Table Of Contents
What Is A Data Lake?
A data lake is a data storage system that can store unstructured, semi-structured, and structured data from various sources. Additionally, it can store data on cloud-based applications and on-premises devices. It aims to provide a low-cost alternative to businesses that require to collect and store massive amounts of data.
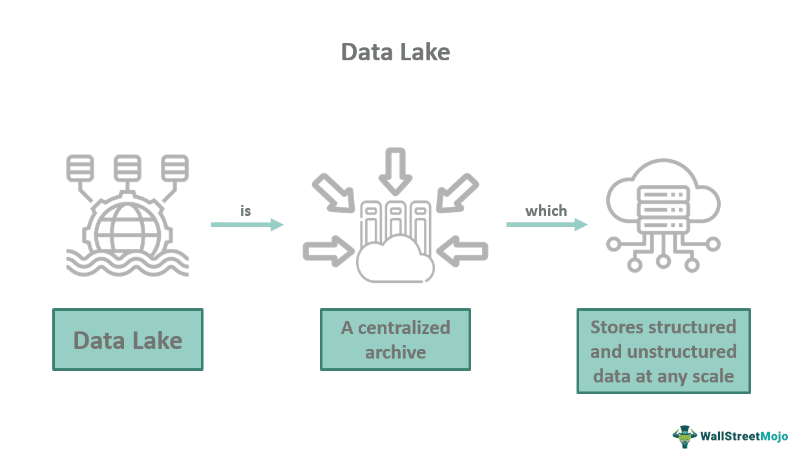
A data lake storage can hold data in its rawest form, like images, emails, and text files. It can also store structured information from relational databases. Businesses can store this data for analysis using machine learning and graph analytics. Most organizations are switching to cloud-based systems from on-premise systems over time.
Table of contents
- A data lake system stores massive amounts of unstructured, semi-structured, and structured data for further processing and analysis. It provides a cost-effective alternative to companies requiring significant data for their operations.
- These systems can be on-premises, requiring users to be located within the organization’s facilities. Or, it may be cloud-based, where the user can transfer and store data online in the supplier’s cloud.
- However, implanting this system is a complicated procedure that requires capital investment and the support of an IT expert.
Data Lake Explained
A data lake is a data archive that companies store vast amounts of data. This system allows users to import unstructured, semi-structured, and structured data. It is beneficial for organizations that require gathering and analyzing vast amounts of data as it is easy to use and cost-effective.
A data lake storage transfers information from numerous sources and stores it as raw, unstructured data. The information can be moved in an ongoing real-time stream or batches based on its source. Then the gathered data is listed so the users may know which data is stored in the system. Finally, a vast range of users can engage analytical instruments to gain insights from the data.
Types
The data lake technology types are as follows:
#1 - On-Premises Data Lakes
This system requires the user to install and run software to operate the system on the company data center’s storage and servers and storage. Organizations need significant capital investment to buy hardware and software licenses. It also requires an IT expert to install the system. An on-premises system may offer better performance to users located within the office premises.
#2 - Cloud Data Lakes
This system runs on the software and hardware in the supplier’s cloud, and the users can access them online. They usually come with a subscription model for payment. The supplier manages the data security, reliability, backup, and performance. The user must only decide which data to import into the system and how to process it.
Examples
Let us understand the concept with the following examples:
Example #1
Suppose Aurora Company is an organization that manufactures cosmetics products. It collects customer information from various sources, including email campaigns, advertising, and social media platforms. Aurora Company employed a system to import data from all those sources, including real-time feeds from phone applications and websites. This system will help the business identify its target customer base and rapidly changing preferences. The organization can analyze the data and develop customized marketing strategies that will help increase its conversion rate. This is a data lake example.
Example #2
Fairfield Market Research has stated that the data lake technology market is likely to reach a valuation of $18.6 billion by the end of 2026. It stated that this market is set for a 15.5% CAGR rate between 2021 and 2026. Due to rising demand from data analysts, data engineers, data scientists, and product managers, this technology will likely witness significant advancement soon. This is another data lake example.
Best Practices
The best practices for this technology are as follows:
- The organization must identify and define its goals. The users must know what the collected data will be used for. The user must set their goals for better utilization of the collected data.
- The business must employ modern data architecture to keep up with the rapid growth of technology and the need for data processing. The equipment must be well-maintained and updated periodically.
- The organizations must ensure that they set up a robust control system for maintaining data safety and privacy. The data must be clean, secure, easily accessible, and accurate. It is easier for the users to work on high-quality data that is clean and authentic.
Advantages And Disadvantages
The advantages of data lake technology are:
- Users can import unstructured, structured, or semi-structured data into a data lake. Collecting and analyzing data from different sources may add value to the research.
- This system may be helpful to a vast range of users across the business because it can store several types of information that can be analyzed in various ways. Data scientists can study the data using advanced analytical and modeling tools, while businesses can conduct more straightforward analyses.
- Information stored in this system is imported in its raw state without further processing.
- These platforms run on low-cost hardware, which makes them a relatively cost-effective data management tool. Thus, businesses can use them to store data that is snowballing.
The disadvantages of this technology are:
- This system can turn into a data swamp of useless and redundant information. Users must not be permitted to import any data they want, and companies must effectively list the data to ensure data accuracy and remove worthless details.
- This technology’s implementation is a complex process and requires careful planning.
Data Lake vs Data Warehouse vs Data Mesh
The differences are as follows:
- Data Lake: The data lake platform can hold both unstructured and structured data from many diversified sources, including weather information, social media posts, and factory equipment sensors. There is no prearranged architecture; data is transferred into the database in its most native and raw form without being processed first. Usually, businesses manage and list the data so that it is precise and the users get to know what data is obtainable. In this platform, new data can import quickly into the archive and be accessible for analysis. This system allows its users to navigate the raw data in several ways, which is beneficial for organizations that do not know beforehand what kinds of data they will require for their operations. This architecture can cost-effectively store massive amounts of data.
- Data Warehouse: This system is created to arrange unfiltered data in enormous amounts from various sources. In the data warehouse, the stored data is processed and structured. The sorted data can be processed for further analysis. This system allows multiple people to access the data simultaneously while delivering high performance.
- Data Mesh: This system has developed as a new approach for data to fulfill the large-scale, intricate, and rising needs in data management. In this platform, the methods and instruments are interconnected and decentralized. As a result, the entire structure is managed on a colossal scale.
Frequently Asked Questions (FAQs)
Setting up storage is the first step in building the data lake platform. Then the user must move the data to that storage. Next, the user must cleanse, prepare, and list the transferred data in the next step. Then they must design and implement security and compliance policies. Finally, the user must make the data available for further analysis.
This platform can store data in its raw and native format. These archives are capable of storing data in terabytes and petabytes. The data usually originates from several diversified sources. They may be unstructured, semi-structured, or structured. It can include structured data from relational databases, that is, columns and rows, or semi-structured data, which includes XML, logs, CSV, and JSON. The data may also be unstructured, like documents, PDFs, and emails, or binary data like audio, video, images, and audio.
This technology benefits businesses that must store large volumes of data in several formats and for real-time analysis. It also aids organizations that use raw, unstructured data for processing and output. It is a cost-effective alternative for data storage.
Recommended Articles
This article has been a guide to what is Data Lake. We compare it with data warehouse and data mesh, its examples, best practices, types, advantages & disadvantages. You may also find some useful articles here -