Data Quality Assurance
Last Updated :
21 Aug, 2024
Blog Author :
Edited by :
Ashish Kumar Srivastav
Reviewed by :
Dheeraj Vaidya
Table Of Contents
What Is Data Quality Assurance?
Data quality assurance (DQA) refers to the process that involves screening and determining anomalies through data cleaning, eliminating obsolete information, and data profiling. Organizations must perform this process to ensure they have up-to-date and clean data. Businesses must carry out such a procedure to achieve and sustain high-quality data.
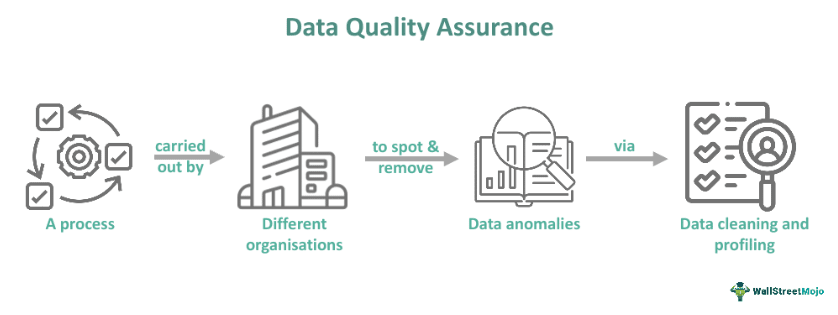
Data are always at risk of getting distorted because of external factors and the influence of individuals. Implementing an organization-wide DQA strategy can help organizations safeguard the value of their data. This type of strategy includes technical interventions and corporate governance measures. Moreover, allows managers to take more prudent decisions owing to improved ML or machine learning models.
Table of contents
- Data quality assurance refers to a procedure that involves utilizing data profiling to find out anomalies and inconsistencies in the data and performing conducting cleansing activities, like eliminating outliers, to enhance the quality of data.
- There are various steps involved in the DQA process. Some of them are data profiling, standardizing, matching and linking, and monitoring.
- The best practices of DQA include corporate measures, compliance, data accuracy and consistency, timeliness, and more.
- A noteworthy benefit of data quality assurance is that it allows companies to discover opportunities in the market quickly.
Data Quality Assurance Explained
Data quality assurance refers to a collective term for processes utilized to ensure that the integrity of the data housed within different databases remains intact. This process is essential for businesses to achieve high data quality and sustain the same.
Often maintaining data quality involves different tasks, such as cross-referencing relevant data stored in different databases, removing obsolete information, and ensuring that no inconstancy exists in the information available within a single or a group of databases. This form of data cleansing is a key aspect of a well-organized data administration.
Different businesses execute the DQA procedure. Depending on an organization’s operating structure, the process may involve ensuring that individual databases, for example, sales database and account receivables, store updated and accurate data. At other times, this procedure ensures that the data qualifies before its warehousing takes place in a backup format. This ensures the warehoused data are accurate and complete during storage.
In many cases, the actual DQA process focuses on spotting and rectifying discrepancies that could exist in the data an organization maintains. This kind of data profiling helps ensure that the data in a particular database is in accordance with the data stored in another database.
For instance, proper data management dictates that the pricing offered to a specific customer must be identical in the sales and accounts receivable databases. This minimizes the possibility of customers getting incorrect information about the current structure of pricing when interacting with the accounting or sales department.
Sometimes, the DQA process converts data into a common format to warehouse or archive the information. Reconciliation of the data before warehousing offers an accurate and complete history for past calendar years that one can access if and when necessary.
Process Steps
The data quality assurance procedure involves the following steps:
#1 - Define Usefulness Metrics
Whether it is to help managers make more prudent business decisions faster or assist the ground-level staff in becoming more responsive, the data must be useful. Hence, one must define the features of ‘useful data.’
- Precision
- Accuracy
- Relevancy
- Validity
- Trustworthiness
- Timeliness
- Completeness
- Ability to be comprehended
Thus, one has several options to define ‘useful data.’
#2 - Data Profiling
Profiling means analyzing information to clarify relationships, content, derivation rules, and structure of the data. When performing this step, users often have an intuitive-level understanding of how the information is unrelated. That said, machines still require precise instructions. Hence, one must profile the data to ensure it works for the users through data analysis software.
One must start by clarifying the relationships of various data points with each other. Moreover, individuals must decide how to group and structure the data for profiling.
#3 - Data Standardization
This step involves formulating policies concerning data standardization to ensure high data quality. The standards help in improving communications. One must note that standardization is of two types — internal and external. The external standards are suitable for the commonly utilized data types. For instance, individuals can use ISO-8601, a globally accepted standard, to represent daytime. Also, one must note that consistent internal standards can help employees remain on the same page and operate within the paradigm established.
