sample size, the lesser the error, sampling results will be true on an average over long periods etc.
The data sampling rate is about how much data or proportion is chosen from the whole dataset. It decides how big the sample is compared to the entire population. This affects how well the sample represents the whole group and how accurate the results are.
Recommended Articles
This article has been a guide to what is Data Sampling. Here, we explain its types, examples, process steps, advantages, and disadvantages. You may also find some useful articles here -