#4 - Linking Or Matching
This involves comparing data to align nearly identical records. Matching may utilize ‘fuzzy logic’ to spot duplicates within the data. Usually, it recognizes that ‘Sam’ and ‘Sma’ could be the same person. It may be able to handle ‘householding’ or identify links between the spouses residing at the same address. Finally, it can take the best components from different data sources and create one super record.
#5 - Monitoring
Individuals must constantly track the alterations in the data received and the outputs produced. A new competitor or regulatory changes may bring alterations to the scene. Moreover, technological advancement may force individuals to make adjustments to their data analysis procedure.
Constantly monitoring data ensures that individuals do not pollute their data warehouse with non-compliant or incorrect data points. One can utilize software to reduce the workload concerning data tracking. The software sends notifications to departments responsible for sanitizing or accumulating the data whenever it detects anomalies, like wrongly inputted data.
#6 - Batch And Real-time
After the data are initially cleansed, organizations usually wish to build the procedures into enterprise applications to ensure the data remain clean.
Examples
Let us look at a few data quality assurance examples to understand the concept better:
Example #1
In May 2023, Validity, a leading provider of email deliverability and data quality solutions, announced that it would launch the Demand Tools File edition to enhance data quality across different departments of the organization.
Moreover, it will help in managing duplicates in spreadsheets within minutes. This means that the efficiency of people utilizing spreadsheets to monitor data will increase as they will know they are working with unique data. For instance, marketing teams can now deduplicate external lists in real-time before transferring data into the Marketing Automation Platform or CRM (Customer Relationship Management) system.
In addition, data analysts can carry out their data quality assurance procedure with the belief that they are running the analytics on one-of-a-kind datasets.
Example #2
Crowdworks, a leading data platform for AI or artificial intelligence, introduced its AI data solutions and HR or human resources management system that is specialized in digital platform and data annotation at the CSE 2023 conference program. On the basis of this move, the organization suggests a new focus on the human resources management system within a digital platform and the ways in which the system impacts the quality of data and projects’ productivity.
Based in Seoul, South Korea, this organization offers the first international data platform that provides comprehensive data quality assurance conducted by human reviewers. Managing 440,000 crowd workers with more than 170 profile indexes, the organization delivers data of high quality with maximum efficiency.
Best Practices
Some crucial DQA practices are as follows:
- Relevance: One must be able to interpret the data. In other words, the organization should have the right data processing techniques so that the company software can interpret the data format. Moreover, the legal conditions must allow the organization to utilize such data.
- Corporate Measure: An organization that monitors its data strategy can achieve and maintain high data quality by forming a department for data quality management. The department creates data quality rules compatible with organizational data governance to ensure data sustainability.
- Data Consistency And Accuracy: Organizations can ensure data consistency and accuracy using techniques like outlier detection and data filtering.
- Compliance: Organizations must ensure that their data fulfill the legal requirements.
- Timeliness: One must remember that the more updated the data, the more precise the calculations.
Importance
One must go through the following points to understand the importance of DQA:
- Execution of data throughout various departments within an organization for business insights becomes easier.
- Improved quality of data means quicker identification of business opportunities. Moreover, enhanced data quality gives organizations a stronger grip on the market.
- High data quality can increase profitability owing to a more prudent allocation of the resources available to an organization.
- Another key benefit of data quality assurance is that It allows organizations to make better business decisions owing to more accurate Machine Learning models.
Data Quality Assurance vs Data Quality Control
Data quality control and assurance may appear to be the same concepts to individuals new to data analysis. That said, one must note that they are not the same and have distinct features. Individuals must know such characteristics to learn how they differ.
Data Quality Assurance | Data Quality Control |
---|---|
This process deals with defect prevention. | It deals with defect detection. |
Organizations carry out this process prior to and while acquiring data. | Businesses or other organizations perform this process once they can access the data. |
This process ensures that an organization has updated and clean data. | It determines whether the information or data achieves the overall goals related to quality and fulfills the quality parameters for individual values. |
Frequently Asked Questions (FAQs)
Such tools are a number of techniques or methods utilized to assess monitoring and evaluation or M&E systems and plans that accumulate and report data or information for program reporting and management.
Almost all industries dealing with data can benefit from DQA. That said, a few examples of industries that can reap the benefits of this process are telecommunication, healthcare, agriculture, banking, and real estate.
Some common mistakes that organizations must try to minimize are as follows:
- Inaccurate data
- Duplicate data
- Hidden data
- Ambiguous data
- Data downtime
Data QA analysts implement and assess the system and document the overall status and tests for the data QA engineer. Usually, they are the main POC or point of contact with the particular line of business consuming the data. Moreover, the analysts play a vital role in the constant improvement process.
Recommended Articles
This article has been a guide to what is Data Quality Assurance. We explain its examples, process, importance, comparison with data quality control, & best practices. You may also find some useful articles here -